Deep Canonical Correlation Alignment for Sensor Signals
arxiv(2021)
摘要
Sensor technology is becoming increasingly prevalent across a multitude of fields and industries. As a result, simultaneous recordings of multiple inter-correlated signals is becoming increasingly common. With this, more problems of a practical nature emerge due to sensor clock-drift, offsets, and other complications. Processing of multiple sensor data is often dependent on the data being properly aligned in the temporal dimension. The alignment process is a necessary step before the data can be evaluated properly but it is a time consuming process, often involving significant manual labor and expertise. Regularly used methods to align sensor signals have trouble addressing real-world issues such as morphological dissimilarities, excessive noise, or very long, raw sensor signals. In this work, we present Deep Canonical Correlation Sensor Alignment (DCCA), a method that is specifically tailored to address these problems. It exploits common properties specific to misalignments produced by sensor circuitry, such as clock-drift and offsets. On a selection of artificial and real datasets we demonstrate the performance of DCCA under a variety of conditions.
更多查看译文
关键词
sensor signals,correlation
AI 理解论文
溯源树
样例
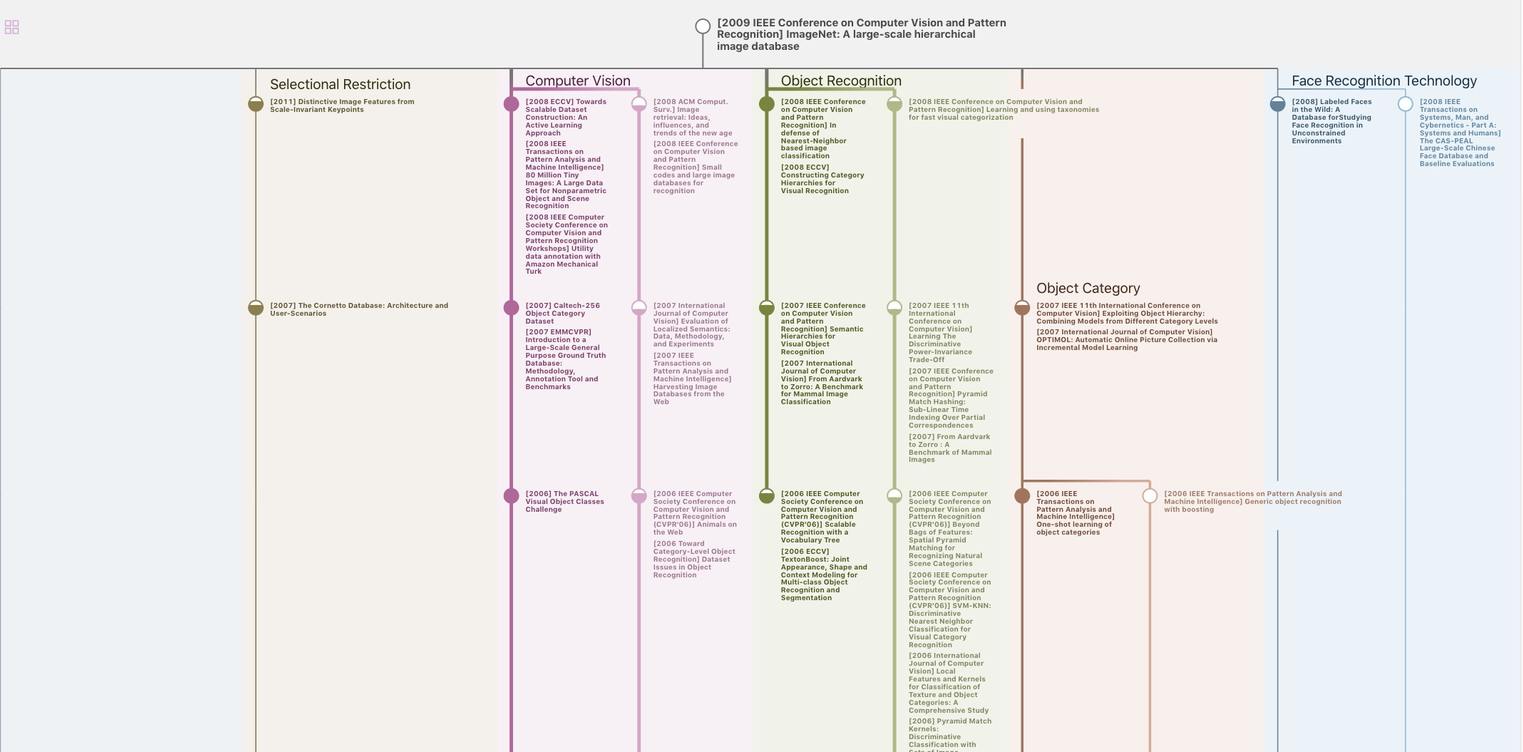
生成溯源树,研究论文发展脉络
Chat Paper
正在生成论文摘要