Cognition-Driven Structural Prior for Instance-Dependent Label Transition Matrix Estimation
IEEE TRANSACTIONS ON NEURAL NETWORKS AND LEARNING SYSTEMS(2024)
摘要
The label transition matrix has emerged as a widely accepted method for mitigating label noise in machine learning. In recent years, numerous studies have centered on leveraging deep neural networks to estimate the label transition matrix for individual instances within the context of instance-dependent noise. However, these methods suffer from low search efficiency due to the large space of feasible solutions. Behind this drawback, we have explored that the real murderer lies in the invalid class transitions, that is, the actual transition probability between certain classes is zero but is estimated to have a certain value. To mask the invalid class transitions, we introduced a human-cognition-assisted method with structural information from human cognition. Specifically, we introduce a structured transition matrix network (STMN ) designed with an adversarial learning process to balance instance features and prior information from human cognition. The proposed method offers two advantages: 1) better estimation effectiveness is obtained by sparing the transition matrix and 2) better estimation accuracy is obtained with the assistance of human cognition. By exploiting these two advantages, our method parametrically estimates a sparse label transition matrix, effectively converting noisy labels into true labels. The efficiency and superiority of our proposed method are substantiated through comprehensive comparisons with state-of-the-art methods on three synthetic datasets and a real-world dataset. Our code will be available at https://github.com/WheatCao/STMN-Pytorch.
更多查看译文
关键词
Human cognition,label correction,label noise,transition matrix
AI 理解论文
溯源树
样例
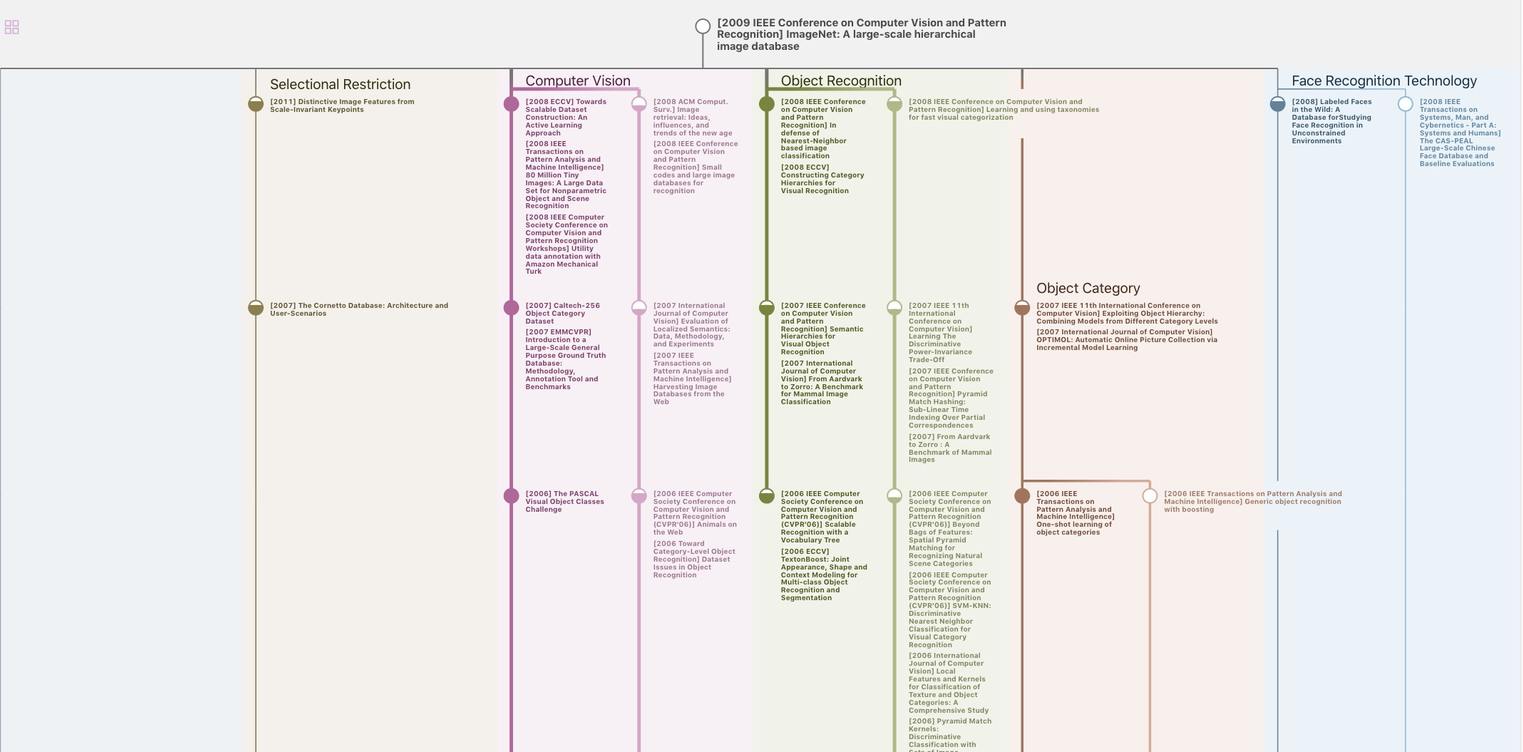
生成溯源树,研究论文发展脉络
Chat Paper
正在生成论文摘要