Neural UpFlow: A Scene Flow Learning Approach to Increase the Apparent Resolution of Particle-Based Liquids
Proceedings of the ACM on Computer Graphics and Interactive Techniques(2021)
摘要
AbstractWe present a novel up-resing technique for generating high-resolution liquids based on scene flow estimation using deep neural networks. Our approach infers and synthesizes small- and large-scale details solely from a low-resolution particle-based liquid simulation. The proposed network leverages neighborhood contributions to encode inherent liquid properties throughout convolutions. We also propose a particle-based approach to interpolate between liquids generated from varying simulation discretizations using a state-of-the-art bidirectional optical flow solver method for fluids in addition with a novel key-event topological alignment constraint. In conjunction with the neighborhood contributions, our loss formulation allows the inference model throughout epochs to reward important differences in regard to significant gaps in simulation discretizations. Even when applied in an untested simulation setup, our approach is able to generate plausible high-resolution details. Using this interpolation approach and the predicted displacements, our approach combines the input liquid properties with the predicted motion to infer semi-Lagrangian advection. We furthermore showcase how the proposed interpolation approach can facilitate generating large simulation datasets with a subset of initial condition parameters.
更多查看译文
关键词
fluid simulation, particle-based liquid, deformation field, optical flow, up-resing, machine learning, deep neural network
AI 理解论文
溯源树
样例
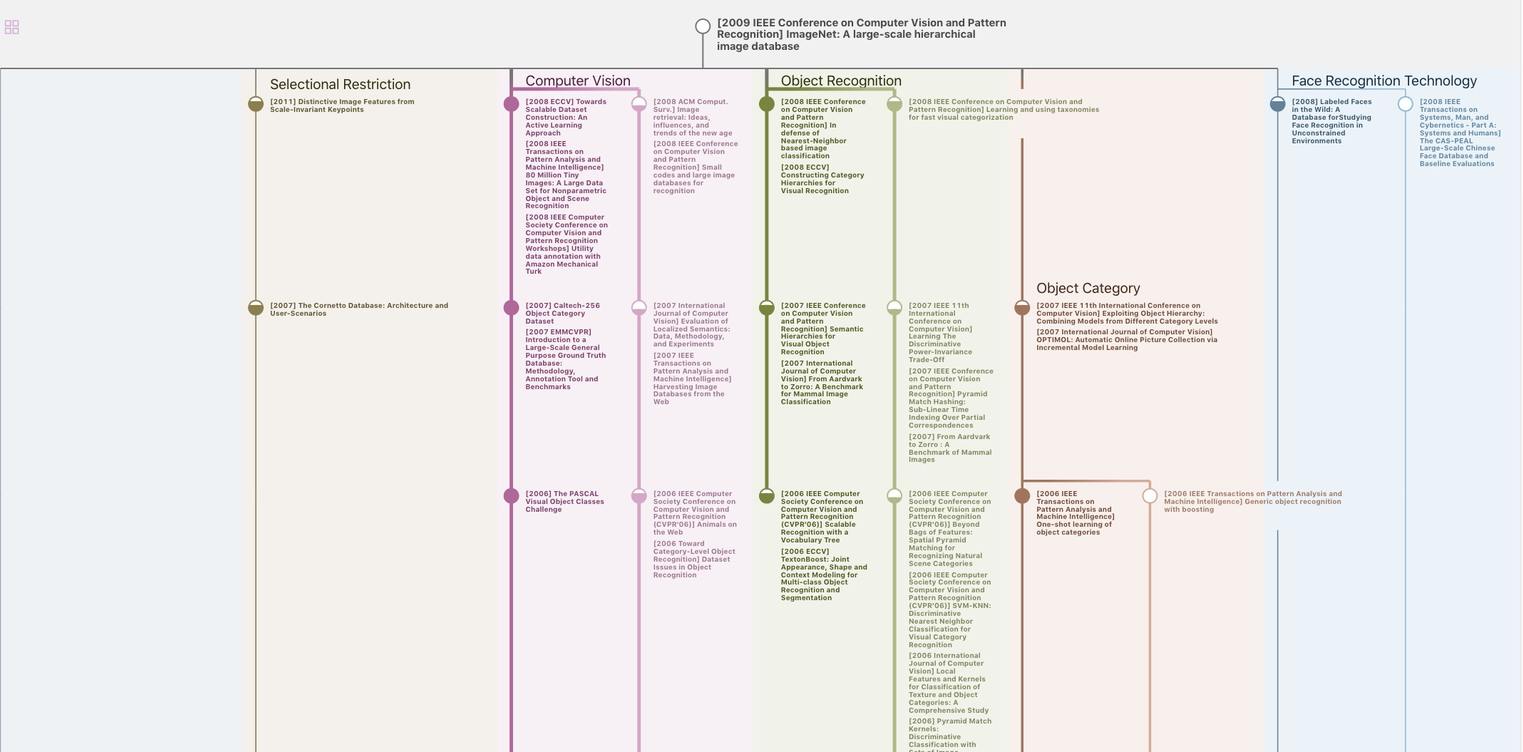
生成溯源树,研究论文发展脉络
Chat Paper
正在生成论文摘要