A Comparison of Pre-Trained Language Models for Multi-Class Text Classification in the Financial Domain
International World Wide Web Conference(2021)
摘要
ABSTRACT Neural networks for language modeling have been proven effective on several sub-tasks of natural language processing. Training deep language models, however, is time-consuming and computationally intensive. Pre-trained language models such as BERT are thus appealing since (1) they yielded state-of-the-art performance, and (2) they offload practitioners from the burden of preparing the adequate resources (time, hardware, and data) to train models. Nevertheless, because pre-trained models are generic, they may underperform on specific domains. In this study, we investigate the case of multi-class text classification, a task that is relatively less studied in the literature evaluating pre-trained language models. Our work is further placed under the industrial settings of the financial domain. We thus leverage generic benchmark datasets from the literature and two proprietary datasets from our partners in the financial technological industry. After highlighting a challenge for generic pre-trained models (BERT, DistilBERT, RoBERTa, XLNet, XLM) to classify a portion of the financial document dataset, we investigate the intuition that a specialized pre-trained model for financial documents, such as FinBERT, should be leveraged. Nevertheless, our experiments show that the FinBERT model, even with an adapted vocabulary, does not lead to improvements compared to the generic BERT models.
更多查看译文
关键词
BERT, FinBERT, financial text classification
AI 理解论文
溯源树
样例
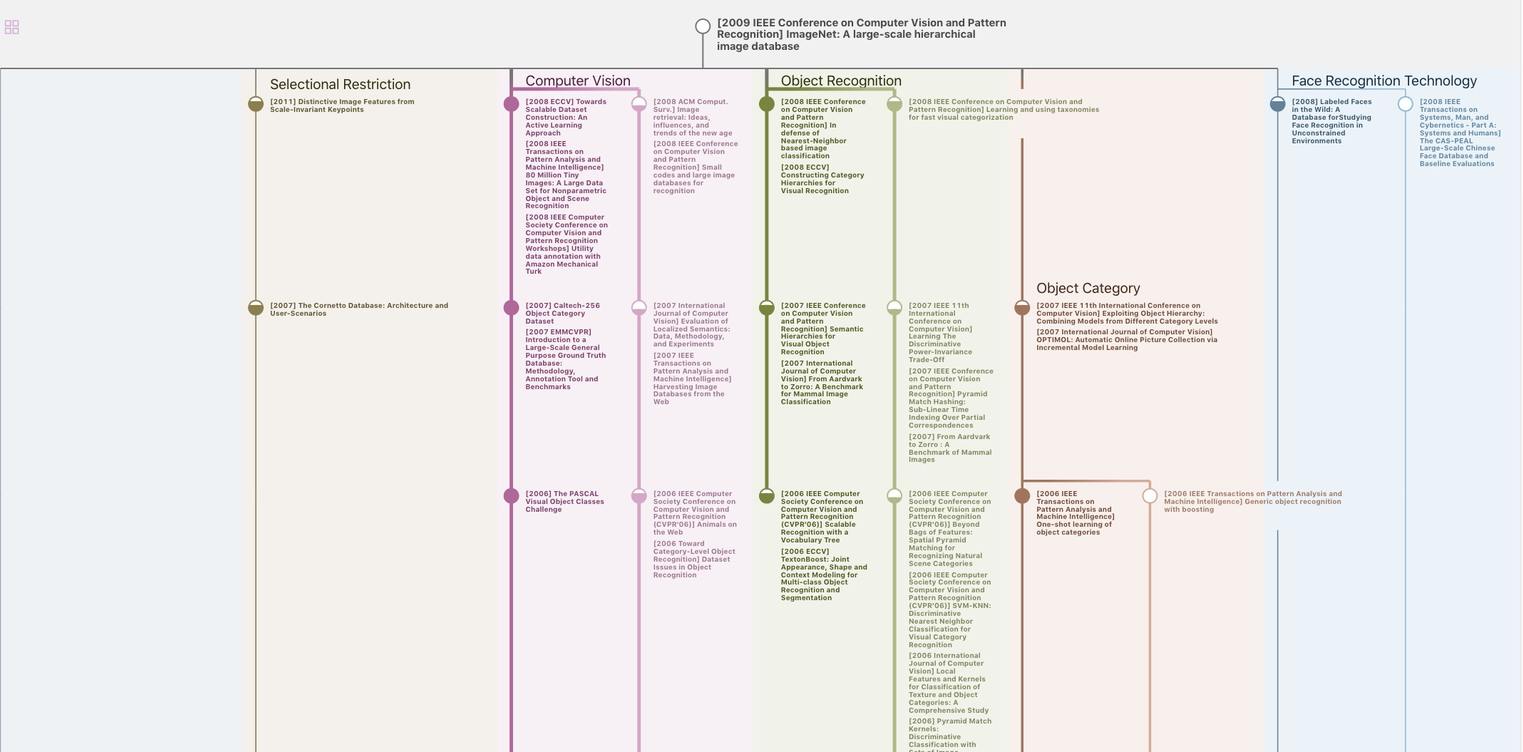
生成溯源树,研究论文发展脉络
Chat Paper
正在生成论文摘要