BoolNet: Minimizing The Energy Consumption of Binary Neural Networks
arxiv(2021)
摘要
Recent works on Binary Neural Networks (BNNs) have made promising progress in narrowing the accuracy gap of BNNs to their 32-bit counterparts. However, the accuracy gains are often based on specialized model designs using additional 32-bit components. Furthermore, almost all previous BNNs use 32-bit for feature maps and the shortcuts enclosing the corresponding binary convolution blocks, which helps to effectively maintain the accuracy, but is not friendly to hardware accelerators with limited memory, energy, and computing resources. Thus, we raise the following question: How can accuracy and energy consumption be balanced in a BNN network design? We extensively study this fundamental problem in this work and propose a novel BNN architecture without most commonly used 32-bit components: \textit{BoolNet}. Experimental results on ImageNet demonstrate that BoolNet can achieve 4.6x energy reduction coupled with 1.2\% higher accuracy than the commonly used BNN architecture Bi-RealNet. Code and trained models are available at: https://github.com/hpi-xnor/BoolNet.
更多查看译文
关键词
binary neural networks,neural networks,energy consumption
AI 理解论文
溯源树
样例
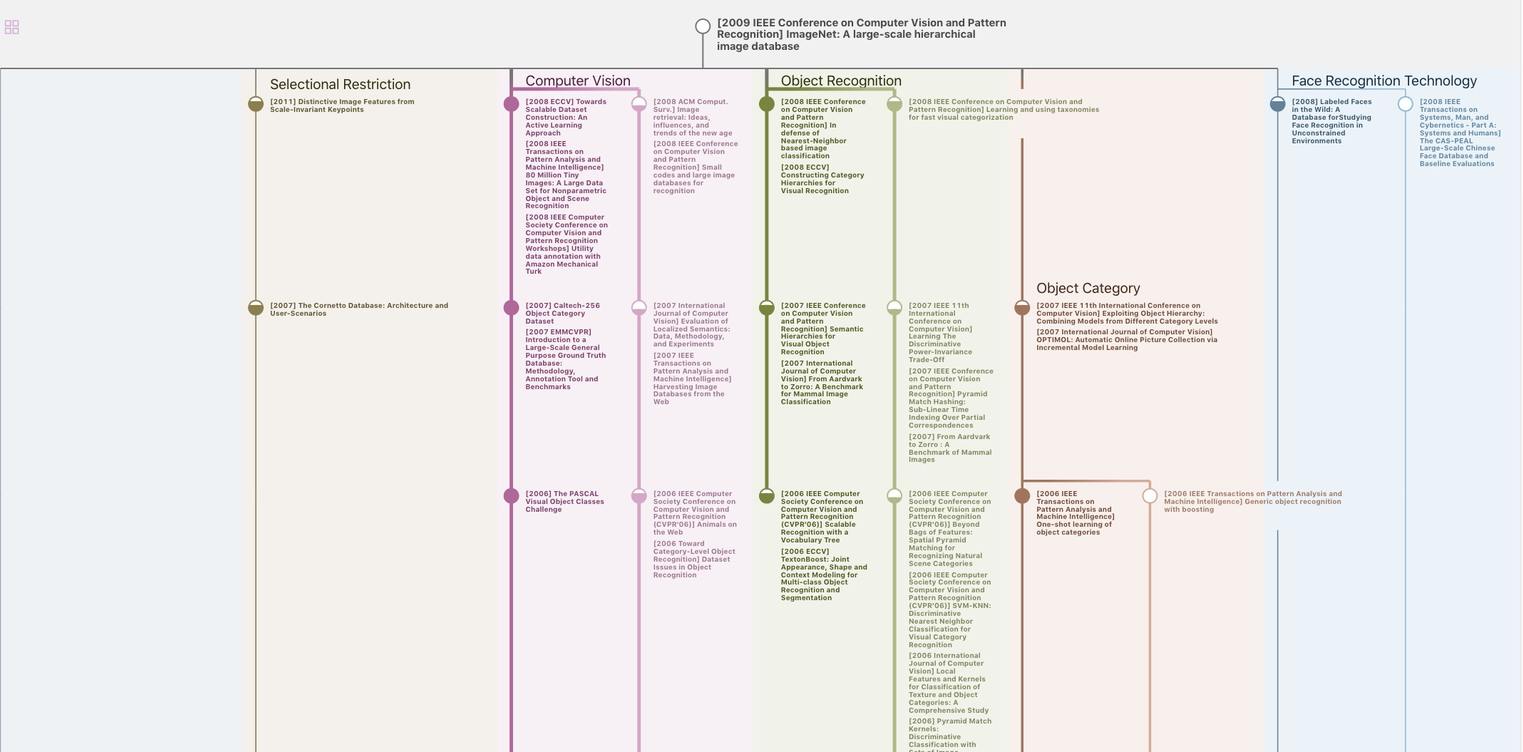
生成溯源树,研究论文发展脉络
Chat Paper
正在生成论文摘要