Multi-VFL: A Vertical Federated Learning System for Multiple Data and Label Owners
ArXiv(2021)
摘要
Vertical Federated Learning (VFL) refers to the collaborative training of a model on a dataset where the features of the dataset are split among multiple data owners, while label information is owned by a single data owner. In this paper, we propose a novel method, Multi Vertical Federated Learning (Multi-VFL), to train VFL models when there are multiple data and label owners. Our approach is the first to consider the setting where $D$-data owners (across which features are distributed) and $K$-label owners (across which labels are distributed) exist. This proposed configuration allows different entities to train and learn optimal models without having to share their data. Our framework makes use of split learning and adaptive federated optimizers to solve this problem. For empirical evaluation, we run experiments on the MNIST and FashionMNIST datasets. Our results show that using adaptive optimizers for model aggregation fastens convergence and improves accuracy.
更多查看译文
关键词
vertical federated learning system,label owners,multiple data,multi-vfl
AI 理解论文
溯源树
样例
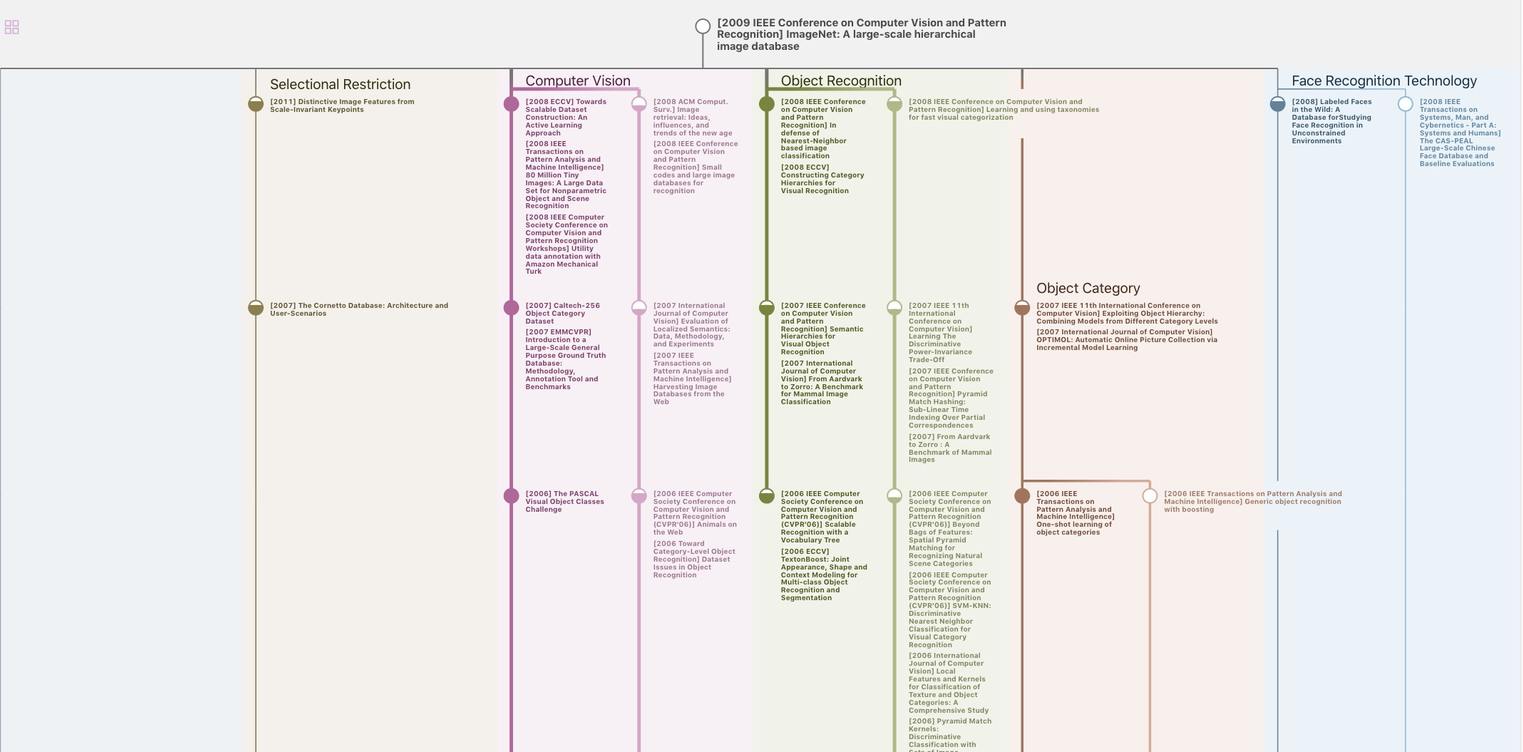
生成溯源树,研究论文发展脉络
Chat Paper
正在生成论文摘要