Parallel Batch-Dynamic k-Core Decomposition
ArXiv(2021)
摘要
Maintaining a k-core decomposition quickly in a dynamic graph is an important problem in many applications, including social network analytics, graph visualization, centrality measure computations, and community detection algorithms. The main challenge for designing efficient k-core decomposition algorithms is that a single change to the graph can cause the decomposition to change significantly. We present the first parallel batch-dynamic algorithm for maintaining an approximate k-core decomposition that is efficient in both theory and practice. Given an initial graph withm edges, and a batch of B updates, our algorithmmaintains a (2+δ)-approximation of the coreness values for all vertices (for any constant δ > 0) in O (B log2m) amortized work and O (log2m log logm) depth (parallel time) with high probability. Our algorithm also maintains a low out-degree orientation of the graph in the same bounds. We implemented and experimentally evaluated our algorithm on a 30-core machine with two-way hyper-threading on 11 graphs of varying densities and sizes. Compared to the state-of-the-art algorithms, our algorithm achieves up to a 114.52x speedup against the best multicore implementation and up to a 497.63x speedup against the best sequential algorithm, obtaining results for graphs that are orders-of-magnitude larger than those used in previous studies. In addition, we present the first approximate static k-core algorithm with linear work and polylogarithmic depth. We show that on a 30-core machine with two-way hyper-threading, our implementation achieves up to a 3.9x speedup in the static case over the previous state-of-the-art parallel algorithm. Artifact Availability: The source code, data, and/or other artifacts have been made available at https://github.com/qqliu/batch-dynamic-kcore-decomposition/.
更多查看译文
关键词
decomposition,batch-dynamic,k-core
AI 理解论文
溯源树
样例
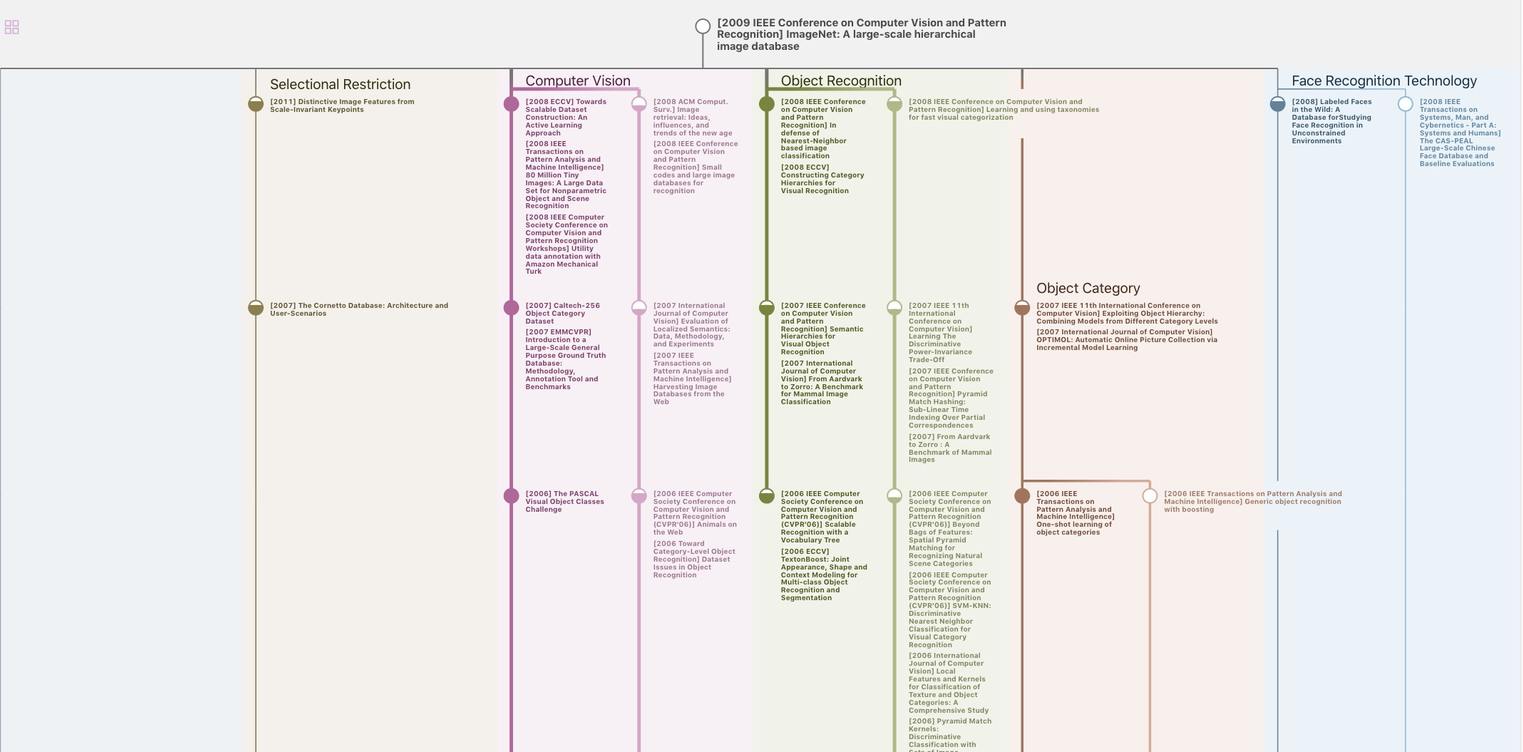
生成溯源树,研究论文发展脉络
Chat Paper
正在生成论文摘要