High-Dimensional Bayesian Optimisation with Variational Autoencoders and Deep Metric Learning
arxiv(2021)
摘要
We introduce a method based on deep metric learning to perform Bayesian optimisation over high-dimensional, structured input spaces using variational autoencoders (VAEs). By extending ideas from supervised deep metric learning, we address a longstanding problem in high-dimensional VAE Bayesian optimisation, namely how to enforce a discriminative latent space as an inductive bias. Importantly, we achieve such an inductive bias using just 1% of the available labelled data relative to previous work, highlighting the sample efficiency of our approach. As a theoretical contribution, we present a proof of vanishing regret for our method. As an empirical contribution, we present state-of-the-art results on real-world high-dimensional black-box optimisation problems including property-guided molecule generation. It is the hope that the results presented in this paper can act as a guiding principle for realising effective high-dimensional Bayesian optimisation.
更多查看译文
关键词
deep metric learning,variational autoencoders,high-dimensional
AI 理解论文
溯源树
样例
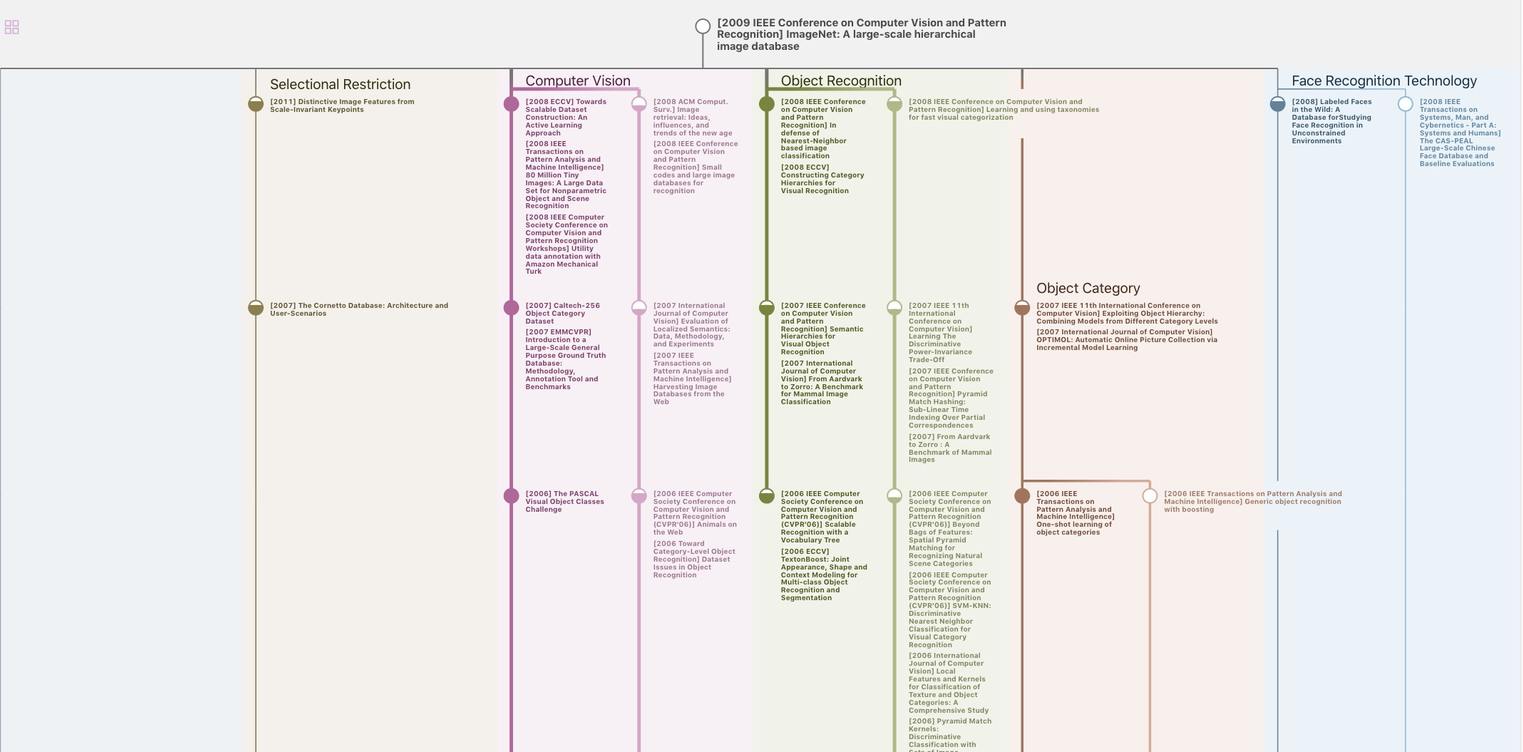
生成溯源树,研究论文发展脉络
Chat Paper
正在生成论文摘要