Autonomous State Inference For Data-Driven Optimization Of Neural Modulation
2021 10TH INTERNATIONAL IEEE/EMBS CONFERENCE ON NEURAL ENGINEERING (NER)(2021)
摘要
Neural modulation is a fundamental tool for treating neurological diseases and understanding their mechanisms. One of the challenges in neural modulation includes selecting stimulation parameters, as parameter spaces are very large and their induced effects can exhibit complex behavior. Moreover, the effect of stimulation may depend on the underlying neural state, which can be difficult or impossible to quantify a priori. In this study, we first use an unsupervised learning approach to demonstrate that the effect of medial septum optogenetic stimulation on hippocampal activity differs between awake and anesthetized behavioral states. We then use these data to construct a simulation model of a neural modulation experiment and demonstrate a novel Bayesian optimization method that automatically learns the subject-specific relationship between neural state and its effect on modulation. This approach outperformed standard Bayesian optimization and identified ground-truth optimal parameters of the simulation model, suggesting that this method can efficiently explore complex state-dependent relationships of parameter spaces to improve neural modulation.
更多查看译文
关键词
awake states,anesthetized behavioral states,neural modulation experiment,identified ground-truth optimal parameters,complex state-dependent relationships,parameter spaces,autonomous state inference,data-driven optimization,stimulation parameters,induced effects,underlying neural state,medial septum optogenetic stimulation
AI 理解论文
溯源树
样例
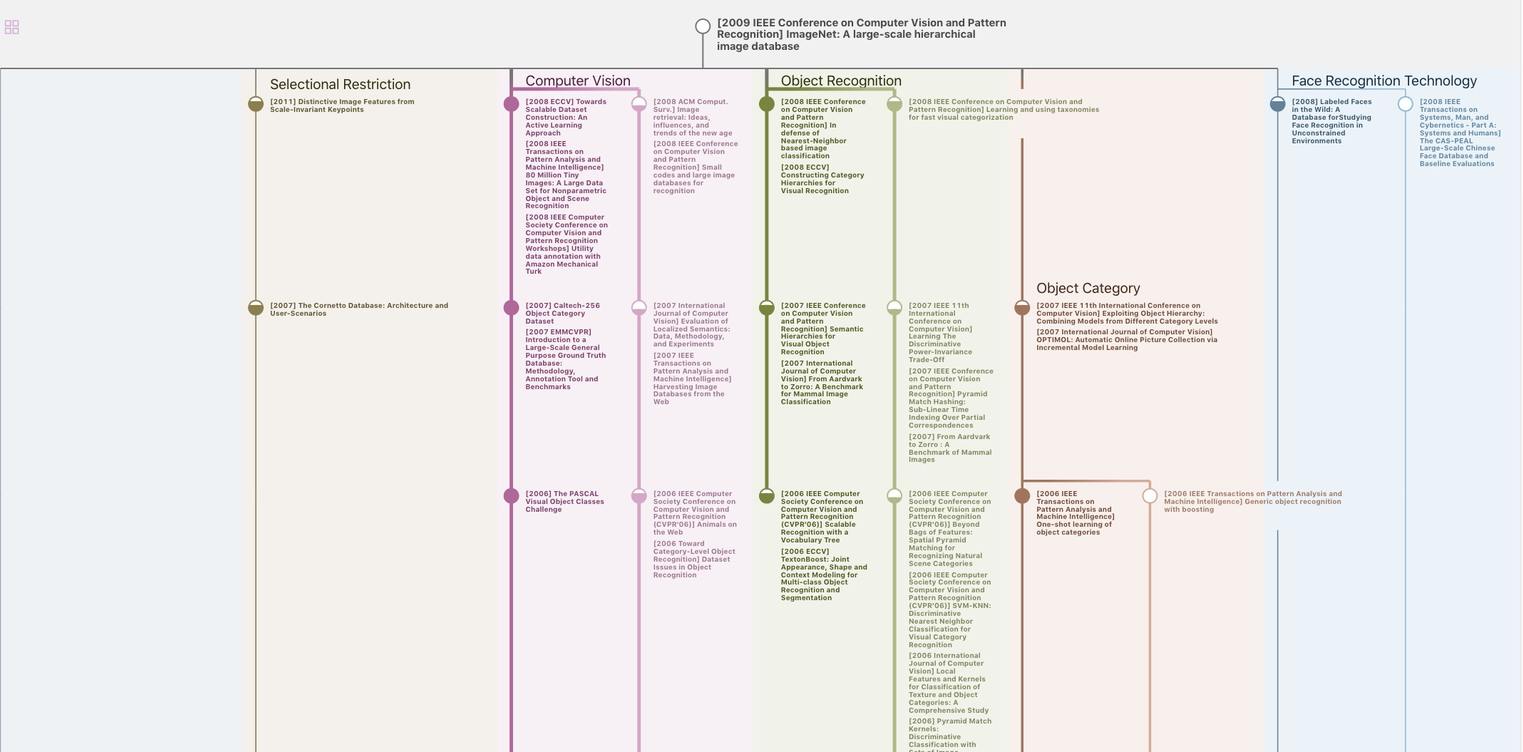
生成溯源树,研究论文发展脉络
Chat Paper
正在生成论文摘要