Performance Diagnosis in Cloud Microservices Using Deep Learning
SERVICE-ORIENTED COMPUTING, ICSOC 2020(2020)
摘要
Microservice architectures are increasingly adopted to design large-scale applications. However, the highly distributed nature and complex dependencies of microservices complicate automatic performance diagnosis and make it challenging to guarantee service level agreements (SLAs). In particular, identifying the culprits of a microservice performance issue is extremely difficult as the set of potential root causes is large and issues can manifest themselves in complex ways. This paper presents an application-agnostic system to locate the culprits for microservice performance degradation with fine granularity, including not only the anomalous service from which the performance issue originates but also the culprit metrics that correlate to the service abnormality. Our method first finds potential culprit services by constructing a service dependency graph and next applies an autoencoder to identify abnormal service metrics based on a ranked list of reconstruction errors. Our experimental evaluation based on injection of performance anomalies to a microservice benchmark deployed in the cloud shows that our system achieves a good diagnosis result, with 92% precision in locating culprit service and 85.5% precision in locating culprit metrics.
更多查看译文
关键词
Performance diagnosis, Root cause analysis, Microservices, Cloud computing, Autoencoder
AI 理解论文
溯源树
样例
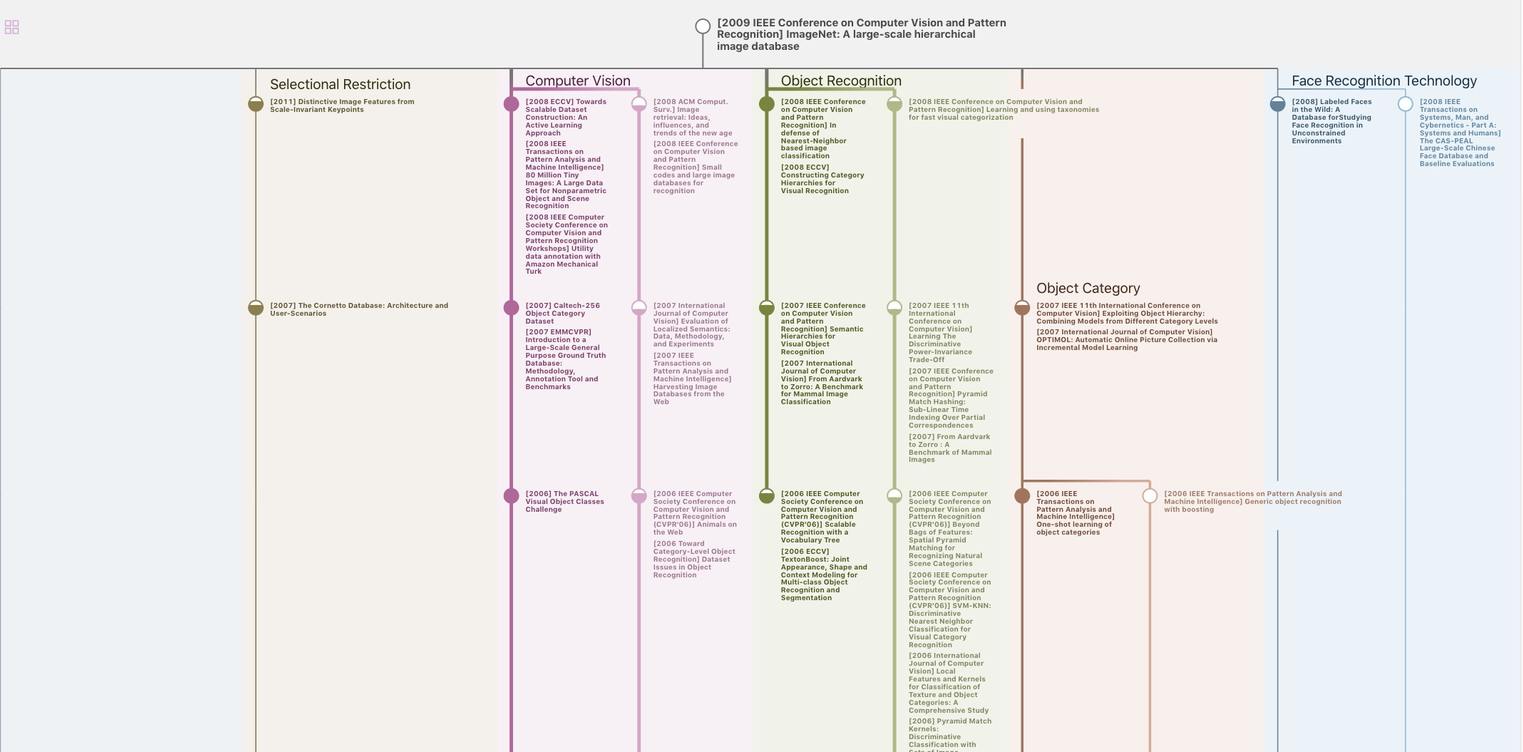
生成溯源树,研究论文发展脉络
Chat Paper
正在生成论文摘要