Optimal Sensor Precision for Multirate Sensing for Bounded Estimation Error
IEEE Transactions on Aerospace and Electronic Systems(2022)
摘要
We address the problem of determining optimal sensor precisions for estimating the states of linear time-varying discrete-time stochastic dynamical systems, with guaranteed bounds on the estimation errors. This is performed in the Kalman filtering framework, where the sensor precisions are treated as variables. They are determined by solving a constrained convex optimization problem, which guarantees the specified upper bound on the posterior error variance. Optimal sensor precisions are determined by minimizing the
$l_1$
norm, which promotes sparseness in the solution and indirectly addresses the sensor selection problem. The theory is applied to realistic flight mechanics and astrodynamics problems to highlight its engineering value. These examples demonstrate the application of the presented theory to 1) determine redundant sensing architectures for linear time invariant systems, 2) accurately estimate states with low-cost sensors, and 3) optimally schedule sensors for linear time-varying systems.
更多查看译文
关键词
Convex optimization,Kalman filtering,sensor precision
AI 理解论文
溯源树
样例
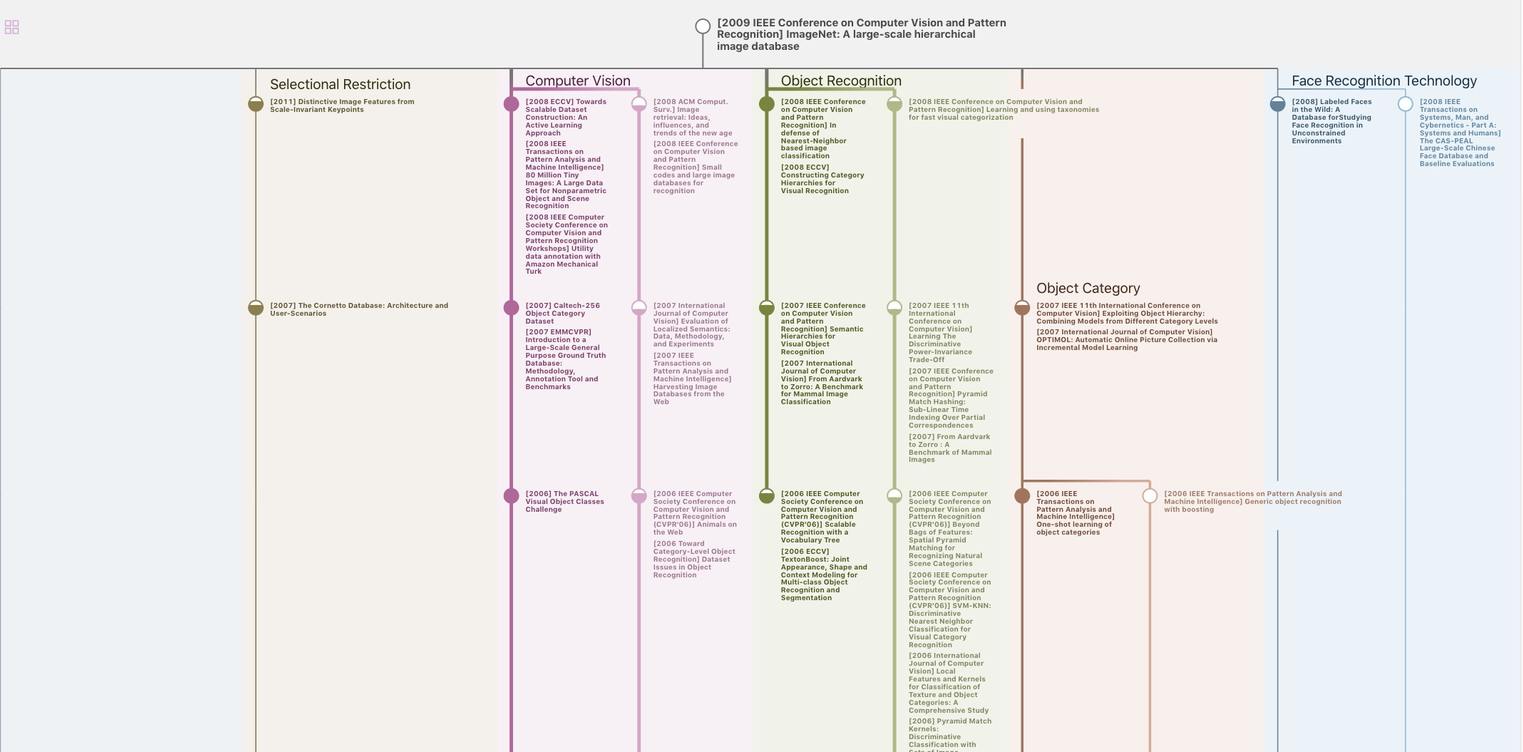
生成溯源树,研究论文发展脉络
Chat Paper
正在生成论文摘要