Learning from the Crowd with Pairwise Comparison
ICML 2021(2020)
摘要
Efficient learning of halfspaces is arguably one of the most important problems in machine learning and statistics. With the unprecedented growth of large-scale data sets, it has become ubiquitous to appeal to crowd for data annotation, and the central problem that attracts a surge of recent interests is how one can provably learn the underlying halfspace from the highly noisy crowd feedback. On the other hand, a large body of recent works have been dedicated to the problem of learning with not only labels, but also pairwise comparisons, since in many cases it is easier to compare than to label. In this paper we study the problem of learning halfspaces from the crowd under the realizable PAC learning setting, and we assume that the crowd workers can provide (noisy) labels or pairwise comparison tags upon request. We show that with a powerful boosting framework, together with our novel design of a filtering process, the overhead (to be defined) of the crowd acts as a constant, whereas the natural extension of standard approaches to crowd setting leads to an overhead growing with the size of the data sets.
更多查看译文
关键词
crowd,learning,comparison
AI 理解论文
溯源树
样例
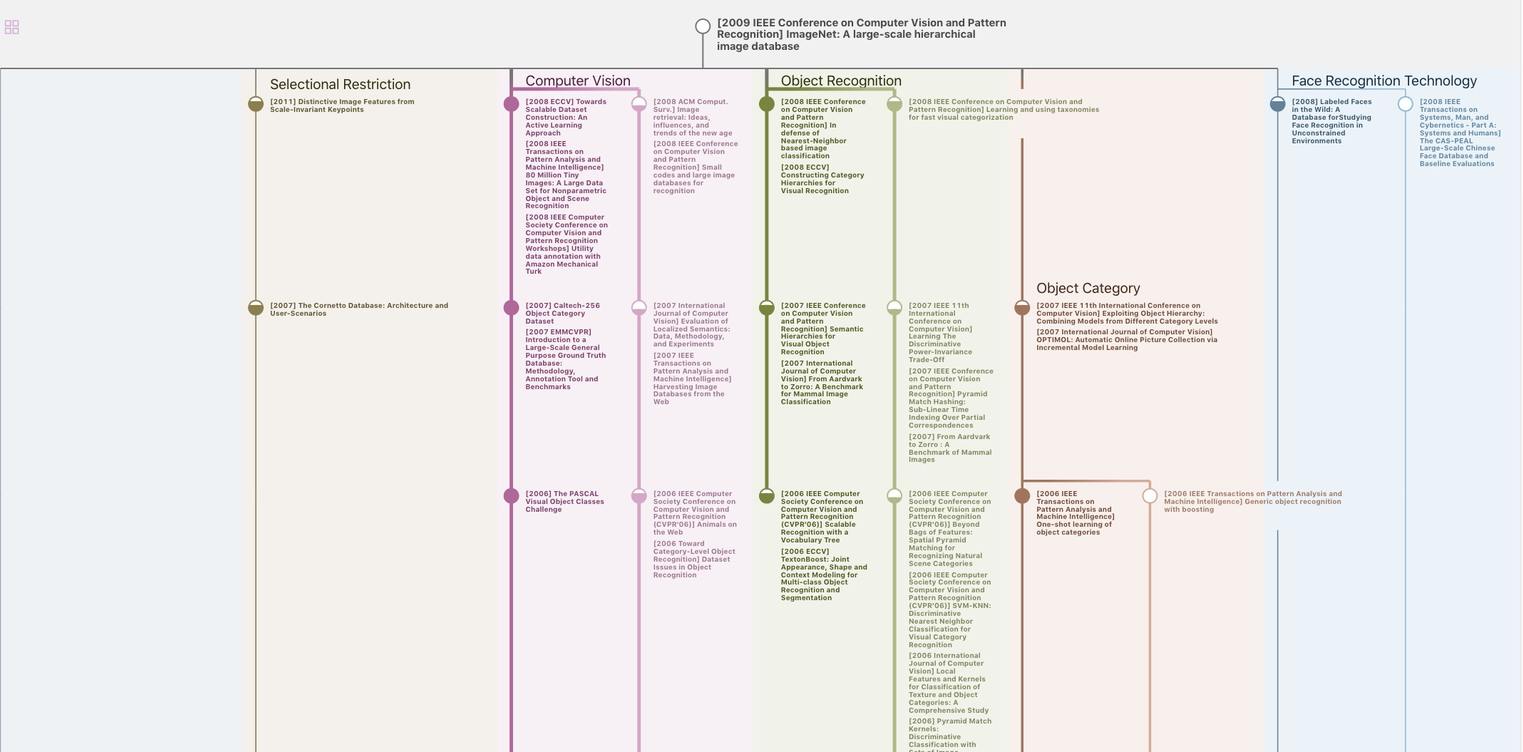
生成溯源树,研究论文发展脉络
Chat Paper
正在生成论文摘要