Almost Optimal Anytime Algorithm For Batched Multi-Armed Bandits
INTERNATIONAL CONFERENCE ON MACHINE LEARNING, VOL 139(2021)
摘要
In batched multi-armed bandit problems, the learner can adaptively pull arms and adjust strategy in batches. In many real applications, not only the regret but also the batch complexity need to be optimized Existing batched bandit algorithms usually assume that the time horizon T is known in advance. However, many applications involve an unpredictable stopping time. In this paper, we study the anytime batched multiarmed bandit problem. We propose an anytime algorithm that achieves the asymptotically optimal regret for exponential families of reward distributions with O(log log T . ilog(alpha)(T))(1) batches, where alpha is an element of O-T(1). Moreover, we prove that for any constant c > 0, no algorithm can achieve the asymptotically optimal regret within c log log T batches.
更多查看译文
关键词
optimal anytime algorithm,multi-armed
AI 理解论文
溯源树
样例
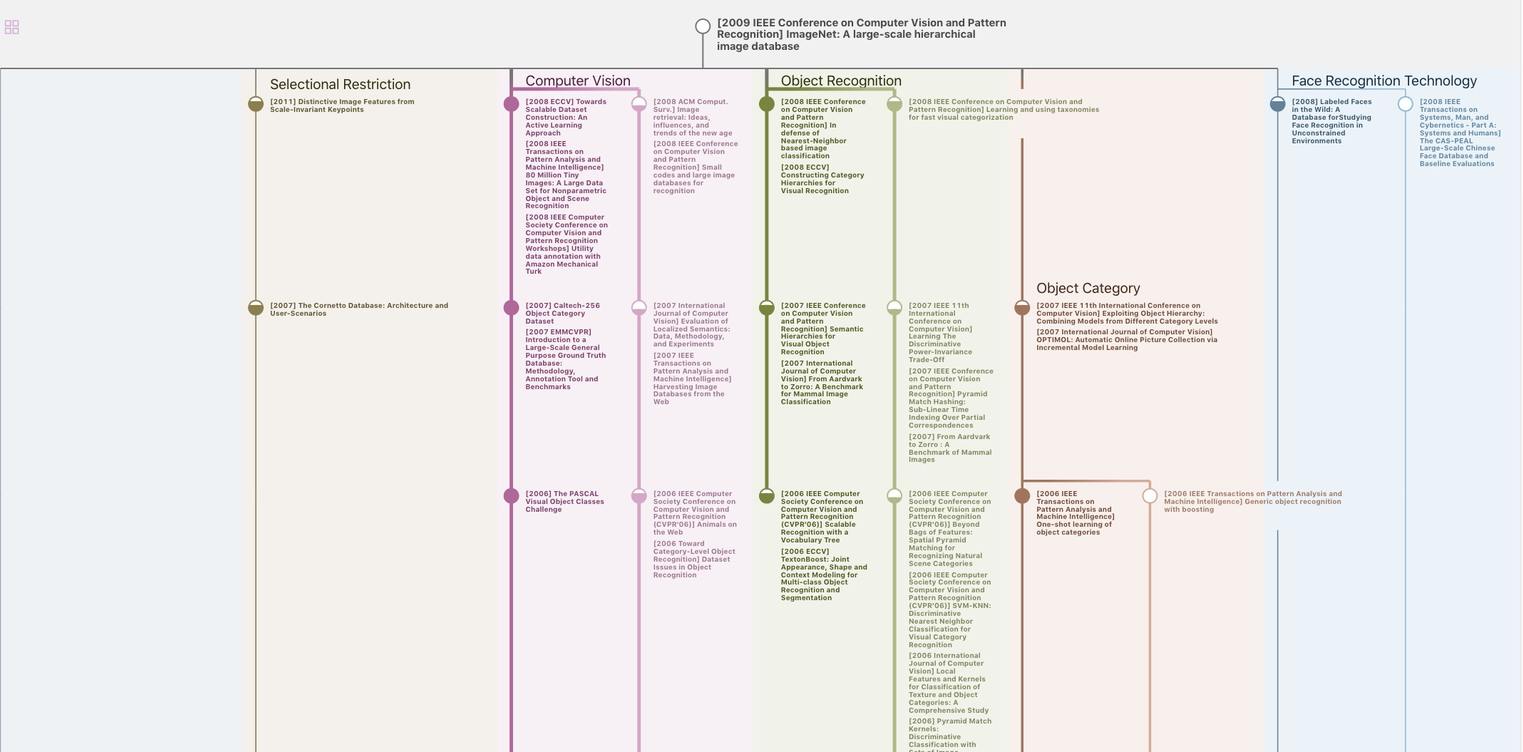
生成溯源树,研究论文发展脉络
Chat Paper
正在生成论文摘要