Convex-Hull Feature Adaptation for Oriented and Densely Packed Object Detection
IEEE transactions on circuits and systems for video technology(2022)
摘要
Detecting oriented and densely packed objects is a challenging problem considering that the receptive field intersection between objects causes spatial feature aliasing. In this paper, we propose a convex-hull feature adaptation (CFA) approach, with the aim to configure convolutional features in accordance with irregular object layouts. CFA roots in the convex-hull feature representation, which defines a set of dynamically sampled feature points guided by the convex intersection over union (CIoU) to bound object extent. CFA pursues optimal feature assignment by constructing convex-hull sets and iteratively splitting positive or negative convex-hulls. By simultaneously considering overlapping convex-hulls and objects and penalizing convex-hulls shared by multiple objects, CFA defines a systematic way to adapt convolutional features on regular grids to objects of irregular shapes. Experiments on DOTA and SKU110K-R datasets show that CFA achieved new state-of-the-art performance for detecting oriented and densely packed objects. CFA also sets a solid baseline for convex polygon prediction on the MS COCO dataset defined for general object detection. Code is available at https://github.com/SDL-GuoZonghao/BeyondBoundingBox .
更多查看译文
关键词
Feature extraction,Layout,Detectors,Training,Object detection,Location awareness,Transformers,Oriented object detection,dense object detection,feature adaptation,convex-hull,polygon detection
AI 理解论文
溯源树
样例
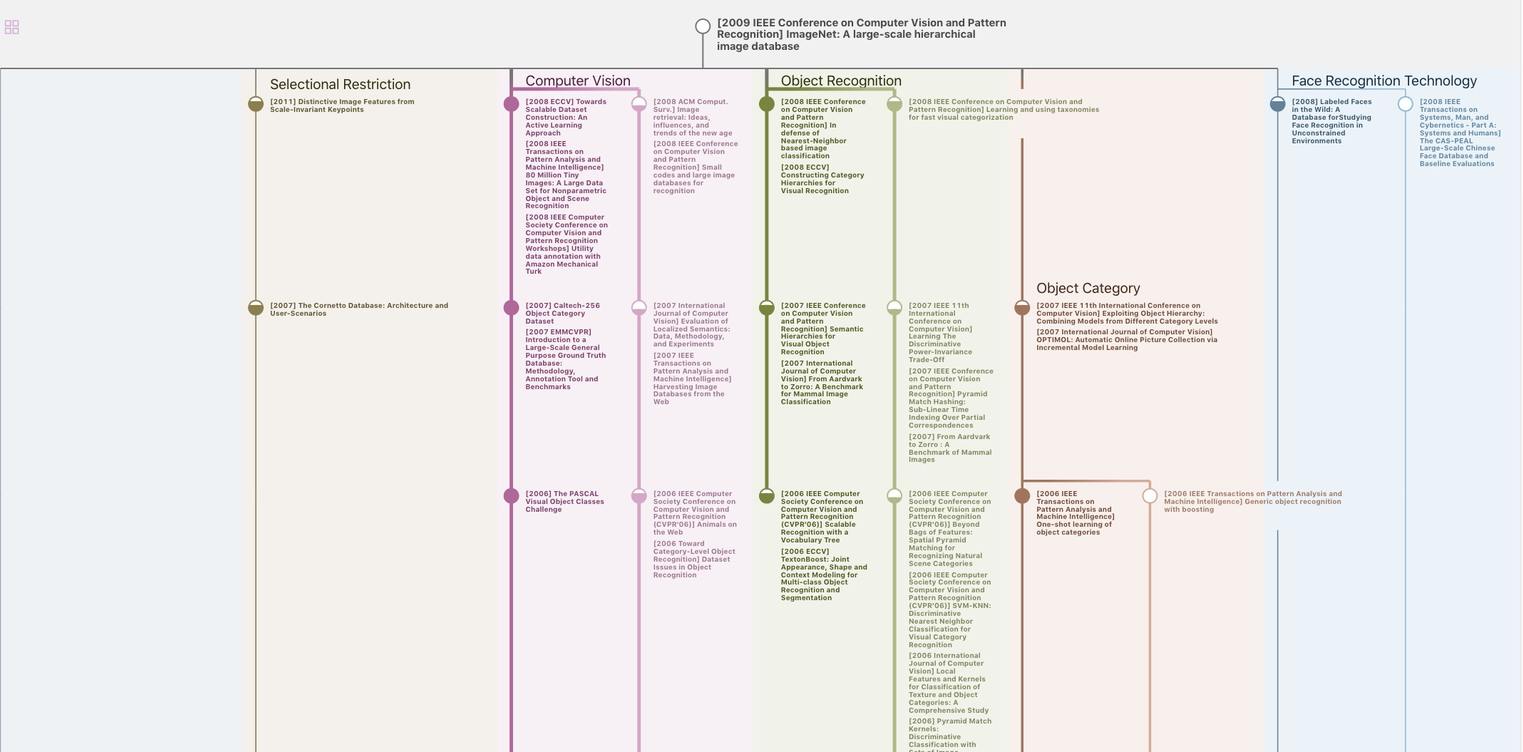
生成溯源树,研究论文发展脉络
Chat Paper
正在生成论文摘要