Learning Multi-Scale Photo Exposure Correction
2021 IEEE/CVF CONFERENCE ON COMPUTER VISION AND PATTERN RECOGNITION, CVPR 2021(2021)
摘要
Capturing photographs with wrong exposures remains a major source of errors in camera-based imaging. Exposure problems are categorized as either: (i) overexposed, where the camera exposure was too long, resulting in bright and washed-out image regions, or (ii) underexposed, where the exposure was too short, resulting in dark regions. Both under- and overexposure greatly reduce the contrast and visual appeal of an image. Prior work mainly focuses on underexposed images or general image enhancement. In contrast, our proposed method targets both over- and underexposure errors in photographs. We formulate the exposure correction problem as two main sub-problems: (i) color enhancement and (ii) detail enhancement. Accordingly, we propose a coarse-to-fine deep neural network (DNN) model, trainable in an end-to-end manner, that addresses each sub-problem separately. A key aspect of our solution is a new dataset of over 24,000 images exhibiting the broadest range of exposure values to date with a corresponding properly exposed image. Our method achieves results on par with existing state-of-the-art methods on underexposed images and yields significant improvements for images suffering from overexposure errors.
更多查看译文
关键词
camera-based imaging,camera exposure,image regions,dark regions,visual appeal,underexposed images,general image enhancement,underexposure errors,exposure correction problem,color enhancement,detail enhancement,coarse-to-fine deep neural network model,image exposure,overexposure errors,multiscale photo exposure correction learning,photograph underexposure errors,DNN
AI 理解论文
溯源树
样例
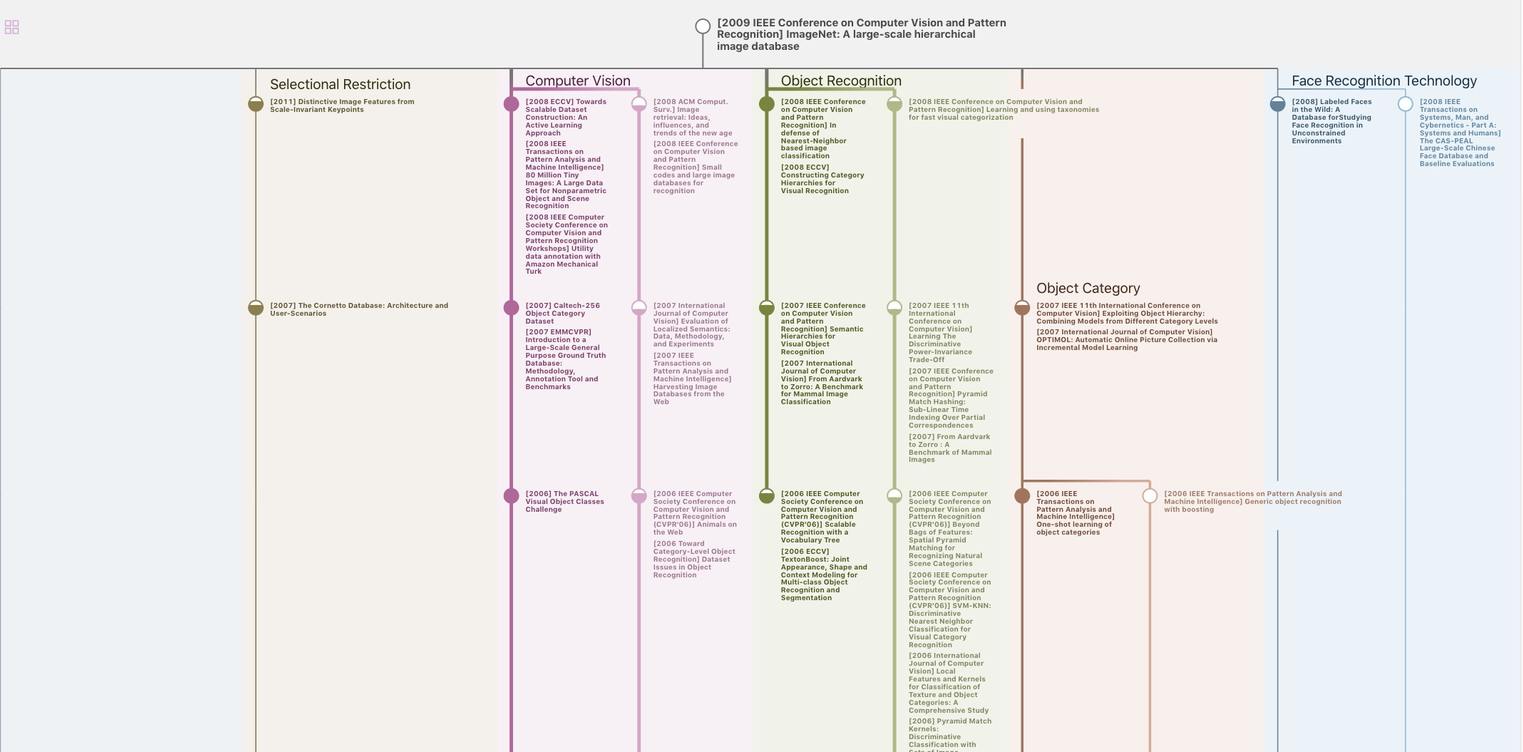
生成溯源树,研究论文发展脉络
Chat Paper
正在生成论文摘要