Subspace-constrained approaches to low-rank fMRI acceleration
NeuroImage(2020)
摘要
Acceleration methods in fMRI aim to reconstruct high fidelity images from undersampled k-space, allowing fMRI datasets to achieve higher temporal resolution, reduced physiological noise aliasing, and increased statistical degrees of freedom. While low levels of acceleration are typically part of standard fMRI protocols through parallel imaging, there exists the potential for approaches that allow much greater acceleration. One such existing approach is k-t FASTER, which exploits the inherent low-rank nature of fMRI. In this paper, we present a reformulated version of k-t FASTER which includes additional L2 constraints within a low-rank framework.
We evaluated the effect of three different constraints against existing low-rank approaches to fMRI reconstruction: Tikhonov constraints, low-resolution priors, and temporal subspace smoothness. The different approaches are separately tested for robustness to undersampling and thermal noise levels, in both retrospectively and prospectively-undersampled finger-tapping task fMRI data. Reconstruction quality is evaluated by accurate reconstruction of low-rank subspaces and activation maps.
The use of L2 constraints were found to achieve consistently improved results, producing high fidelity reconstructions of statistical parameter maps at higher acceleration factors and lower SNR values than existing methods, but at a cost of longer computation time. In particular, the Tikhonov constraint proved very robust across all tested datasets, and the temporal subspace smoothness constraint provided the best reconstruction scores in the prospectively-undersampled dataset. These results demonstrate that regularized low-rank reconstruction of fMRI data can recover functional information at high acceleration factors without the use of any model-based spatial constraints.
Highlights
### Competing Interest Statement
The authors have declared no competing interest.
更多查看译文
AI 理解论文
溯源树
样例
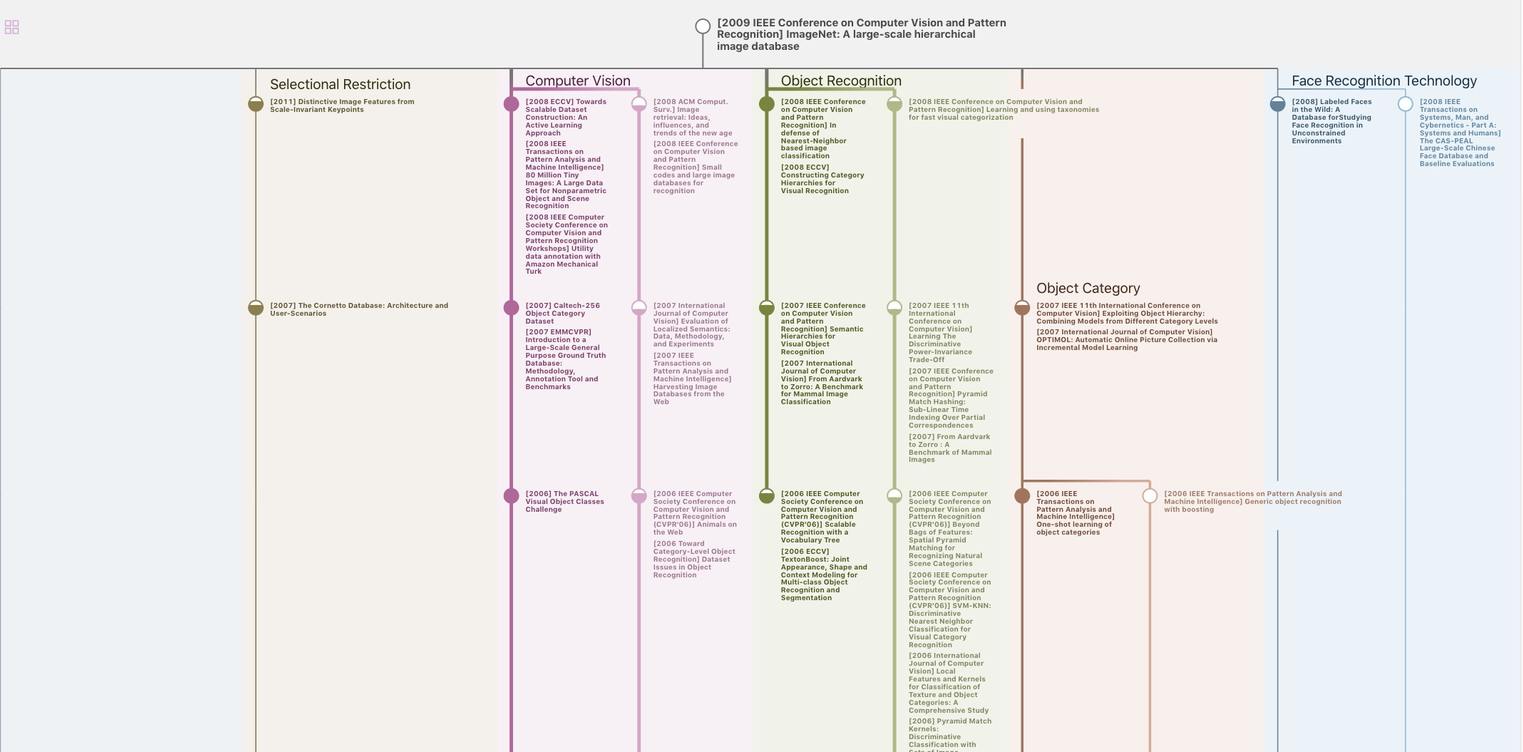
生成溯源树,研究论文发展脉络
Chat Paper
正在生成论文摘要