Distributed Nonparametric Regression Imputation for Missing Response Problems with Large-scale Data
JOURNAL OF MACHINE LEARNING RESEARCH(2023)
摘要
Nonparametric regression imputation is commonly used in missing data analysis. However, it suffers from the "curse of dimension". The problem can be alleviated by the explosive sample size in the era of big data, while the large-scale data size presents some challenges in the storage of data and the calculation of estimators. These challenges make the classical nonparametric regression imputation methods no longer applicable. This motivates us to develop two distributed nonparametric regression imputation methods. One is based on kernel smoothing and the other on the sieve method. The kernel-based distributed imputation method has extremely low communication cost, and the sieve-based distributed imputation method can accommodate more local machines. The response mean estimation is considered to illustrate the proposed imputation methods. Two distributed nonparametric regression imputation estimators are proposed for the response mean, which are proved to be asymptotically normal with asymptotic variances achieving the semiparametric efficiency bound. The proposed methods are evaluated through simulation studies and illustrated in a real data analysis.
更多查看译文
关键词
Distributed data,Divide and conquer,Kernel method,Missing data,Sieve method
AI 理解论文
溯源树
样例
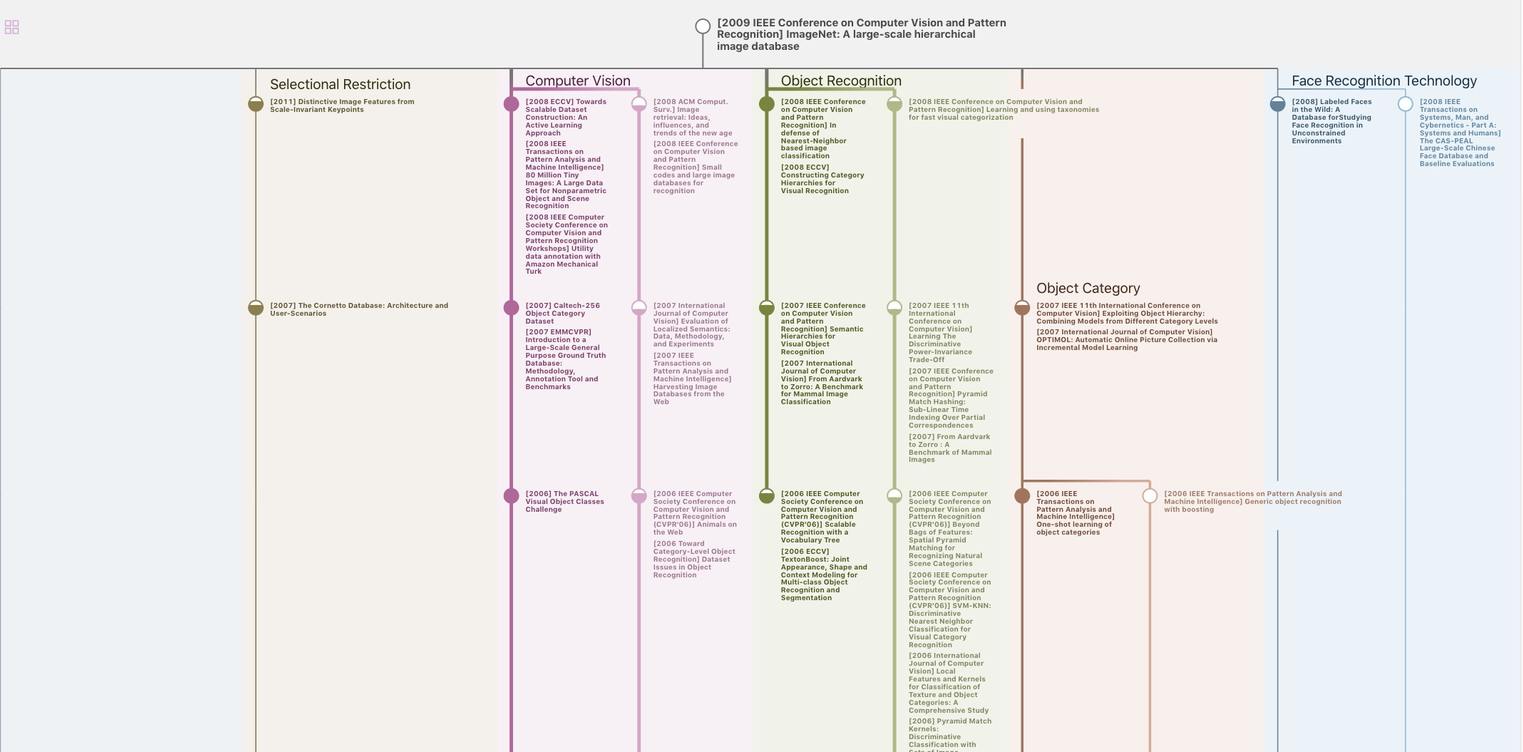
生成溯源树,研究论文发展脉络
Chat Paper
正在生成论文摘要