DTAE: Deep Tensor Autoencoder for 3-D Seismic Data Interpolation
IEEE TRANSACTIONS ON GEOSCIENCE AND REMOTE SENSING(2022)
摘要
The core challenge of seismic data interpolation is how to capture latent spatial-temporal relationships between unknown and known traces in 3-D space. The prevailing tensor-based interpolation schemes seek a globally low-rank approximation to mine the high-dimensional relationships hidden in 3-D seismic data. However, when the low-rank assumption is violated for data involving complex geological structures, the existing interpolation schemes fail to precisely capture the trace relationships, which may influence the interpolation results. As an alternative, this article presents a basic deep tensor autoencoder (DTAE) and two variants to implicitly learn a data-driven, nonlinear, and high-dimensional mapping to explore the complicated relationship among traces without the need for any underlying assumption. Then, tensor backpropagation (TBP), which can be essentially viewed as a tensor version of traditional backpropagation (BP), is introduced to solve for the new model parameters. For ease of implementation, a mathematical relationship between tensor and matrix autoencoders is constructed by taking advantage of the properties of a tensor-tensor product. Based on the derived relationship, the DTAE weight parameters are inferred by applying a matrix autoencoder to each frontal slice in the discrete cosine transform (DCT) domain, and this process is further summarized into a general theoretical and practical framework. Finally, the performance benefits of the proposed DTAE-based method are demonstrated in experiments with both synthetic and real field seismic data.
更多查看译文
关键词
Tensors, Interpolation, Data models, Solid modeling, Transforms, Training, Mathematical model, Deep tensor autoencoder (DTAE), low-rank constraint, matrix autoencoder, tensor back propagation, tensor-tensor product (t-product)
AI 理解论文
溯源树
样例
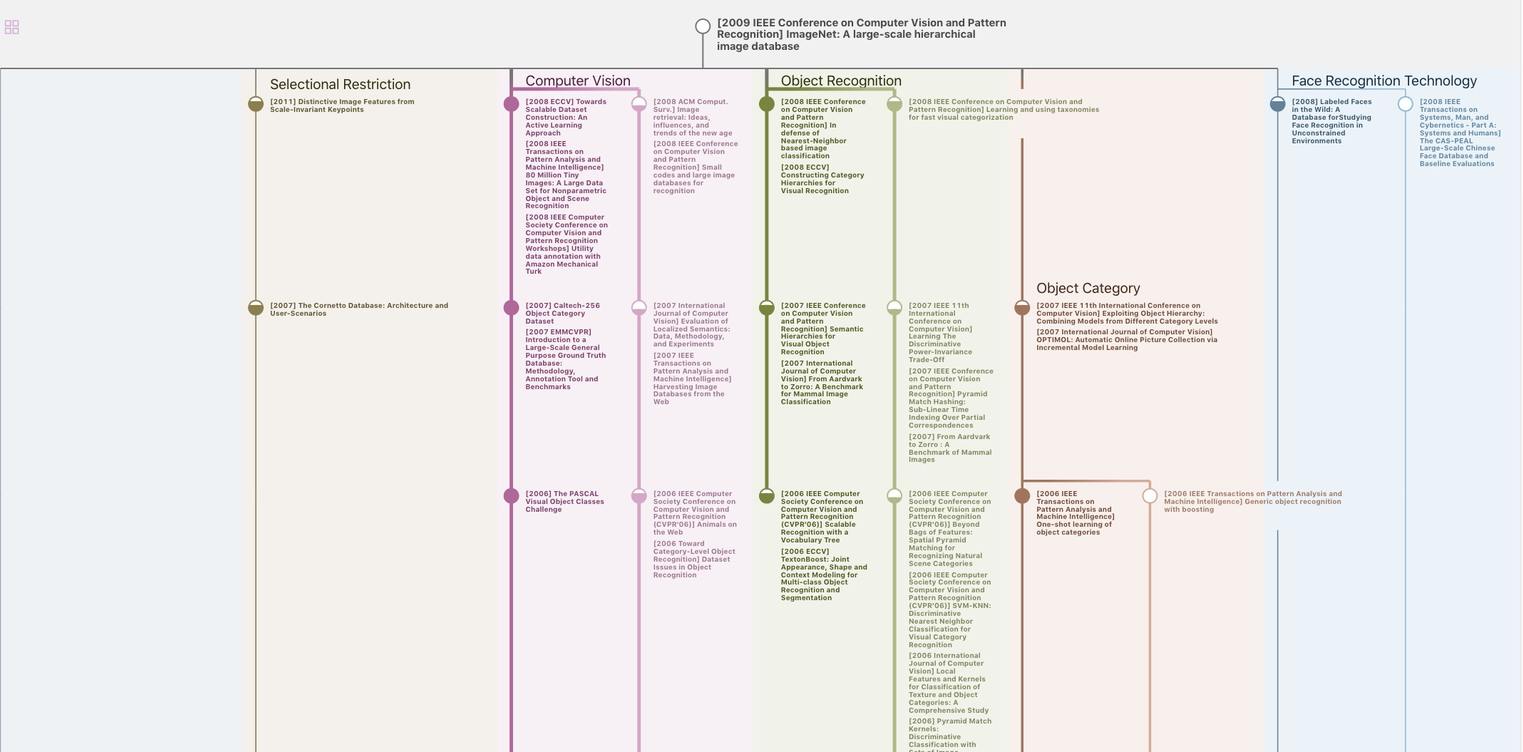
生成溯源树,研究论文发展脉络
Chat Paper
正在生成论文摘要