ASPset: An outdoor sports pose video dataset with 3D keypoint annotations
Image and Vision Computing(2021)
摘要
Recent advances in deep learning approaches to computer vision problems have led to renewed interest in the task of predicting 3D human joint locations from raw image data, with application areas including sports analysis, human-computer interaction, and physical rehabilitation. Although supervised learning of deep neural networks has proven to be effective for pose estimation, it requires a wealth of varied data to generalise well to previously unseen examples. Consequently, progress in 3D human pose estimation has been slowed by the fact that 3D keypoint annotations are notoriously difficult to obtain, traditionally requiring a large array of cameras and/or the use of wearable markers/sensors. In this paper we describe a methodology for obtaining 3D human pose annotations using only three video cameras and without any wearables. We apply this methodology to construct ASPset-510 (Australian Sports Pose Dataset), a large collection of natural sports-related video with 3D pose annotations. Using ASPset-510 as an additional source of training examples, we found that we could improve pose model generalisation on the established MPI-INF-3DHP benchmark. We make ASPset-510 publicly available, and provide strong baseline results for future work to compare against.
更多查看译文
关键词
Markerless motion capture,Human pose estimation,Triangulation,Camera calibration
AI 理解论文
溯源树
样例
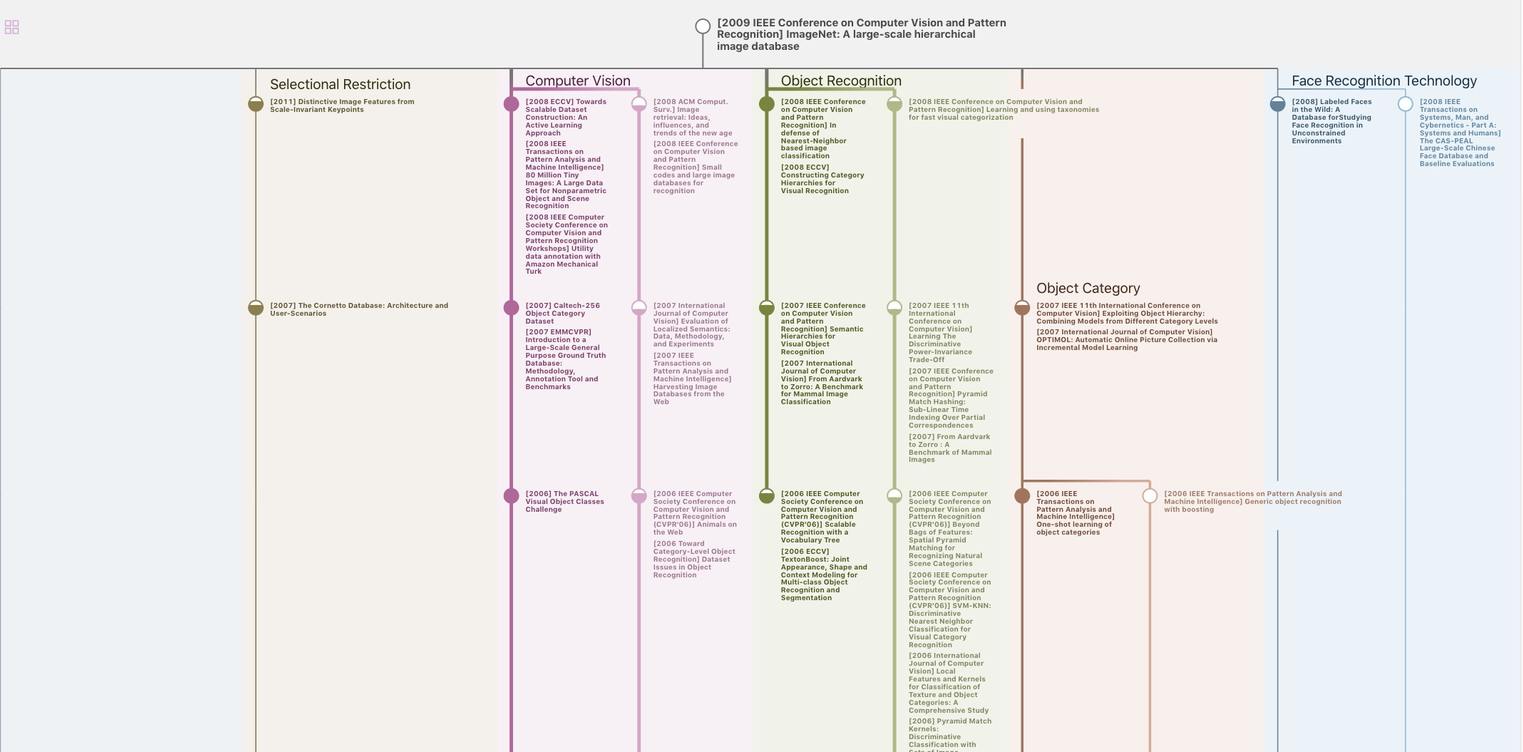
生成溯源树,研究论文发展脉络
Chat Paper
正在生成论文摘要