AutoFT: Automatic Fine-Tune for Parameters Transfer Learning in Click-Through Rate Prediction
arxiv(2021)
摘要
Recommender systems are often asked to serve multiple recommendation scenarios or domains. Fine-tuning a pre-trained CTR model from source domains and adapting it to a target domain allows knowledge transferring. However, optimizing all the parameters of the pre-trained network may result in over-fitting if the target dataset is small and the number of parameters is large. This leads us to think of directly reusing parameters in the pre-trained model which represent more general features learned from multiple domains. However, the design of freezing or fine-tuning layers of parameters requires much manual effort since the decision highly depends on the pre-trained model and target instances. In this work, we propose an end-to-end transfer learning framework, called Automatic Fine-Tuning (AutoFT), for CTR prediction. AutoFT consists of a field-wise transfer policy and a layer-wise transfer policy. The field-wise transfer policy decides how the pre-trained embedding representations are frozen or fine-tuned based on the given instance from the target domain. The layer-wise transfer policy decides how the high?order feature representations are transferred layer by layer. Extensive experiments on two public benchmark datasets and one private industrial dataset demonstrate that AutoFT can significantly improve the performance of CTR prediction compared with state-of-the-art transferring approaches.
更多查看译文
关键词
parameters transfer learning,transfer learning,prediction,fine-tune,click-through
AI 理解论文
溯源树
样例
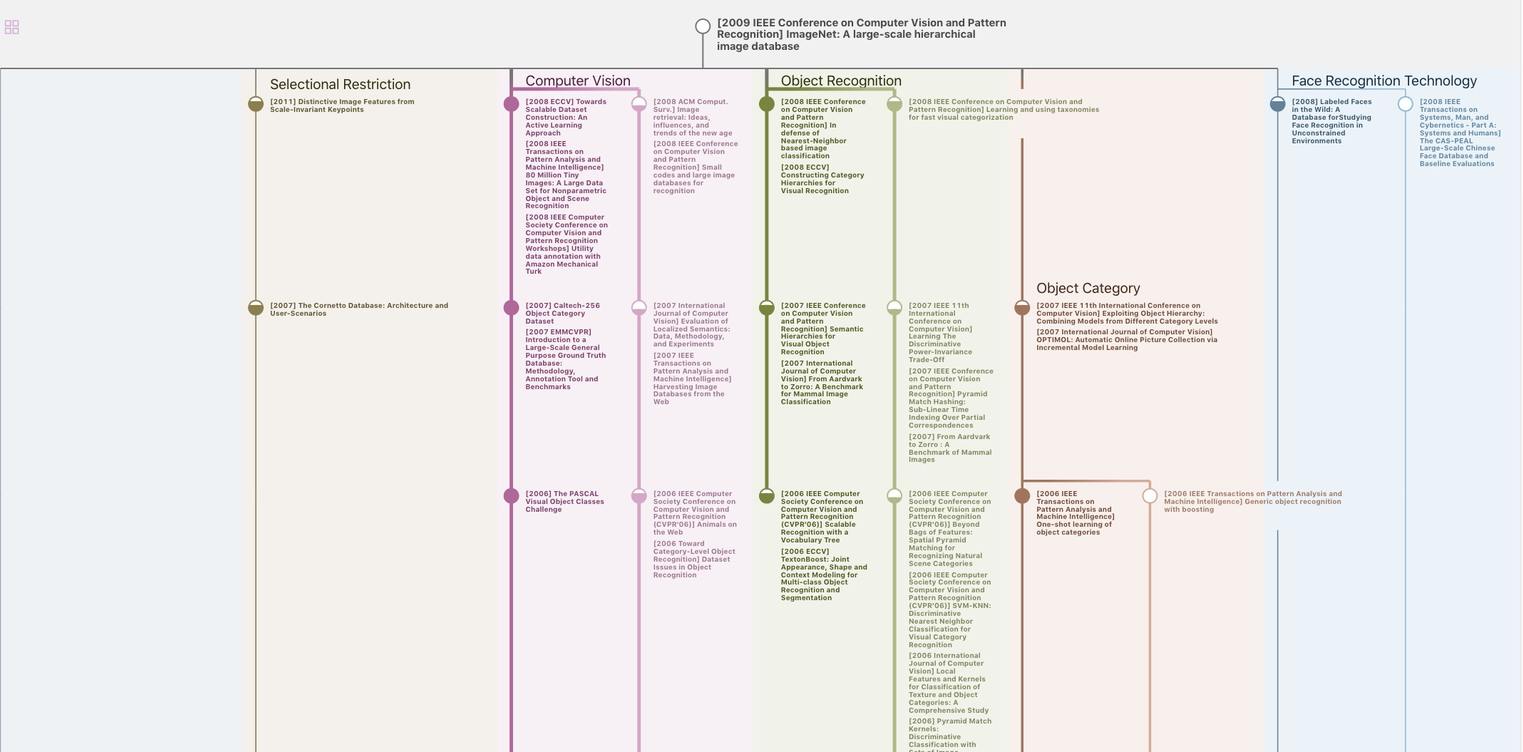
生成溯源树,研究论文发展脉络
Chat Paper
正在生成论文摘要