Anomaly Detection of Bolt Tightening Process Based on Improved SMOTE.
ICRAI(2020)
摘要
For some industrial production processes, deep fault can be detected by data mining and data analytics of the process data. This can help to get a higher level of production quality. Anomaly detection of bolt tightening process was studied in this paper. Imbalanced data set is the main difficulty in this problem. An improved synthetic minority over-sampling technique (SMOTE) algorithm is proposed based on density-based spatial clustering of applications with noise (DBSCAN). By oversampling within-class imbalanced samples, the improved SMOTE algorithm can overcome the shortcomings of the traditional SMOTE method and can retain more sample features. As for the model feature extraction and classification, the sample classifier is trained by the Xgboost algorithm. An Experiment is carried out on a factory's real data set, which shows that the improved SMOTE algorithm can help to achieve great classification performance promotion.
更多查看译文
AI 理解论文
溯源树
样例
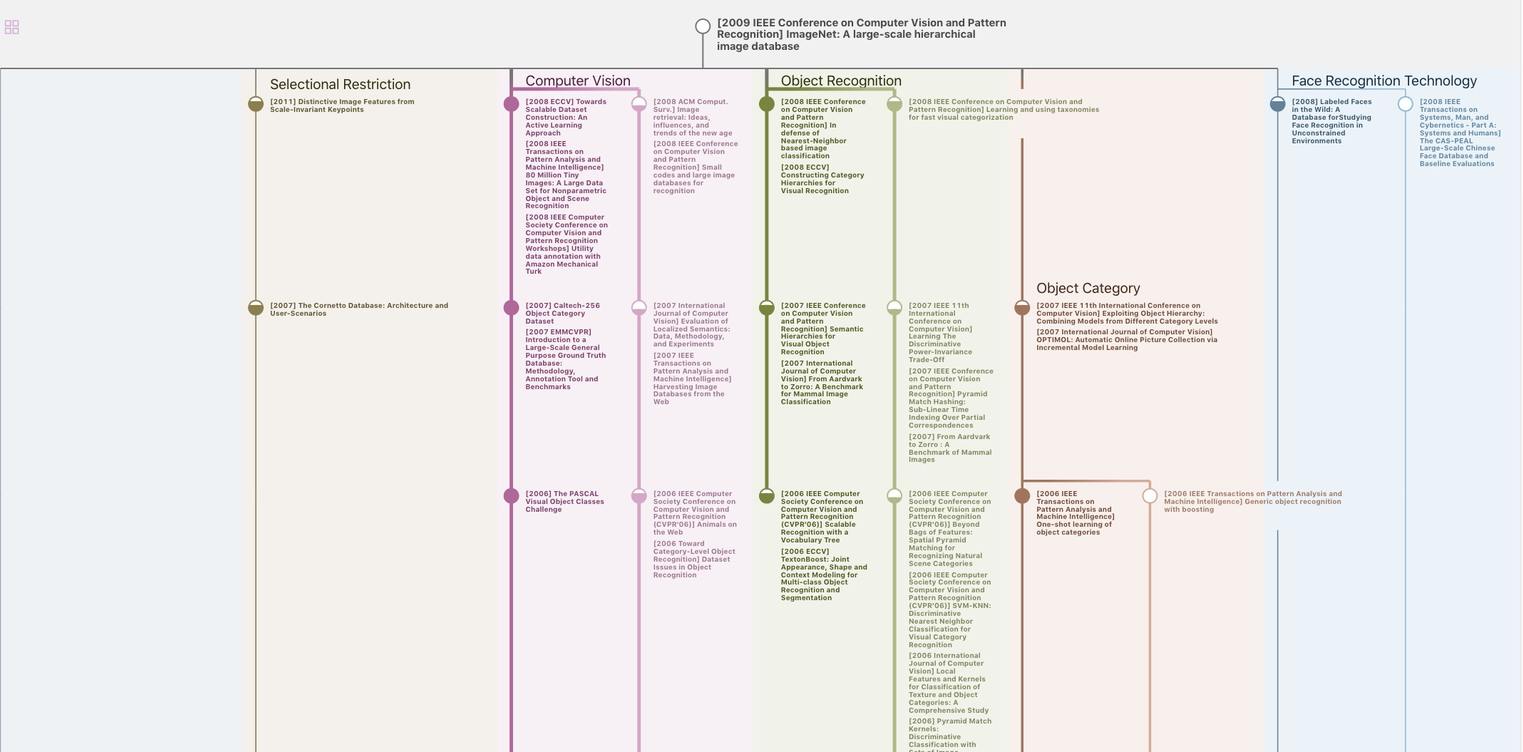
生成溯源树,研究论文发展脉络
Chat Paper
正在生成论文摘要