A scalable multi-step least squares method for network identification with unknown disturbance topology
Automatica(2022)
摘要
Identification methods for dynamic networks typically require prior knowledge of the network and disturbance topology, and often rely on solving poorly scalable non-convex optimization problems. While methods for estimating network topology are available in the literature, less attention has been paid to estimating the disturbance topology, i.e., the (spatial) noise correlation structure and the noise rank in a filtered white noise representation of the disturbance signal. In this work we present an identification method for dynamic networks, in which an estimation of the disturbance topology precedes the identification of the full dynamic network with known network topology. To this end we extend the multi-step Sequential Linear Regression and Weighted Null Space Fitting methods to deal with reduced rank noise, and use these methods to estimate the disturbance topology and the network dynamics in the full measurement situation. As a result, we provide a multi-step least squares algorithm with parallel computation capabilities and that rely only on explicit analytical solutions, thereby avoiding the usual non-convex optimizations involved. Consequently we consistently estimate dynamic networks of Box Jenkins model structure, while keeping the computational burden low. We provide a consistency proof that includes path-based data informativity conditions for allocation of excitation signals in the experimental design. Numerical simulations performed on a dynamic network with reduced rank noise clearly illustrate the potential of this method.
更多查看译文
关键词
System identification,Dynamic networks,Estimation algorithms,Least squares,Topology estimation
AI 理解论文
溯源树
样例
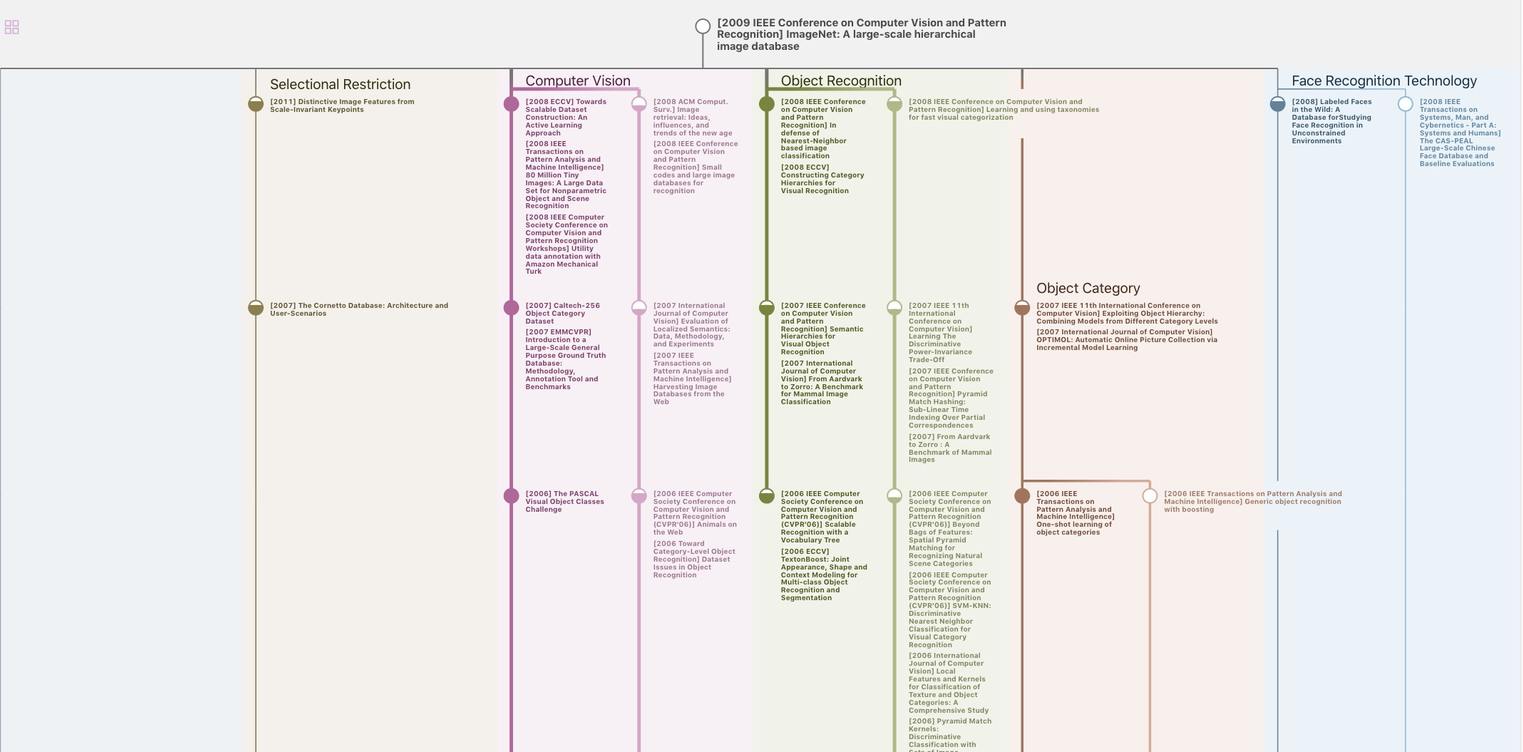
生成溯源树,研究论文发展脉络
Chat Paper
正在生成论文摘要