A Compression-Compilation Framework for On-mobile Real-time BERT Applications.
IJCAI(2021)
摘要
Transformer-based deep learning models have increasingly demonstrated high accuracy on many natural language processing (NLP) tasks. In this paper, we propose a compression-compilation co-design framework that can guarantee the identified model to meet both resource and real-time specifications of mobile devices. Our framework applies a compiler-aware neural architecture optimization method (CANAO), which can generate the optimal compressed model that balances both accuracy and latency. We are able to achieve up to 7.8x speedup compared with TensorFlow-Lite with only minor accuracy loss. We present two types of BERT applications on mobile devices: Question Answering (QA) and Text Generation. Both can be executed in real-time with latency as low as 45ms. Videos for demonstrating the framework can be found on https://www.youtube.com/watch?v=_WIRvK_2PZI
更多查看译文
关键词
compression-compilation,on-mobile,real-time
AI 理解论文
溯源树
样例
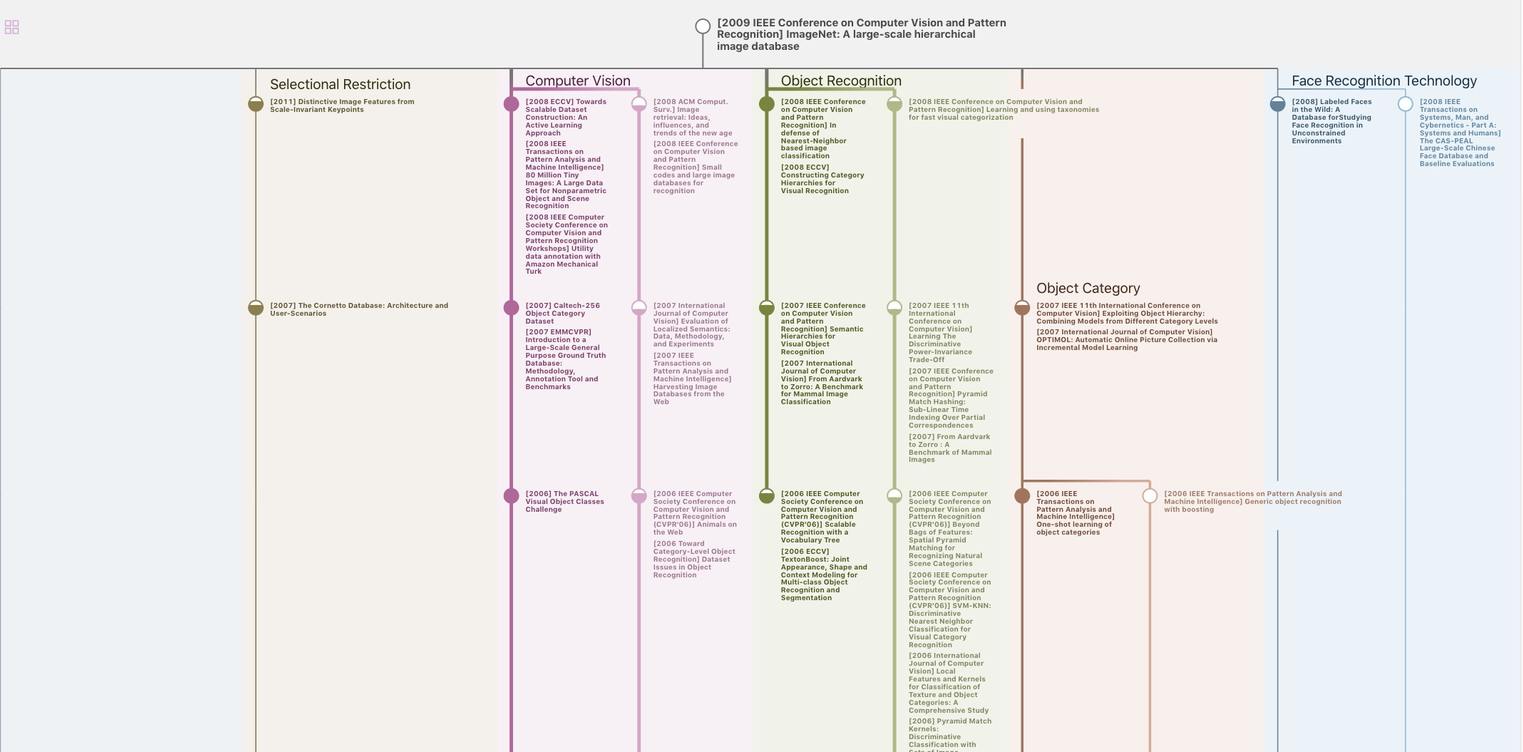
生成溯源树,研究论文发展脉络
Chat Paper
正在生成论文摘要