Adversarial Option-Aware Hierarchical Imitation Learning
INTERNATIONAL CONFERENCE ON MACHINE LEARNING, VOL 139(2021)
摘要
It has been a challenge to learning skills for an agent from long-horizon unannotated demonstrations. Existing approaches like Hierarchical Imitation Learning(HIL) are prone to compounding errors or suboptimal solutions. In this paper, we propose Option-GAIL, a novel method to learn skills at long horizon. The key idea of Option-GAIL is modeling the task hierarchy by options and train the policy via generative adversarial optimization. In particular, we propose an Expectation-Maximization(EM)-style algorithm: an E-step that samples the options of expert conditioned on the current learned policy, and an M-step that updates the low- and high-level policies of agent simultaneously to minimize the newly proposed option-occupancy measurement between the expert and the agent. We theoretically prove the convergence of the proposed algorithm. Experiments show that Option-GAIL outperforms other counterparts consistently across a variety of tasks.
更多查看译文
关键词
learning,option-aware
AI 理解论文
溯源树
样例
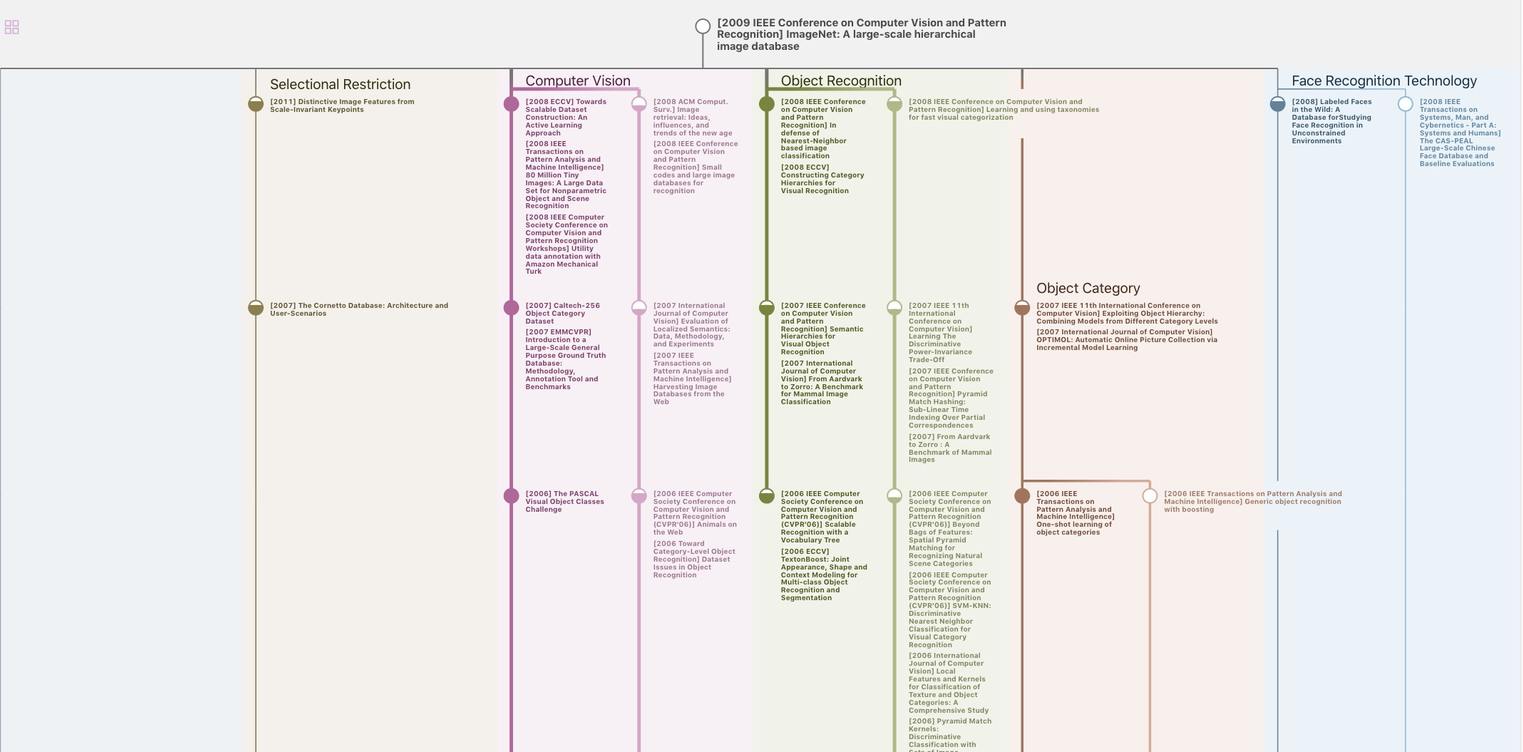
生成溯源树,研究论文发展脉络
Chat Paper
正在生成论文摘要