Improved Machine-Learning Mapping Of Local Climate Zones In Metropolitan Areas Using Composite Earth Observation Data In Google Earth Engine
BUILDING AND ENVIRONMENT(2021)
摘要
Accurate, large-scale local climate zone (LCZ) maps with data consistency are crucial for urban environmental studies globally. However, current approaches using Earth observation data and machine learning (ML) algorithms with local computation power are limited by low accuracy, coverage, and efficiency. Here, we present an improved workflow for generating consistent large-scale LCZ maps based on optimal data and ML algorithm selection using the Google Earth Engine (GEE) platform. Twelve data-composition scenarios and one optimized scenario were designed to explore the effects and synergetic use of nine Earth observation datasets, based on their reported potential in pixel-based classification. Our results show that depending on the intended use of the map, the random forest (RF) classifier and support vector machine (SVM) classifier are by far the most appropriate ML algorithms for pixel-based LCZ classification. While the RF classifier achieves a significantly higher overall accuracy and shows advantages in most of the individual classes, the SVM classifier exhibits significantly less variability with regard to accuracy. In addition, the competitive accuracy of the optimized scenario shows that using "elite variables" in the RF classifier can significantly improve classification accuracy while also reducing computational burden. Furthermore, thermal-infrared variables are far more influential than other variables in LCZ classification. Our study is the first attempt to make a cross-comparison of various remote sensing datasets and ML algorithms for LCZ classification using the GEE platform. As such, our results provide valuable new insights, workflows, and future directions for large-scale LCZ classification to support urban environmental studies globally.
更多查看译文
关键词
Machine learning, Earth observation, Land-use and land-cover, Local climate zone, Google earth engine, Greater bay area
AI 理解论文
溯源树
样例
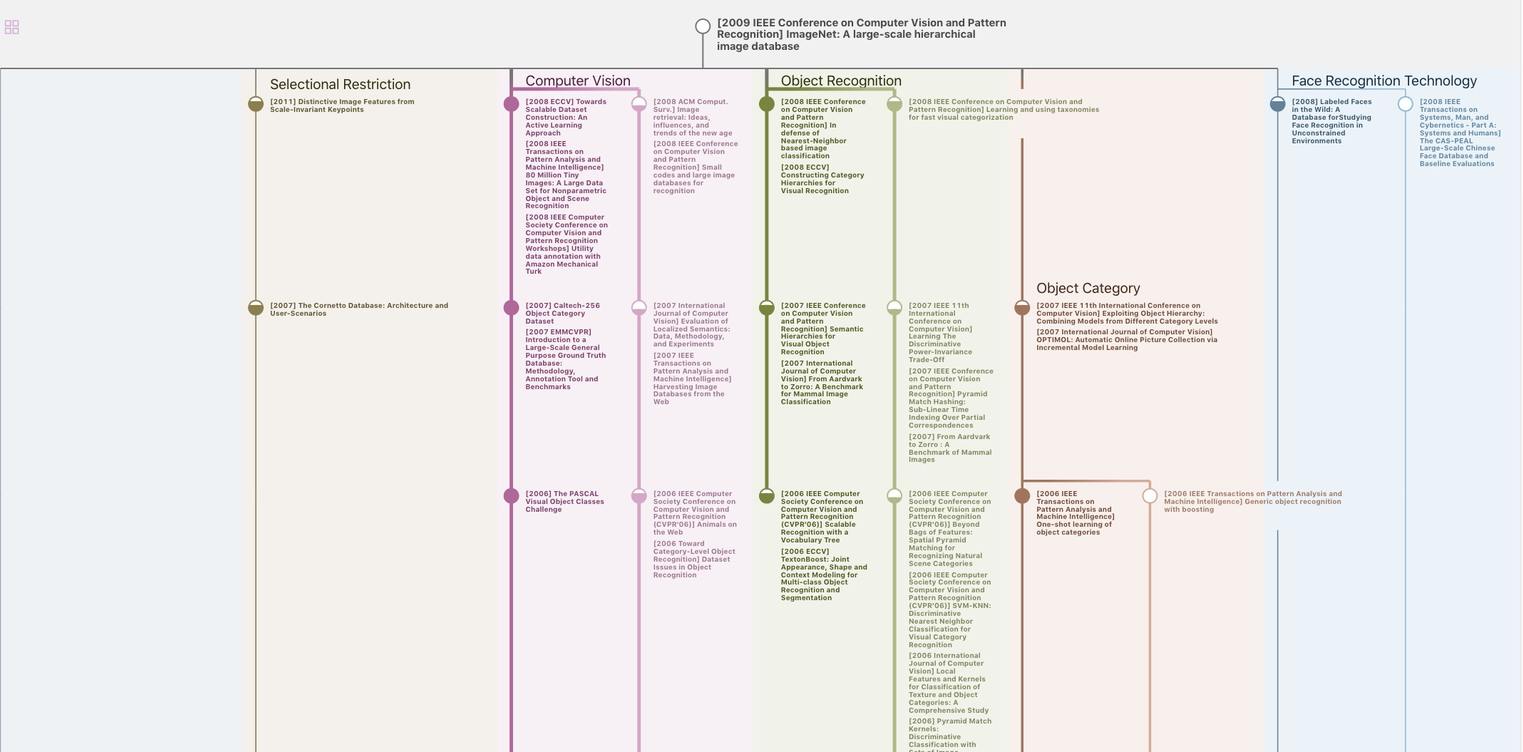
生成溯源树,研究论文发展脉络
Chat Paper
正在生成论文摘要