What Happened Next? Using Deep Learning to Value Defensive Actions in Football Event-Data
Knowledge Discovery and Data Mining(2021)
摘要
ABSTRACTObjectively quantifying the value of player actions in football (soccer) is a challenging problem. To date, studies in football analytics have mainly focused on the attacking side of the game, while there has been less work on event-driven metrics for valuing defensive actions (e.g., tackles and interceptions). Therefore in this paper, we use deep learning techniques to define a novel metric that values such defensive actions by studying the threat of passages of play that preceded them. By doing so, we are able to value defensive actions based on what they prevented from happening in the game. Our Defensive Action Expected Threat (DAxT) model has been validated using real-world event-data from the 2017/2018 and 2018/2019 English Premier League seasons, and we combine our model outputs with additional features to derive an overall rating of defensive ability for players. Overall, we find that our model is able to predict the impact of defensive actions allowing us to better value defenders using event-data.
更多查看译文
关键词
Sports Analytics, Football, Deep Learning, Neural Networks, Applied Machine Learning, Defensive Actions
AI 理解论文
溯源树
样例
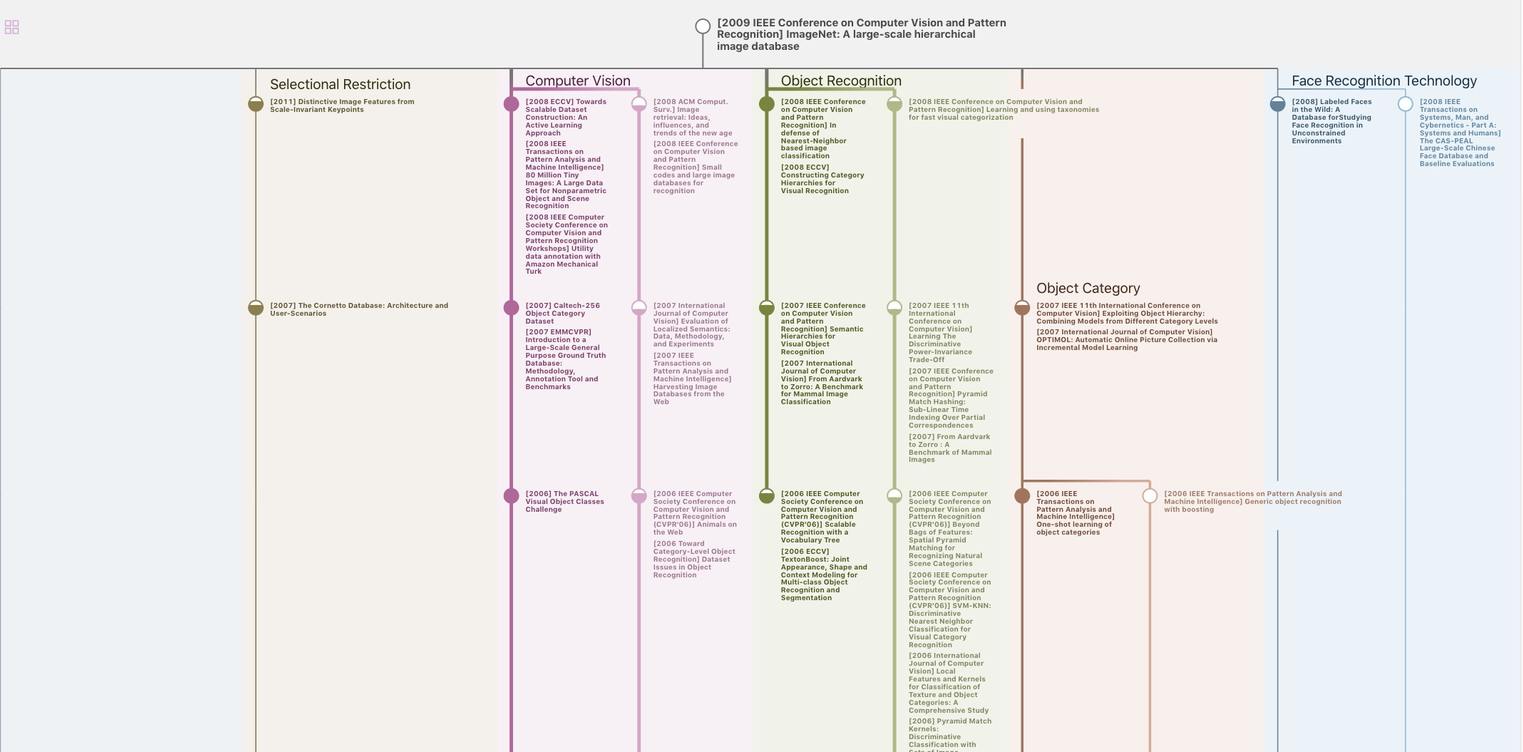
生成溯源树,研究论文发展脉络
Chat Paper
正在生成论文摘要