Augmenting aer2vec: Enriching distributed representations of adverse event report data with orthographic and lexical information
Journal of Biomedical Informatics(2021)
摘要
Adverse Drug Events (ADEs) are prevalent, costly, and sometimes preventable. Post-marketing drug surveillance aims to monitor ADEs that occur after a drug is released to market. Reports of such ADEs are aggregated by reporting systems, such as the Food and Drug Administration (FDA) Adverse Event Reporting System (FAERS). In this paper, we consider the topic of how best to represent data derived from reports in FAERS for the purpose of detecting post-marketing surveillance signals, in order to inform regulatory decision making. In our previous work, we developed aer2vec, a method for deriving distributed representations (concept embeddings) of drugs and side effects from ADE reports, establishing the utility of distributional information for pharmacovigilance signal detection. In this paper, we advance this line of research further by evaluating the utility of encoding orthographic and lexical information. We do so by adapting two Natural Language Processing methods, subword embedding and vector retrofitting, which were developed to encode such information into word embeddings. Models were compared for their ability to distinguish between positive and negative examples in a set of manually curated drug/ADE relationships, with both aer2vec enhancements offering advantages in performances over baseline models, and best performance obtained when retrofitting and subword embeddings were applied in concert. In addition, this work demonstrates that models leveraging distributed representations do not require extensive manual preprocessing to perform well on this pharmacovigilance signal detection task, and may even benefit from information that would otherwise be lost during the normalization and standardization process.
更多查看译文
关键词
Pharmacovigilance,Post-marketing surveillance,Natural language processing,Word embeddings,Retrofitting,Subword embeddings
AI 理解论文
溯源树
样例
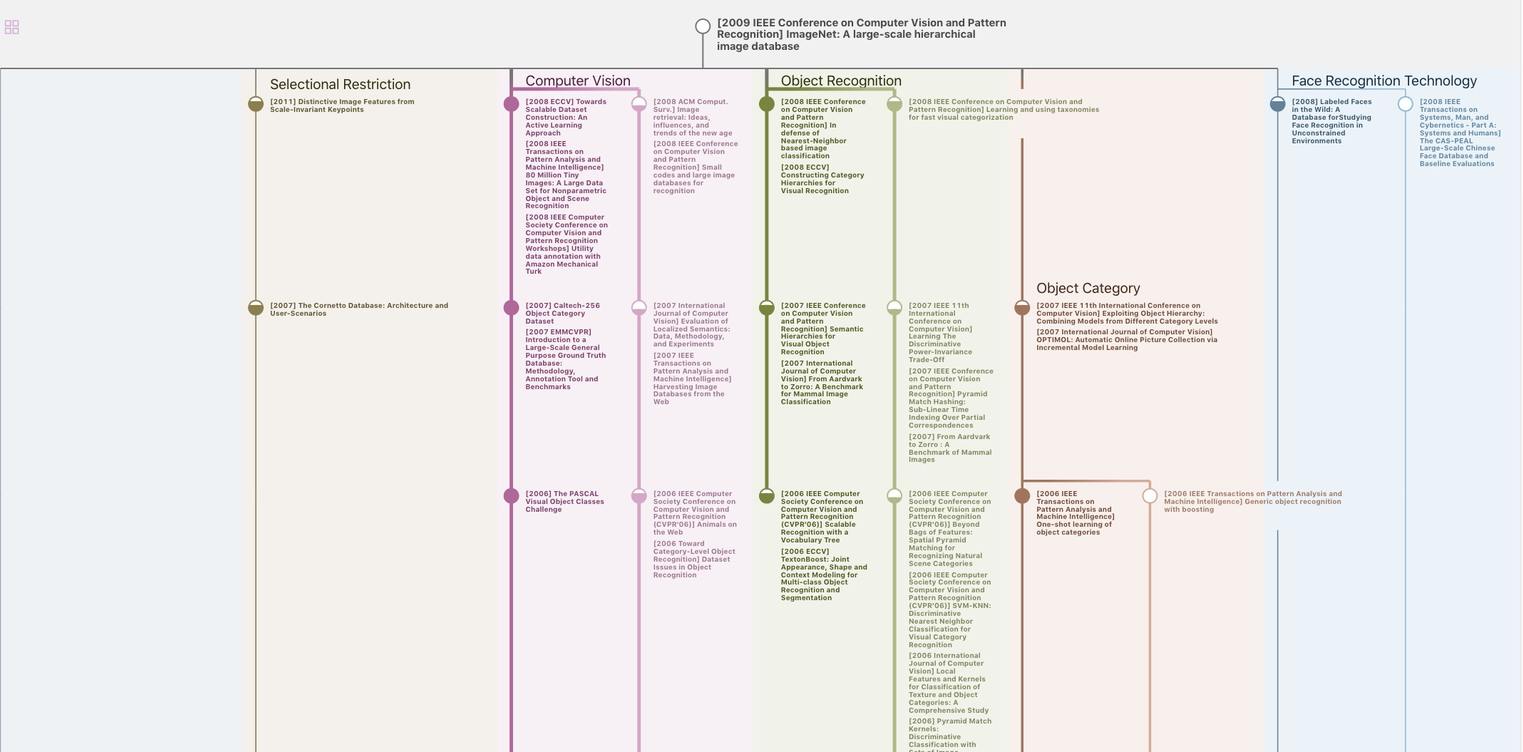
生成溯源树,研究论文发展脉络
Chat Paper
正在生成论文摘要