Dynamic Skill Selection for Learning Joint Actions.
AAMAS(2021)
摘要
Learning in tightly coupled multiagent settings with sparse rewards is challenging because multiple agents must reach the goal state simultaneously for the team to receive a reward. This is even more challenging under temporal coupling constraints - where agents need to sequentially complete different components of a task in a particular order. Here, a single local reward is inadequate for learning an effective policy. We introduce MADyS, Multiagent Learning via Dynamic Skill Selection, a bi-level optimization framework that learns to dynamically switch between multiple local skills to optimize sparse team objectives. MADyS adopts fast policy gradients to learn local skills using local rewards and an evolutionary algorithm to optimize the sparse team objective by recruiting the most optimal skill at any given time. This eliminates the need to generate a single dense reward via reward shaping or other mixing functions. In environments with both spatial and temporal coupling requirements, we outperform prior methods and provides intuitive visualizations of its skill switching strategy.
更多查看译文
AI 理解论文
溯源树
样例
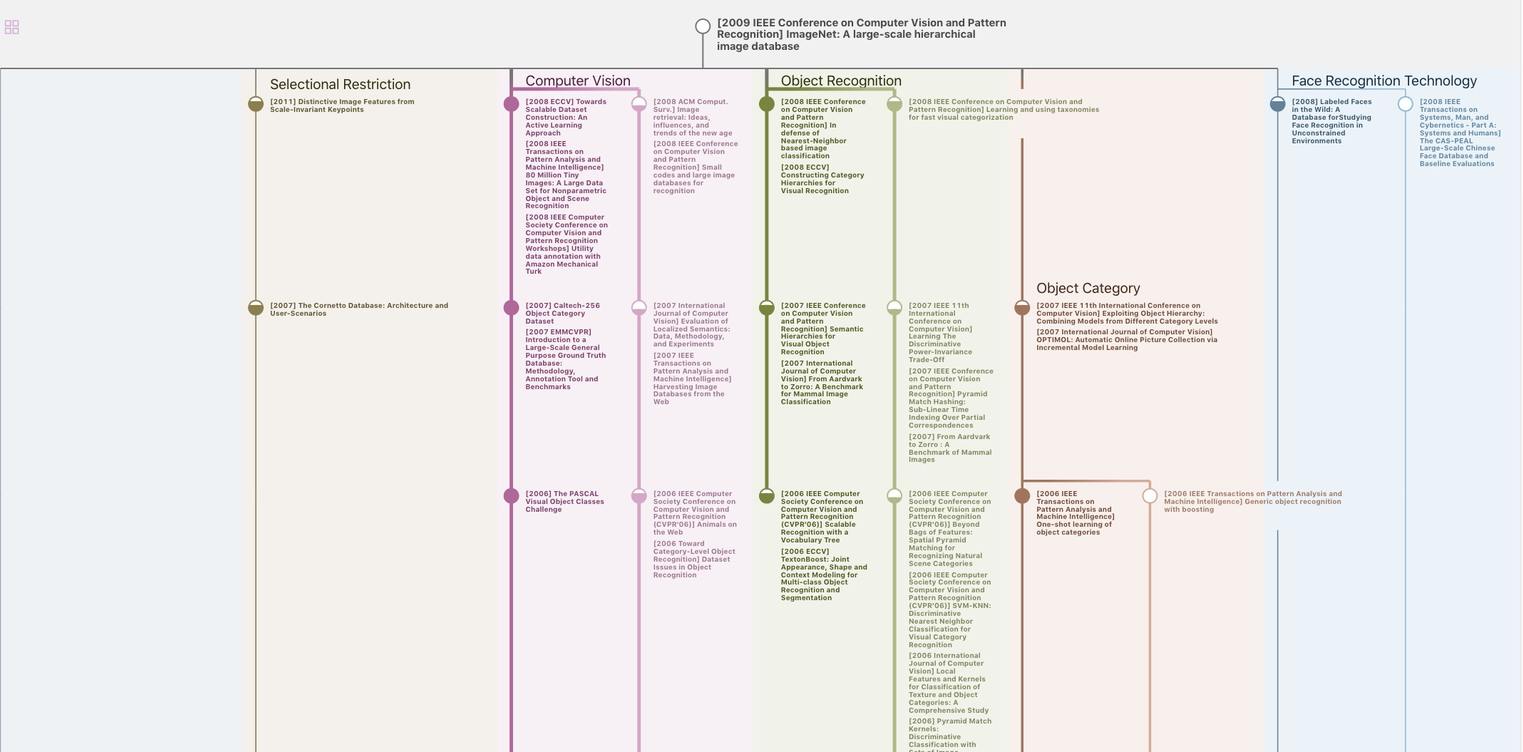
生成溯源树,研究论文发展脉络
Chat Paper
正在生成论文摘要