Generating Adversarial Patches Using Data-Driven MultiD-WGAN
2021 IEEE INTERNATIONAL SYMPOSIUM ON CIRCUITS AND SYSTEMS (ISCAS)(2021)
摘要
In recent years, machine learning algorithms and training data are faced many security threats, which affect the security of practical applications based on machine learning. At present, generating adversarial patches based on Generative Adversarial Nets (GANs) has been an emerging study. However, existing attack strategies are still far from producing local adversarial patches with strong attack power, ignoring the attacked network's perceived sensitivity to the adversarial patches. This paper studies the security threat of adversarial patches to classifiers; adding an adversarial patch to the data can mislead the classifier into incorrect results. Considering the attention to aggression and reality, we propose the data-driven MultiD-WGAN, which can simultaneously enhance adversarial patches' attack power and authenticity through multi-discriminators. The experiments confirm that our data-driven MultiD-WGAN dramatically reduces the recall of seven classifiers attacked on four datasets. The attack of data-driven MultiD-WGAN on 25/28 groups of experiments leads to a decreased recall rate, which is better than the conventional GANs. Finally, we have proved a positive correlation between attack intensity and attack ability, both theoretically and experimentally.
更多查看译文
关键词
Generative Adversarial Nets, Attack Power, Adversarial Patches
AI 理解论文
溯源树
样例
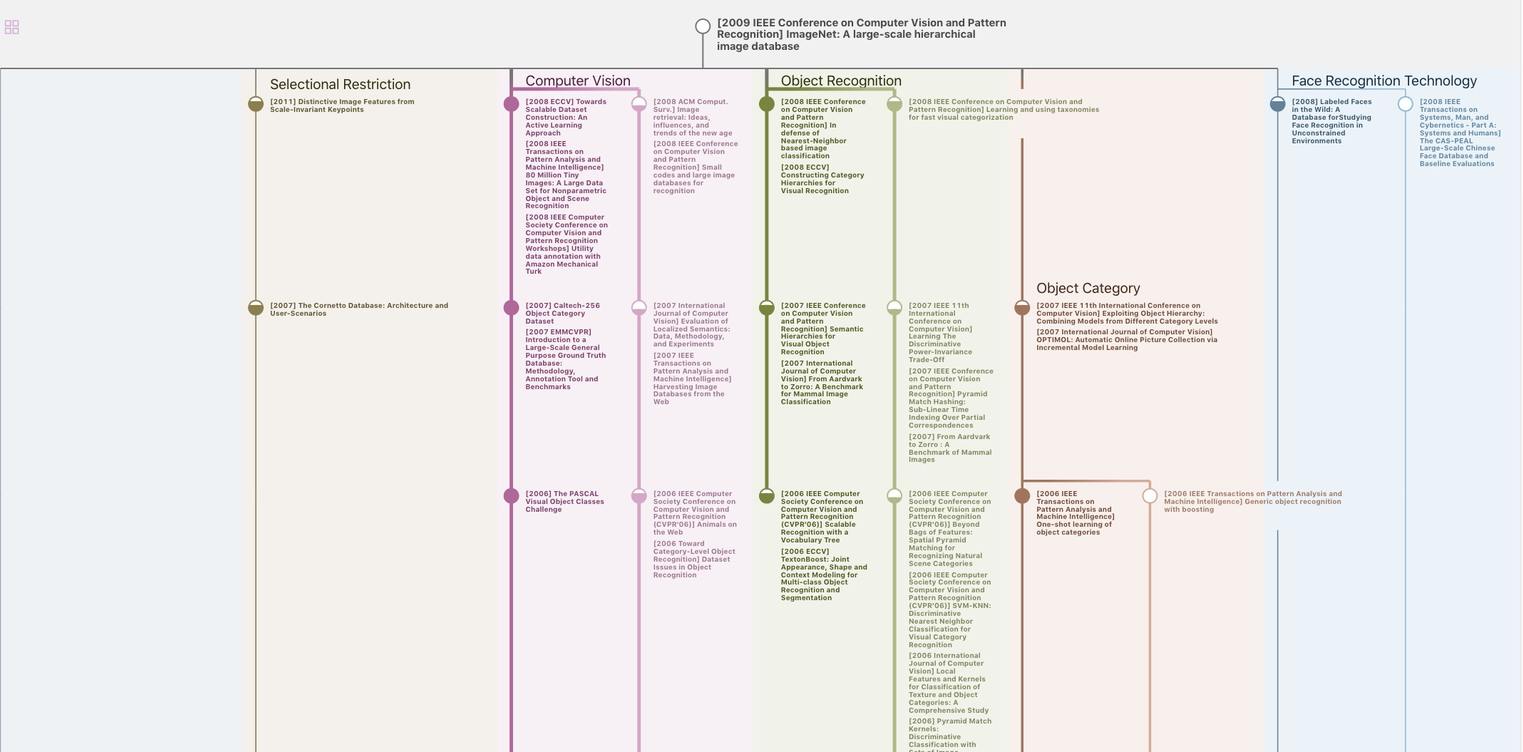
生成溯源树,研究论文发展脉络
Chat Paper
正在生成论文摘要