Continuous Estimation of Human Upper Limb Joint Angles by Using PSO-LSTM Model
IEEE access(2021)
摘要
Aiming at the continuous motion control problem of the upper limb auxiliary exoskeleton. In this paper, we use particle swarm optimization (PSO) to optimize the long short-term memory network (LSTM), and use the optimized network to establish a map between surface electromyography (sEMG) signals and joint angles. The inputs of the model are sEMG feature time series, which have been dimension reduction processed, and the outputs of the model are the joint angles of the elbow and wrist. To validate the effectiveness of the PSO-LSTM model, eight healthy subjects participated in the experiment. Eight channels of sEMG from eight human upper limb muscles were recorded and two joint angles including the elbow joint and wrist joint were acquired. The proposed PSO-LSTM model and back propagation neural network (BP) model were trained and tested, by using the sEMG feature time series. These time series have been processed for continuous estimation of human upper limb movements. Our experimental results showed that the proposed PSO-LSTM model could achieve a significantly lower estimation root mean square error (RMSE) than the BP model. The RMSE of PSO-LSTM is 0.0599 and the RMSE of BP is 0.1986 in the experiment of continuous elbow movement. The RMSE of PSO-LSTM is 0.1025 and the RMSE of BP is 0.2348 in the experiment of continuous wrist movement. These results suggest that the proposed PSO-LSTM model has a good performance on joint angles estimation by using sEMG feature time series that have been processed for subjects. This model would be used on rehabilitation robots for active rehabilitation of spinal cord injury (SCI) patients or stroke patients.
更多查看译文
关键词
Continuous estimation,long short-term memory network (LSTM),particle swarm optimization (PSO) algorithm,surface electromyography (sEMG)
AI 理解论文
溯源树
样例
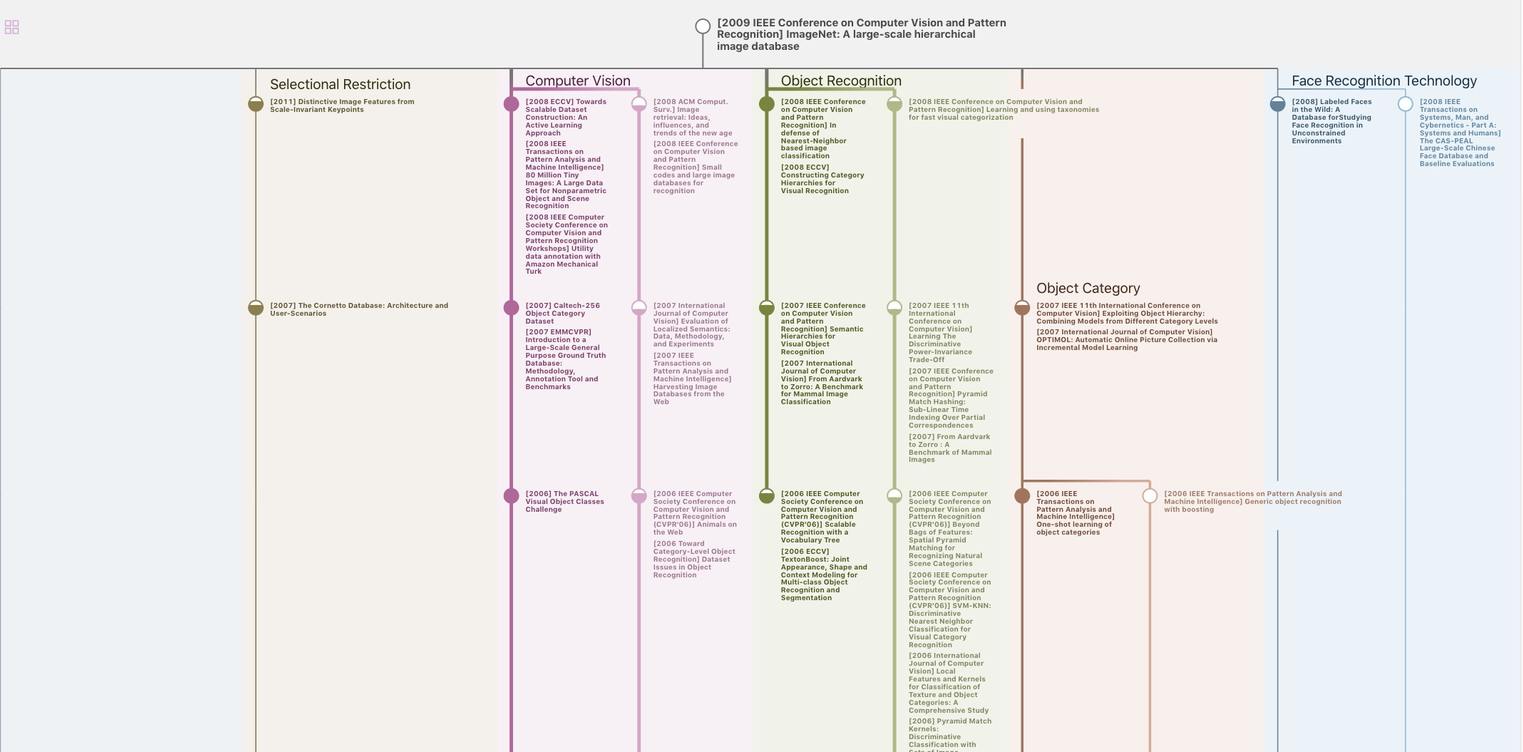
生成溯源树,研究论文发展脉络
Chat Paper
正在生成论文摘要