A Representation Learning Perspective on the Importance of Train-Validation Splitting in Meta-Learning
INTERNATIONAL CONFERENCE ON MACHINE LEARNING, VOL 139(2021)
摘要
An effective approach in meta-learning is to utilize multiple "train tasks" to learn a good initialization for model parameters that can help solve unseen "test tasks" with very few samples by fine-tuning from this initialization. Although successful in practice, theoretical understanding of such methods is limited. This work studies an important aspect of these methods: splitting the data from each task into train (support) and validation (query) sets during meta-training. Inspired by recent work (Raghu et al., 2020), we view such meta-learning methods through the lens of representation learning and argue that the train-validation split encourages the learned representation to be low-rank without compromising on expressivity, as opposed to the non-splitting variant that encourages high-rank representations. Since sample efficiency benefits from low-rankness, the splitting strategy will require very few samples to solve unseen test tasks. We present theoretical results that formalize this idea for linear representation learning on a subspace meta-learning instance, and experimentally verify this practical benefit of splitting in simulations and on standard meta-learning benchmarks.
更多查看译文
关键词
representation learning perspective,train-validation,meta-learning
AI 理解论文
溯源树
样例
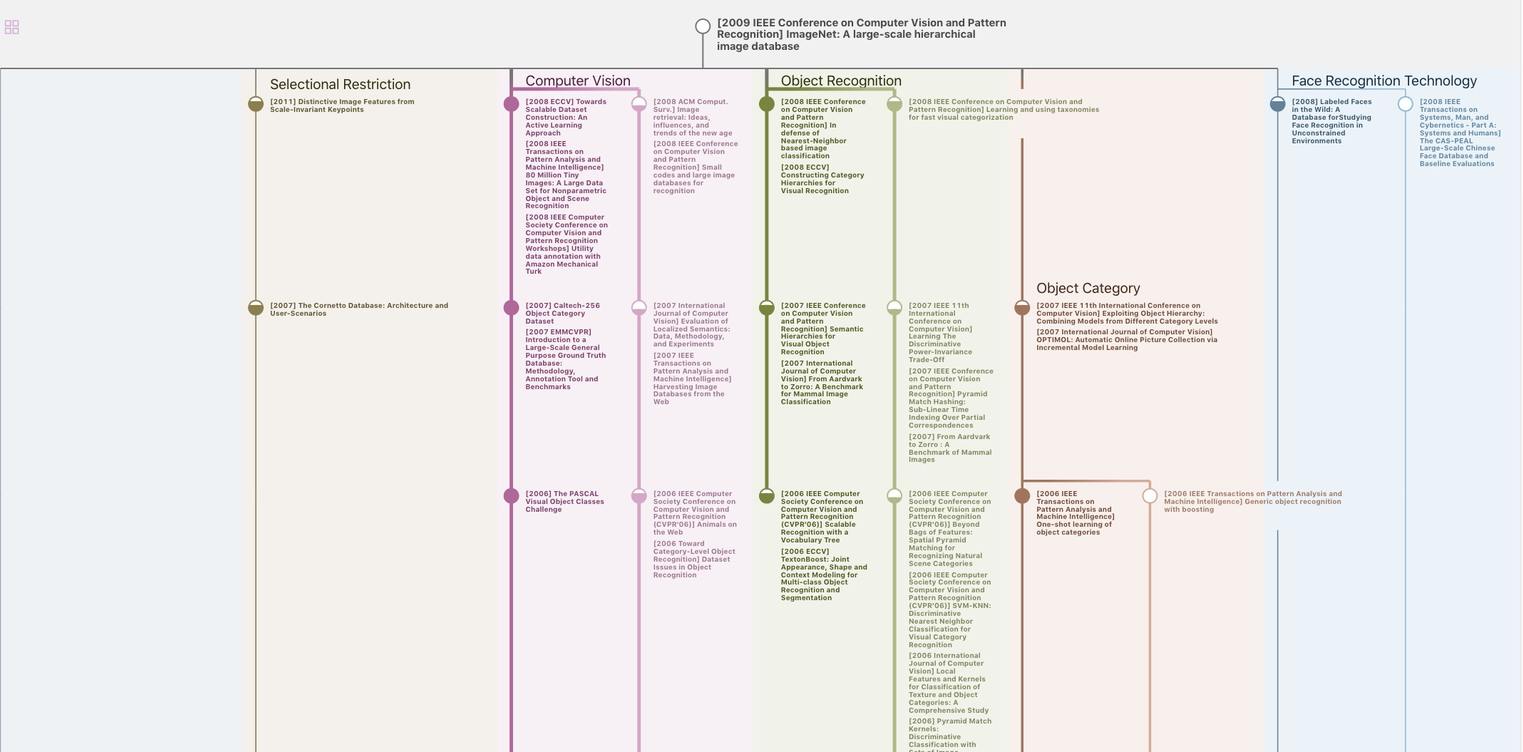
生成溯源树,研究论文发展脉络
Chat Paper
正在生成论文摘要