DNN-based Topology Optimisation: Spatial Invariance and Neural Tangent Kernel.
Annual Conference on Neural Information Processing Systems(2021)
摘要
We study the Solid Isotropic Material Penalization (SIMP) method with a density field generated by a fully-connected neural network, taking the coordinates as inputs. In the large width limit, we show that the use of DNNs leads to a filtering effect similar to traditional filtering techniques for SIMP, with a filter described by the Neural Tangent Kernel (NTK). This filter is however not invariant under translation, leading to visual artifacts and non-optimal shapes. We propose two embeddings of the input coordinates, which lead to (approximate) spatial invariance of the NTK and of the filter. We empirically confirm our theoretical observations and study how the filter size is affected by the architecture of the network. Our solution can easily be applied to any other coordinates-based generation method.
更多查看译文
关键词
topology optimisation,neural tangent kernel,spatial invariance,dnn-based
AI 理解论文
溯源树
样例
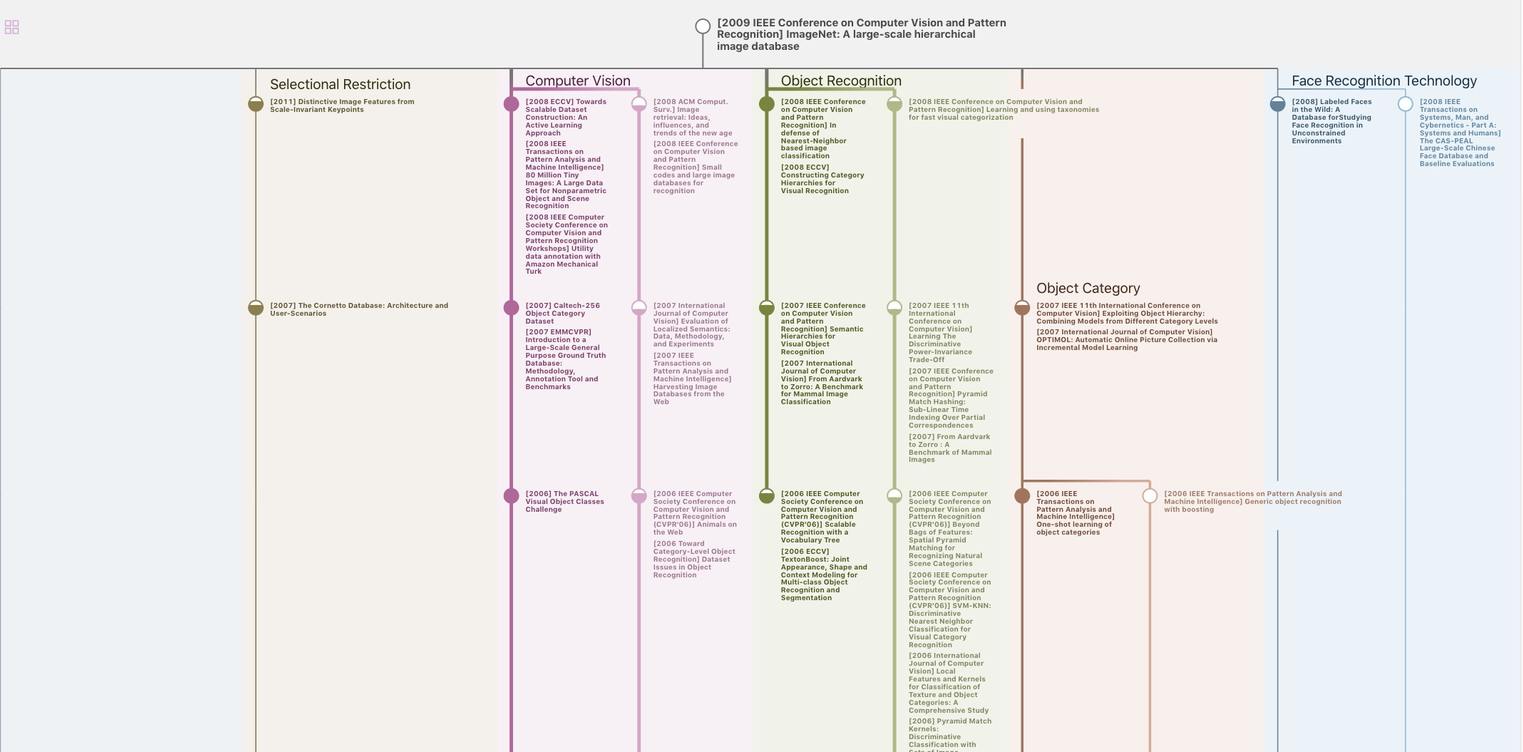
生成溯源树,研究论文发展脉络
Chat Paper
正在生成论文摘要