Eliciting Fairness in Multiplayer Bargaining through Network-Based Role Assignment.
AAMAS(2021)
摘要
From employment contracts to climate agreements, individuals often engage in groups that must reach decisions with varying levels of fairness. These dilemmas also pervade AI, e.g. in automated negotiation, conflict resolution or resource allocation. As evidenced by the Ultimatum Game, payoff maximization is frequently at odds with fairness. Eliciting equality in populations of self-regarding agents thus requires judicious interventions. Here we use knowledge about agents' social networks to implement fairness mechanisms, in the context of Multiplayer Ultimatum Games. We show that preferentially attributing the role of Proposer to low-connected nodes enhances fairness. We further show that, when high-degree must be the Proposers, stricter voting rules (i.e., requiring consensus for collectives to accept a proposal) reduce unfairness.
更多查看译文
AI 理解论文
溯源树
样例
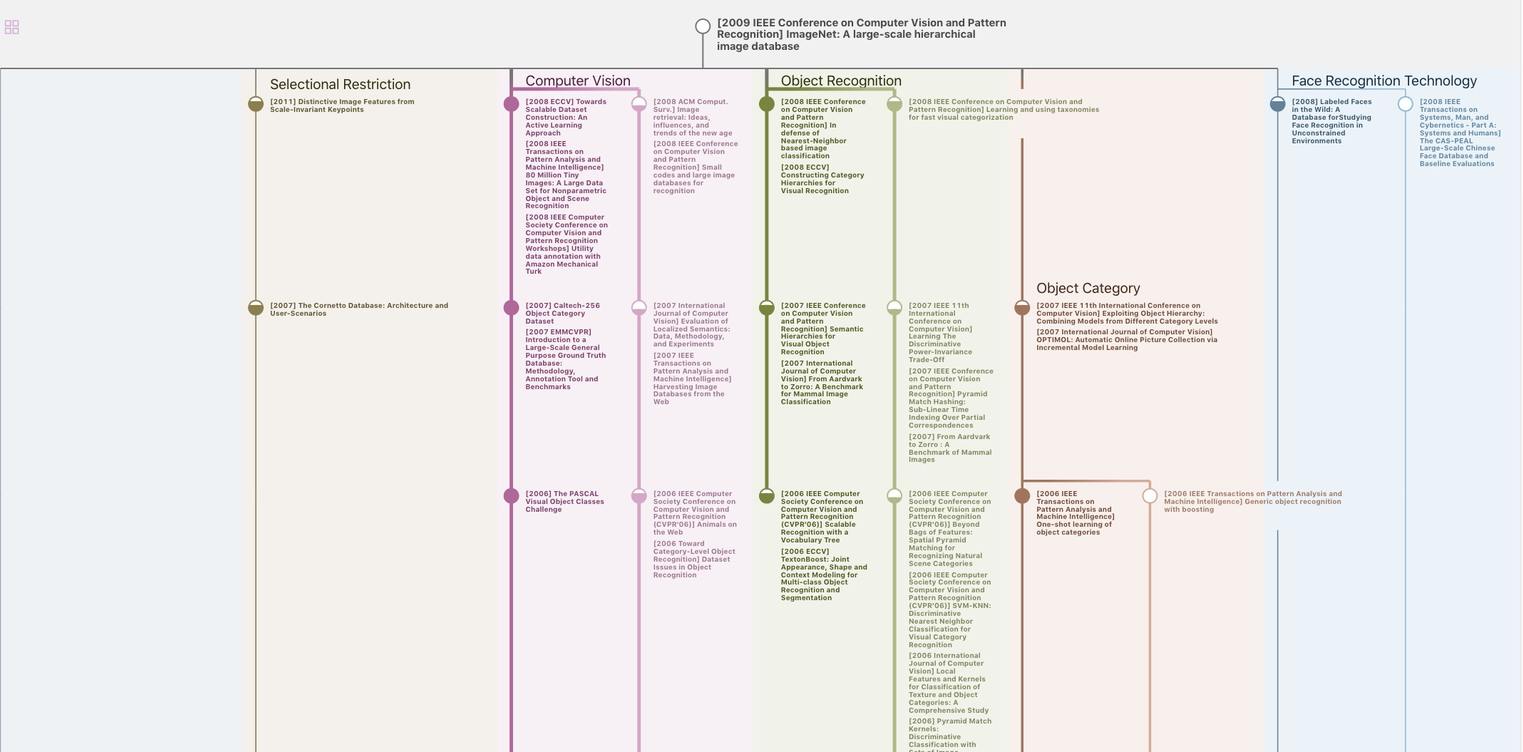
生成溯源树,研究论文发展脉络
Chat Paper
正在生成论文摘要