nmT5 - Is parallel data still relevant for pre-training massively multilingual language models?
arxiv(2021)
摘要
Recently, mT5 - a massively multilingual version of T5 - leveraged a unified text-to-text format to attain state-of-the-art results on a wide variety of multilingual NLP tasks. In this paper, we investigate the impact of incorporating parallel data into mT5 pre-training. We find that multi-tasking language modeling with objectives such as machine translation during pretraining is a straightforward way to improve performance on downstream multilingual and cross-lingual tasks. However, the gains start to diminish as the model capacity increases, suggesting that parallel data might not be as essential for larger models. At the same time, even at larger model sizes, we find that pre-training with parallel data still provides benefits in the limited labelled data regime.
更多查看译文
关键词
multilingual language models,parallel data,pre-training
AI 理解论文
溯源树
样例
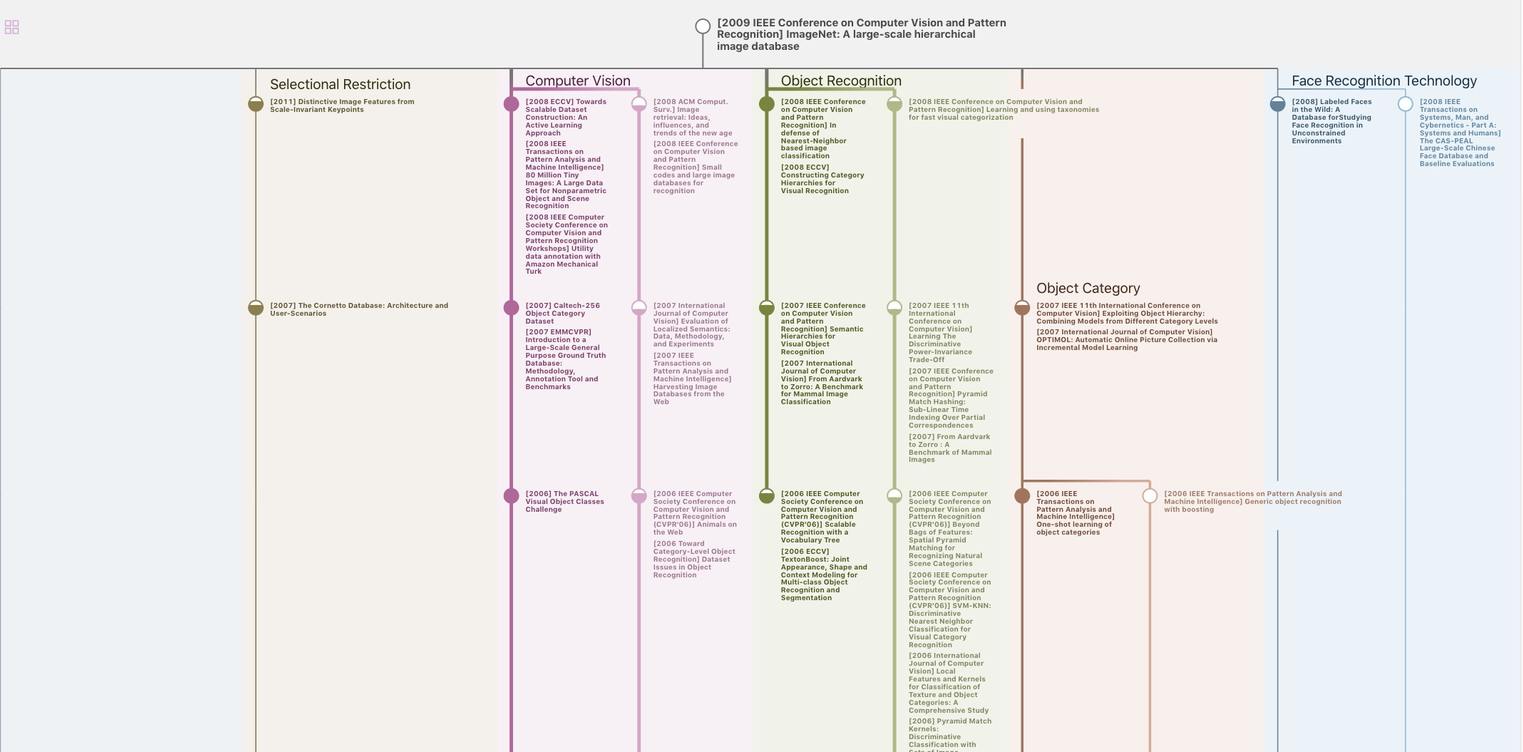
生成溯源树,研究论文发展脉络
Chat Paper
正在生成论文摘要