A Solver Of Fukunaga Koontz Transformation Without Matrix Decomposition
2021 IEEE INTERNATIONAL SYMPOSIUM ON CIRCUITS AND SYSTEMS (ISCAS)(2021)
摘要
Fukunaga Koontz Transformation provides a powerful tool for extracting discriminant subspaces in pattern classification. The discriminant subspaces are generally extracted by a matrix decomposition procedure involving scatter matrices where a nontrivial singularity problem is inevitable when sample number is limited. In this work, instead of matrix decomposition, a novel subspace extraction procedure based on solving a set of least-norm equations is proposed. This subspace extraction procedure does not rely on a large sample number and its computational complexity is only related to the number of samples. Experiments based on benchmark MNIST and PIE face recognition datasets show a promising potential of using the proposed method for certain image based recognition application where the image size is large while the sample number is limited.
更多查看译文
关键词
Fukunaga Koontz Transformation, subspace analysis, binary classification, face recognition
AI 理解论文
溯源树
样例
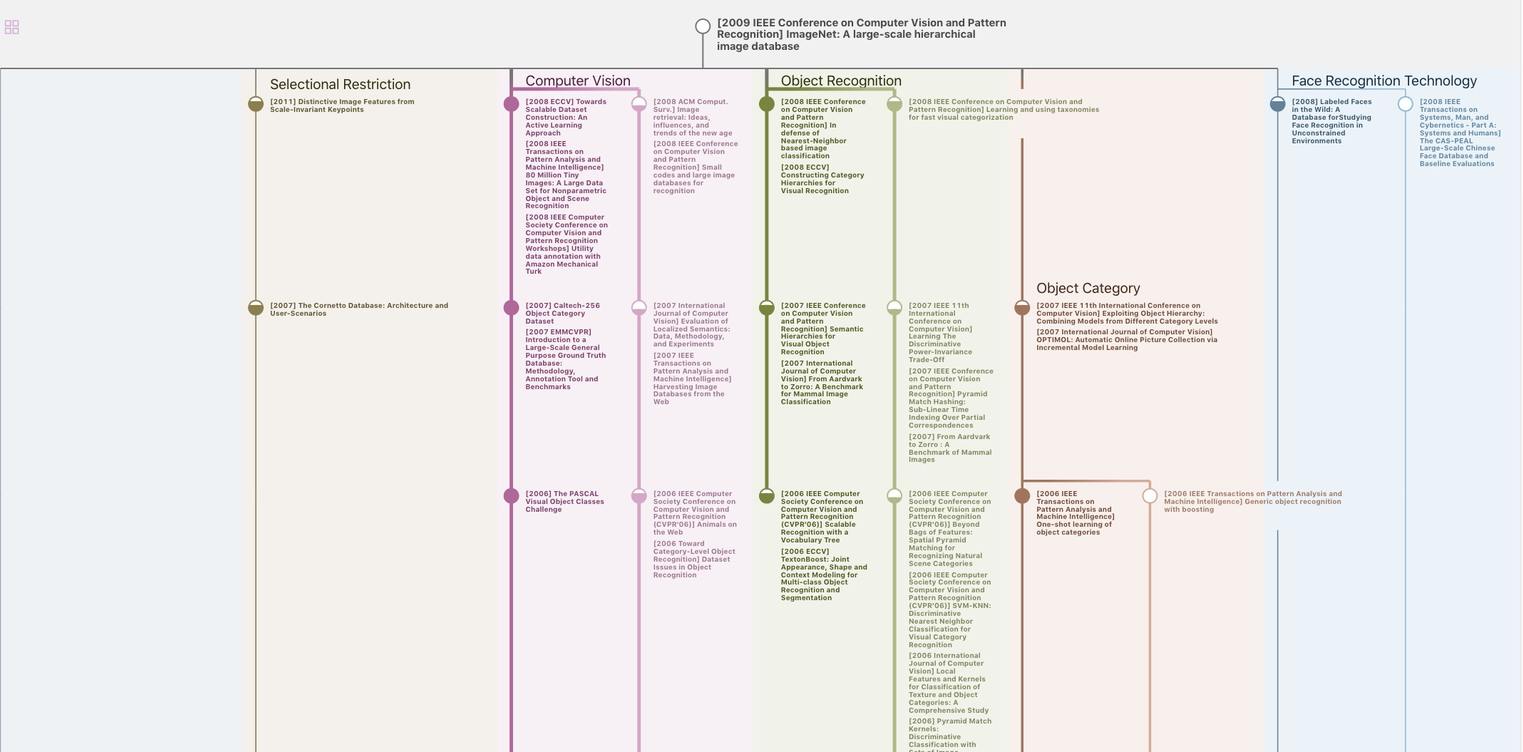
生成溯源树,研究论文发展脉络
Chat Paper
正在生成论文摘要