Augmented multiplexed asymmetric-detection time-stretch optical microscopy by generative deep learning
user-5d8054e8530c708f9920ccce(2021)
摘要
We report a robust method based on generative deep learning to reconstruct quantitative phase image (QPI). By employing multiplexed asymmetric-detection time-stretch optical microscopy (multi-ATOM), we simultaneously captured multiple intensity image contrasts of the same cell in microfluidic flow, revealing different phase-gradient orientations at high throughput (10,000 cells/sec). Using conditional generative adversarial networks (cGAN), we performed a systematic analysis of how different orientations of the phase-gradient contrasts and their combinations influence the QPI prediction performance, which overall general achieves a high similarity (structural similarity index > 0.91) and low error rate (mean squared error < 0.01).
更多查看译文
关键词
Mean squared error,Word error rate,Deep learning,Similarity (network science),Iterative reconstruction,Throughput (business),Multiplexing,Pattern recognition,Structural similarity,Computer science,Artificial intelligence
AI 理解论文
溯源树
样例
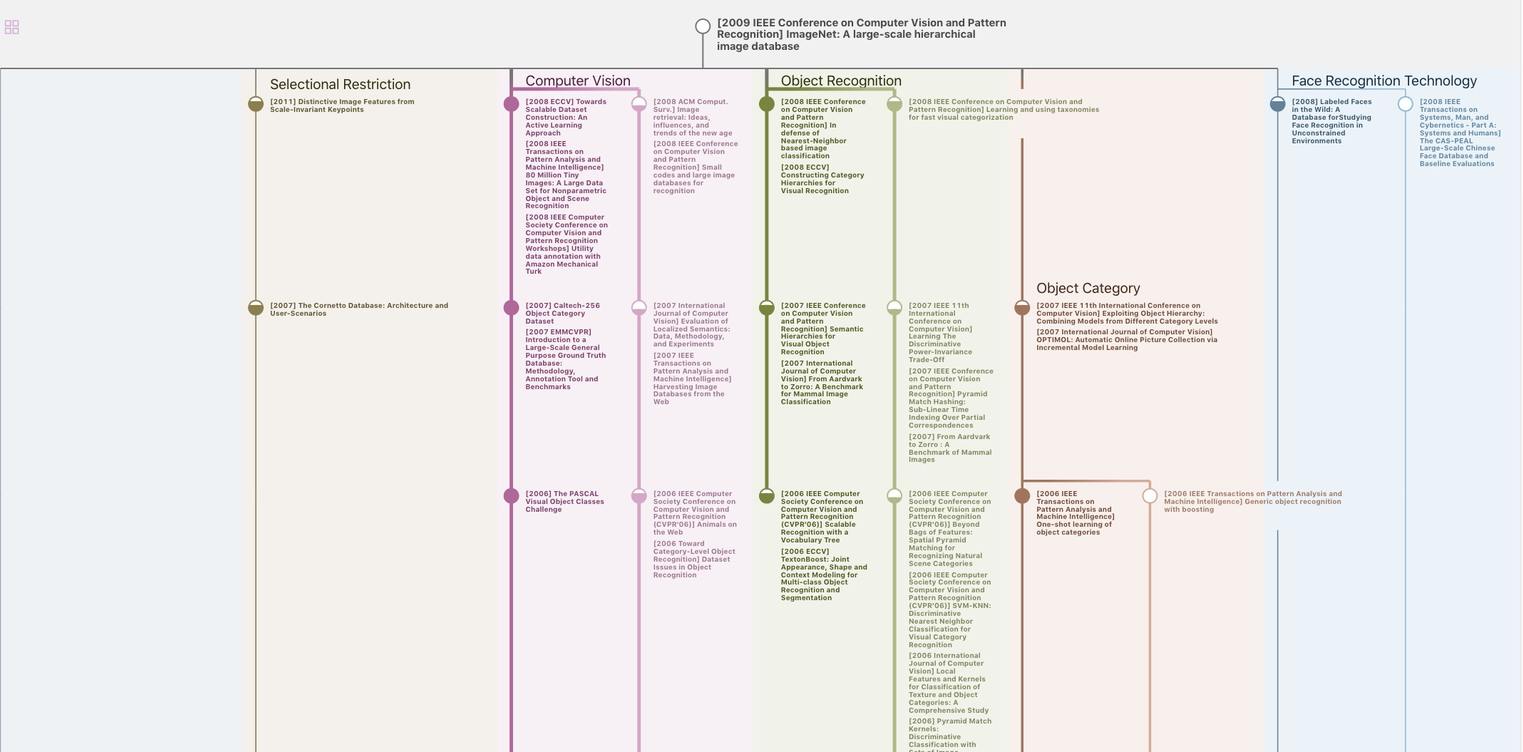
生成溯源树,研究论文发展脉络
Chat Paper
正在生成论文摘要