BERTMHC: improved MHC-peptide class II interaction prediction with transformer and multiple instance learning
BIOINFORMATICS(2021)
摘要
Motivation: Increasingly comprehensive characterization of cancer-associated genetic alterations has paved the way for the development of highly specific therapeutic vaccines. Predicting precisely the binding and presentation of peptides to major histocompatibility complex (MHC) alleles is an important step toward such therapies. Recent data suggest that presentation of both class I and II epitopes are critical for the induction of a sustained effective immune response. However, the prediction performance for MHC class II has been limited compared to class I. Results: We present a transformer neural network model which leverages self-supervised pretraining from a large corpus of protein sequences. We also propose a multiple instance learning (MIL) framework to deconvolve mass spectrometry data where multiple potential MHC alleles may have presented each peptide. We show that pretraining boosted the performance for these tasks. Combining pretraining and the novel MIL approach, our model outperforms state-of-the-art models based on peptide and MHC sequence only for both binding and cell surface presentation predictions.
更多查看译文
关键词
interaction,learning,mhc-peptide
AI 理解论文
溯源树
样例
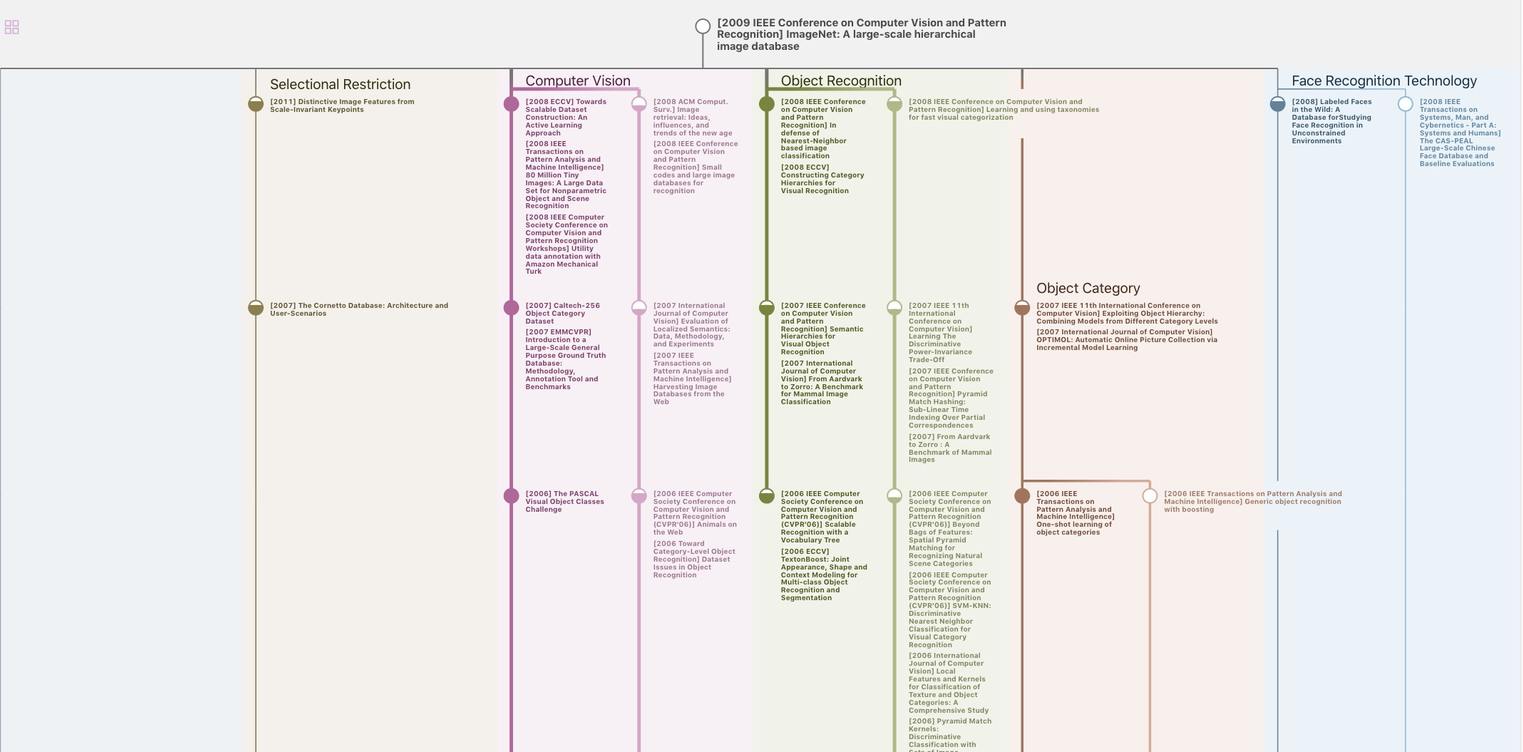
生成溯源树,研究论文发展脉络
Chat Paper
正在生成论文摘要