Evaluation Metrics for Graph Generative Models: Problems, Pitfalls, and Practical Solutions
International Conference on Learning Representations (ICLR)(2022)
摘要
Graph generative models are a highly active branch of machine learning. Given the steady development of new models of ever-increasing complexity, it is necessary to provide a principled way to evaluate and compare them. In this paper, we enumerate the desirable criteria for comparison metrics, discuss the development of such metrics, and provide a comparison of their respective expressive power. We perform a systematic evaluation of the main metrics in use today, highlighting some of the challenges and pitfalls researchers inadvertently can run into. We then describe a collection of suitable metrics, give recommendations as to their practical suitability, and analyse their behaviour on synthetically generated perturbed graphs as well as on recently proposed graph generative models.
更多查看译文
关键词
graph generative models,model evaluation
AI 理解论文
溯源树
样例
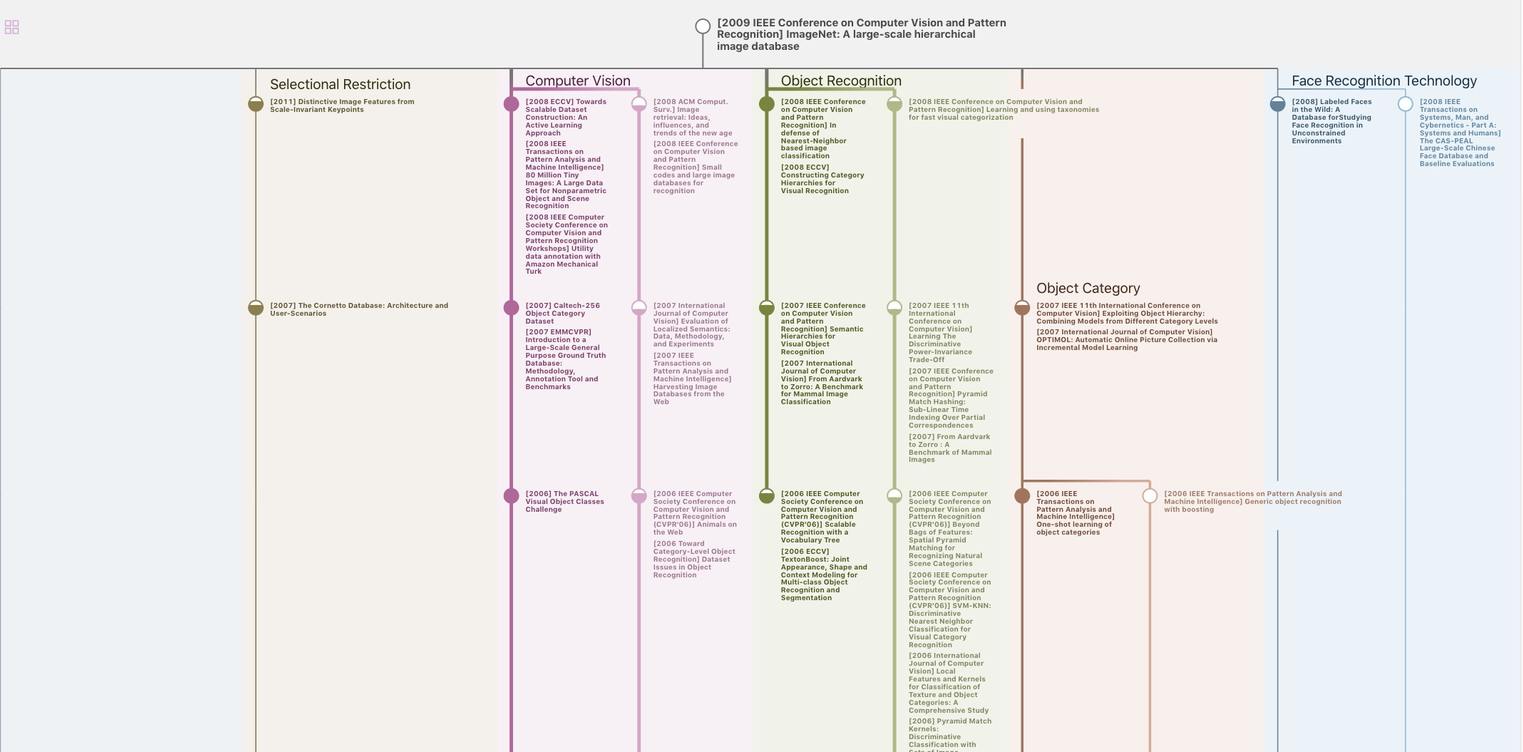
生成溯源树,研究论文发展脉络
Chat Paper
正在生成论文摘要