iPCPA: Interval permutation combination population analysis for spectral wavelength selection
Analytica Chimica Acta(2021)
摘要
As one of the most important preprocessing procedures in spectral detection, wavelength selection approaches play an irreplaceable role in reducing the model overfitting and prediction errors. In this paper, we propose a two-step wavelength selection method called interval permutation combination population analysis (iPCPA), which improves the selective performance by combining three different wavelength selection algorithms. First, interval partial least squares (iPLS) is used as the rough selection step to efficiently exclude the uninformative variables in the spectrum, which reduces the variable space and ensures that the following selection step can focus on selecting informative variables. Then, permutation combination population analysis (PCPA) is proposed, which introduces the core idea of permutation analysis into the variable combination population analysis (VCPA) and hence improves its ability in evaluating the importance of informative variables. Six state-of-the-art wavelength selection methods are used to compare with iPCPA and their performances are tested by using three real spectral datasets: corn, beer, and soil datasets. The final experimental results prove that iPCPA has the best predictive abilities, combined with a good selective performance.
更多查看译文
关键词
Wavelength selection,Multivariate calibration,Synergistic influence,Permutation analysis,Variable combination population analysis
AI 理解论文
溯源树
样例
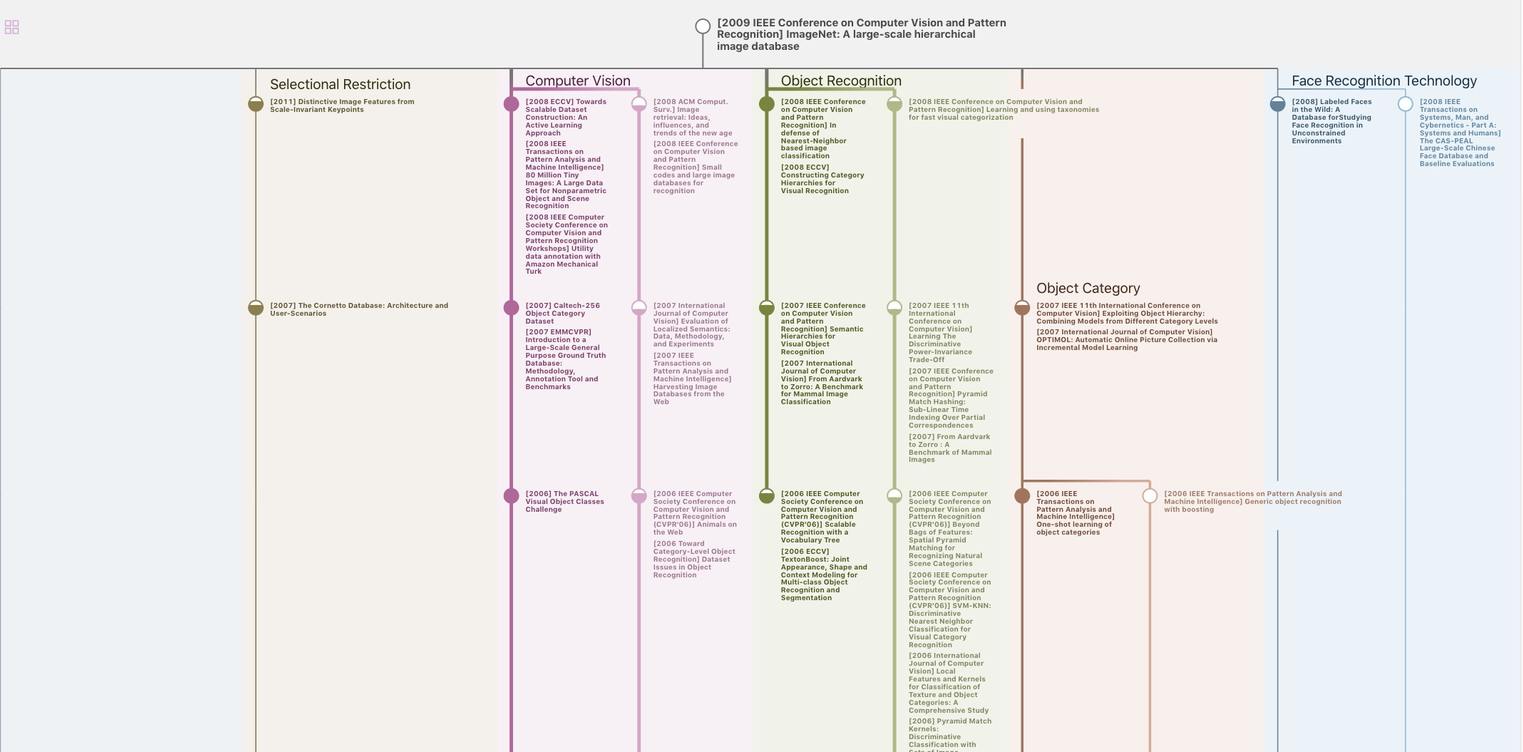
生成溯源树,研究论文发展脉络
Chat Paper
正在生成论文摘要