SLMAD: Statistical Learning-Based Metric Anomaly Detection
SERVICE-ORIENTED COMPUTING, ICSOC 2020(2020)
摘要
Technology companies have become increasingly data-driven, collecting and monitoring a growing list of metrics, such as response time, throughput, page views, and user engagement. With hundreds of metrics in a production environment, an automated approach is needed to detect anomalies and alert potential incidents in real-time. In this paper, we develop a time series anomaly detection framework called Statistical Learning-Based Metric Anomaly Detection (SLMAD) that allows for the detection of anomalies from key performance indicators (KPIs) in streaming time series data. We demonstrate the integrated workflow and algorithms of our anomaly detection framework, which is designed to be accurate, efficient, unsupervised, online, robust, and generalisable. Our approach consists of a three-stage pipeline including analysis of time series, dynamic grouping, and model training and evaluation. The experimental results show that the SLMAD can accurately detect anomalies on a number of benchmark data sets and Huawei production data while maintaining efficient use of resources.
更多查看译文
关键词
Anomaly detection, Unsupervised learning, Online machine learning, Streaming time series, Cloud computing
AI 理解论文
溯源树
样例
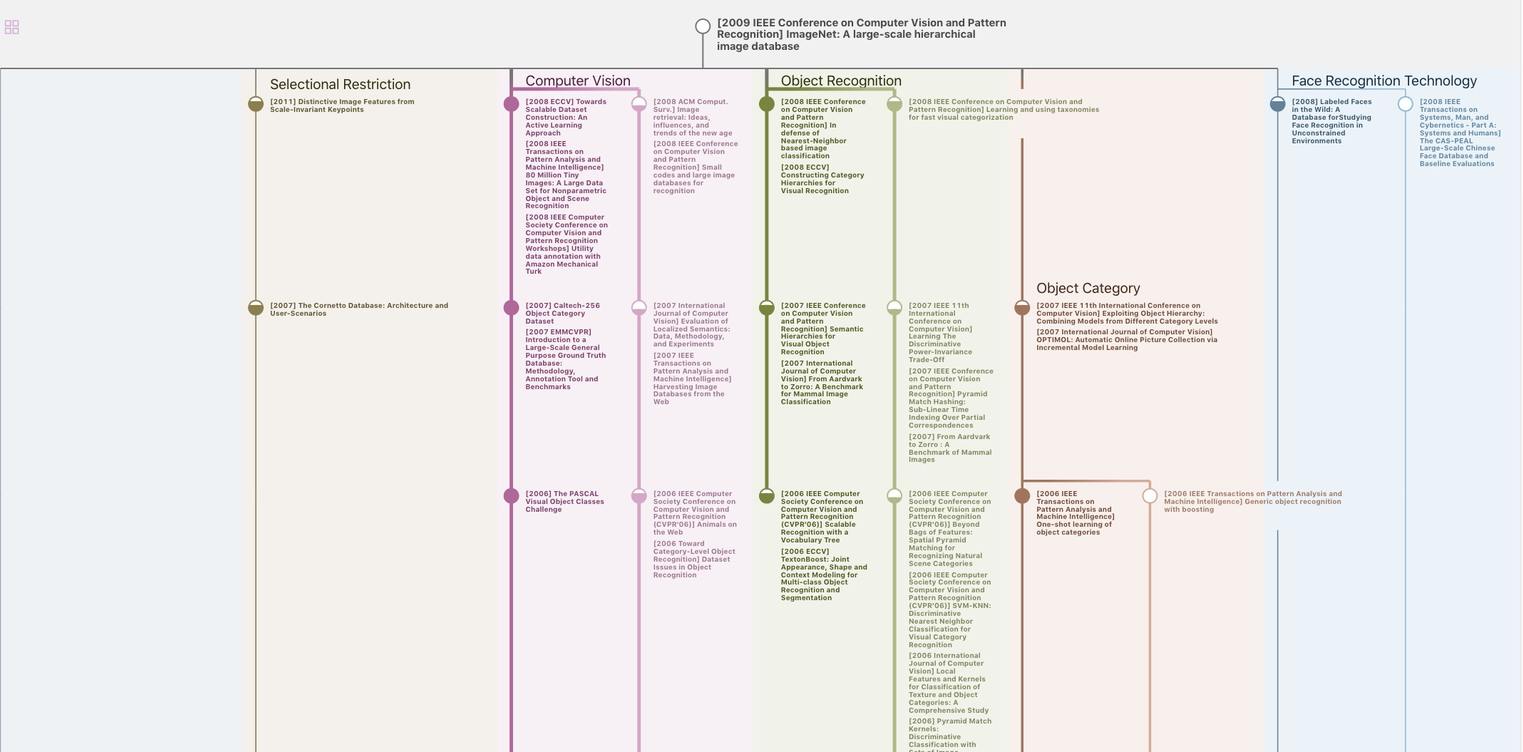
生成溯源树,研究论文发展脉络
Chat Paper
正在生成论文摘要