Seasonality in Infection Predictions Using Interpretable Models for High Dimensional Imbalanced Datasets.
AIME(2021)
摘要
Seasonality plays a significant role in the prevalence of infectious diseases. We evaluate the performance of different approaches used to deal with seasonality in clinical prediction models, including a new proposal based on sliding windows. Class imbalance, high dimensionality and interpretable models are also considered since they are common traits of clinical datasets. We tested these approaches with four datasets: two created synthetically and two extracted from the MIMIC-III database. Our results corroborate that clinical prediction models for infections can be improved by considering the effect of seasonality. However, the techniques employed to obtain the best results are highly dependent on the dataset.
更多查看译文
关键词
Seasonality,Concept drift,Clinical prediction models
AI 理解论文
溯源树
样例
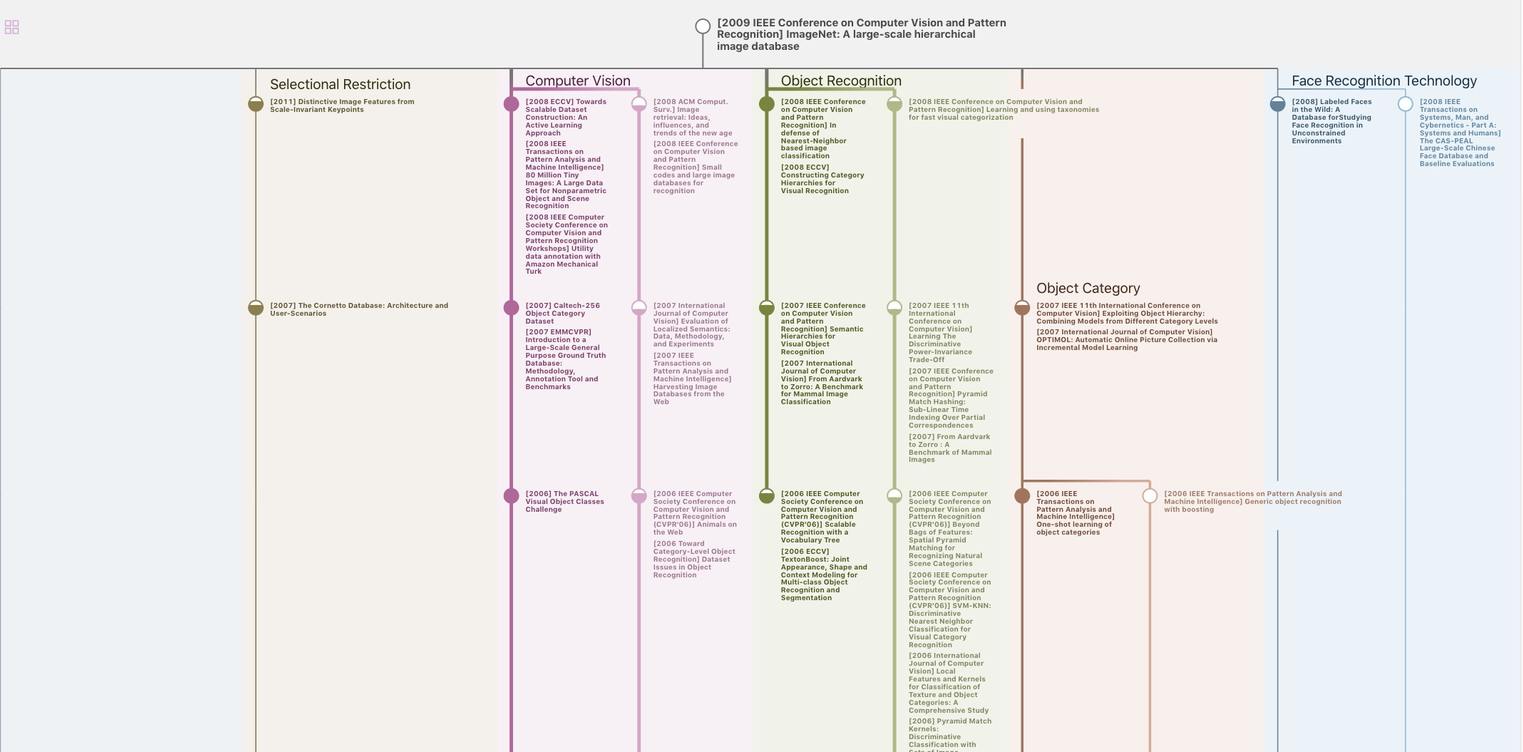
生成溯源树,研究论文发展脉络
Chat Paper
正在生成论文摘要