Sequential Attack Detection In Recommender Systems
IEEE TRANSACTIONS ON INFORMATION FORENSICS AND SECURITY(2021)
摘要
Recommender systems are widely used in electronic commerce, social media and online streaming services to provide personalized recommendations to the users by exploiting past ratings and interactions. This paper considers the security aspect with quick and accurate detection of attacks by observing the newly created profiles sequentially to prevent the damage which may be incurred by the injection of new profiles with dishonest ratings. The proposed framework consists of a latent variable model, which is trained by a variational EM algorithm, followed by a sequential detection algorithm. The latent variable model generates homogeneous representations of the users given their rating history and mixed data-type attributes such as age and gender. The representations are then exploited to generate univariate statistics to be efficiently used in a CUSUM-like sequential detection algorithm that can quickly detect persistent attacks while maintaining low false alarm rates. We apply our proposed framework to three different real-world datasets and exhibit superior performance in comparison to the existing baseline algorithms for both attack profile and sequential detection. Furthermore, we demonstrate robustness to different attack strategies and configurations.
更多查看译文
关键词
Detection algorithms, Recommender systems, Data models, Computational modeling, History, Hidden Markov models, Data integration, Recommender systems, cyber-attack detection, quickest detection, latent variable model, variational inference
AI 理解论文
溯源树
样例
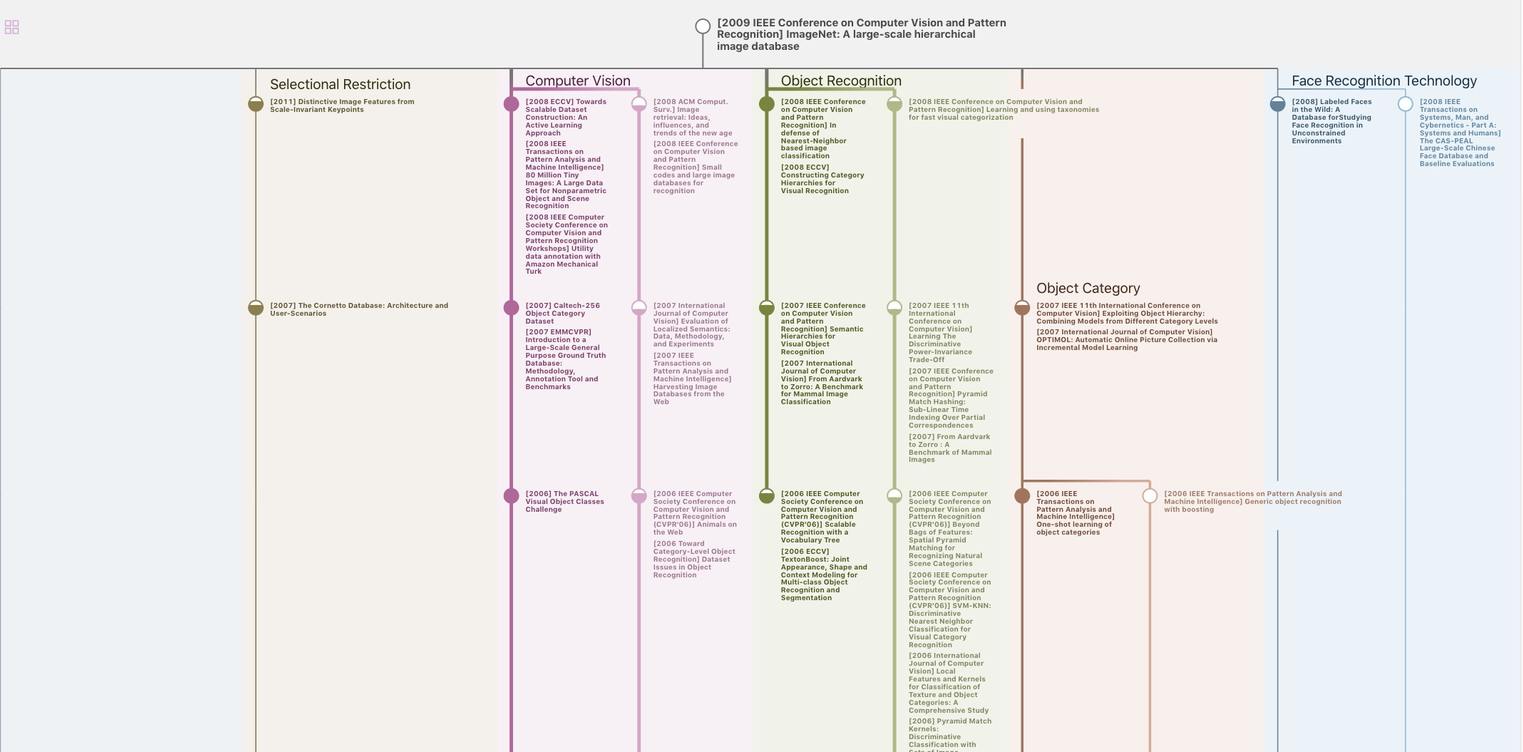
生成溯源树,研究论文发展脉络
Chat Paper
正在生成论文摘要