Optimal Rates of (Locally) Differentially Private Heavy-tailed Multi-Armed Bandits
INTERNATIONAL CONFERENCE ON ARTIFICIAL INTELLIGENCE AND STATISTICS, VOL 151(2022)
摘要
In this paper we investigate the problem of stochastic multi-armed bandits (MAB) in the (local) differential privacy (DP/LDP) model. Unlike previous results that assume bounded/sub-Gaussian reward distributions, we focus on the setting where each arm's reward distribution only has (1+v)-th moment with some v is an element of (0, 1]. In the first part, we study the problem in the central epsilon-DP model. We first provide a near-optimal result by developing a private and robust Upper Confidence Bound (UCB) algorithm. Then, we improve the result via a private and robust version of the Successive Elimination (SE) algorithm. Finally, we establish the lower bound to show that the instance-dependent regret of our improved algorithm is optimal. In the second part, we study the problem in the epsilon-LDP model. We propose an algorithm that can be seen as locally private and robust version of SE algorithm, which provably achieves (near) optimal rates for both instance-dependent and instance-independent regret. Our results reveal differences between the problem of private MAB with bounded/sub-Gaussian rewards and heavy-tailed rewards. To achieve these (near) optimal rates, we develop several new hard instances and private robust estimators as byproducts, which might be used to other related problems. Finally, experiments also support our theoretical findings and show the effectiveness of our algorithms.
更多查看译文
关键词
optimal rates,heavy-tailed,multi-armed
AI 理解论文
溯源树
样例
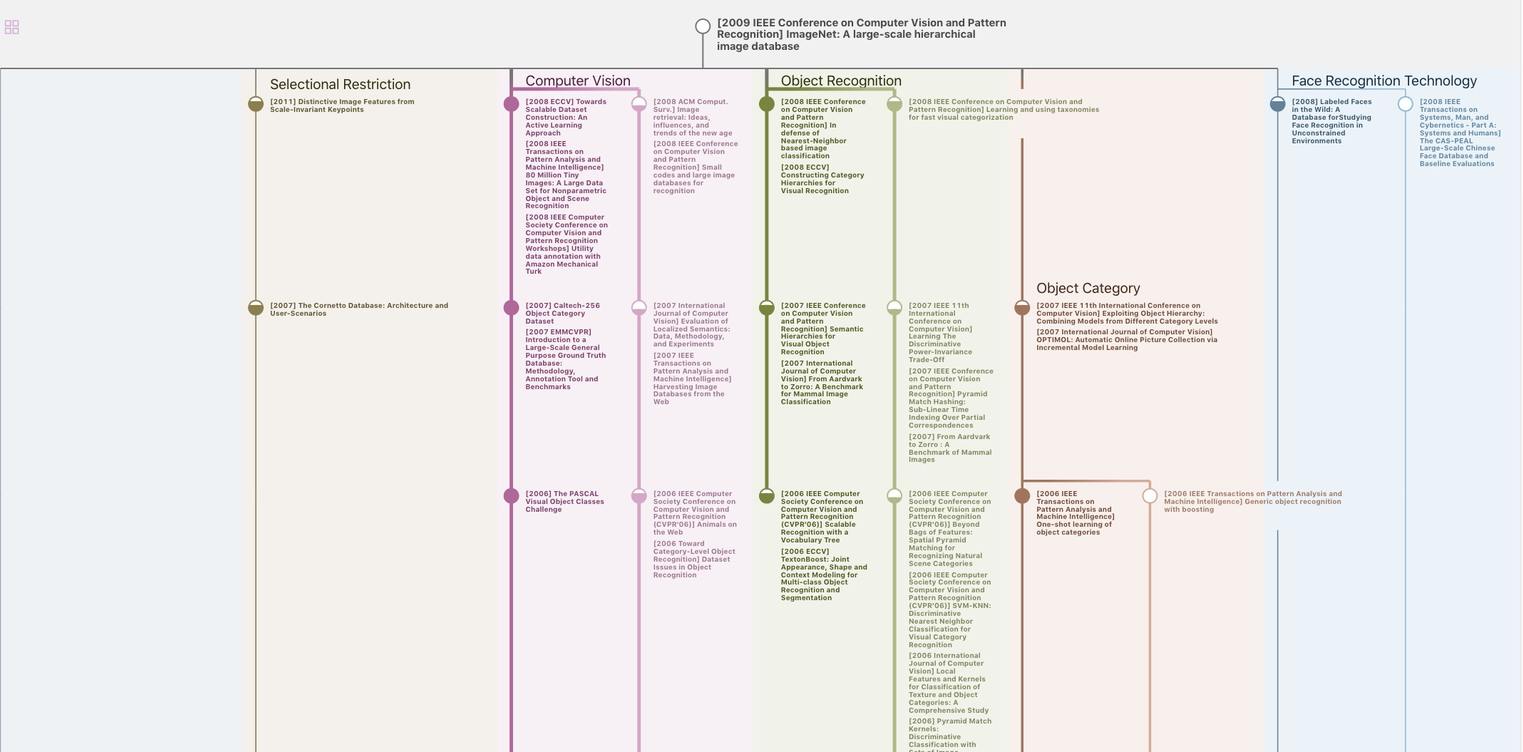
生成溯源树,研究论文发展脉络
Chat Paper
正在生成论文摘要