Dual-GAN: Joint BVP and Noise Modeling for Remote Physiological Measurement
2021 IEEE/CVF CONFERENCE ON COMPUTER VISION AND PATTERN RECOGNITION, CVPR 2021(2021)
摘要
Remote photoplethysmography (rPPG) based physiological measurement has great application values in health monitoring, emotion analysis, etc. Existing methods mainly focus on how to enhance or extract the very weak blood volume pulse (BVP) signals from face videos, but seldom explicitly model the noises that dominate face video content. Thus, they may suffer from poor generalization ability in unseen scenarios. This paper proposes a novel adversarial learning approach for rPPG based physiological measurement by using Dual Generative Adversarial Networks (Dual-GAN) to model the BVP predictor and noise distribution jointly. The BVP-GAN aims to learn a noiseresistant mapping from input to ground-truth BVP, and the Noise-GAN aims to learn the noise distribution. The two GANs can promote each other's capability, leading to improved feature disentanglement between BVP and noises. Besides, a plug-and-play block named ROI alignment and fusion (ROI-AF) block is proposed to alleviate the inconsistencies between different ROIs and exploit informative features from a wider receptive field in terms of ROIs. In comparison to state-of-the-art methods, our approach achieves better performance in heart rate, heart rate variability, and respiration frequency estimation from face videos.
更多查看译文
关键词
dual generative adversarial networks,BVP-GAN,noise-resistant mapping,Noise-GAN,noise modeling,remote physiological measurement,remote photoplethysmography,health monitoring,blood volume pulse signals,rPPG based physiological measurement,dual-GAN,plug-and-play block,ROI alignment and fusion block,ROI-AF block,adversarial learning
AI 理解论文
溯源树
样例
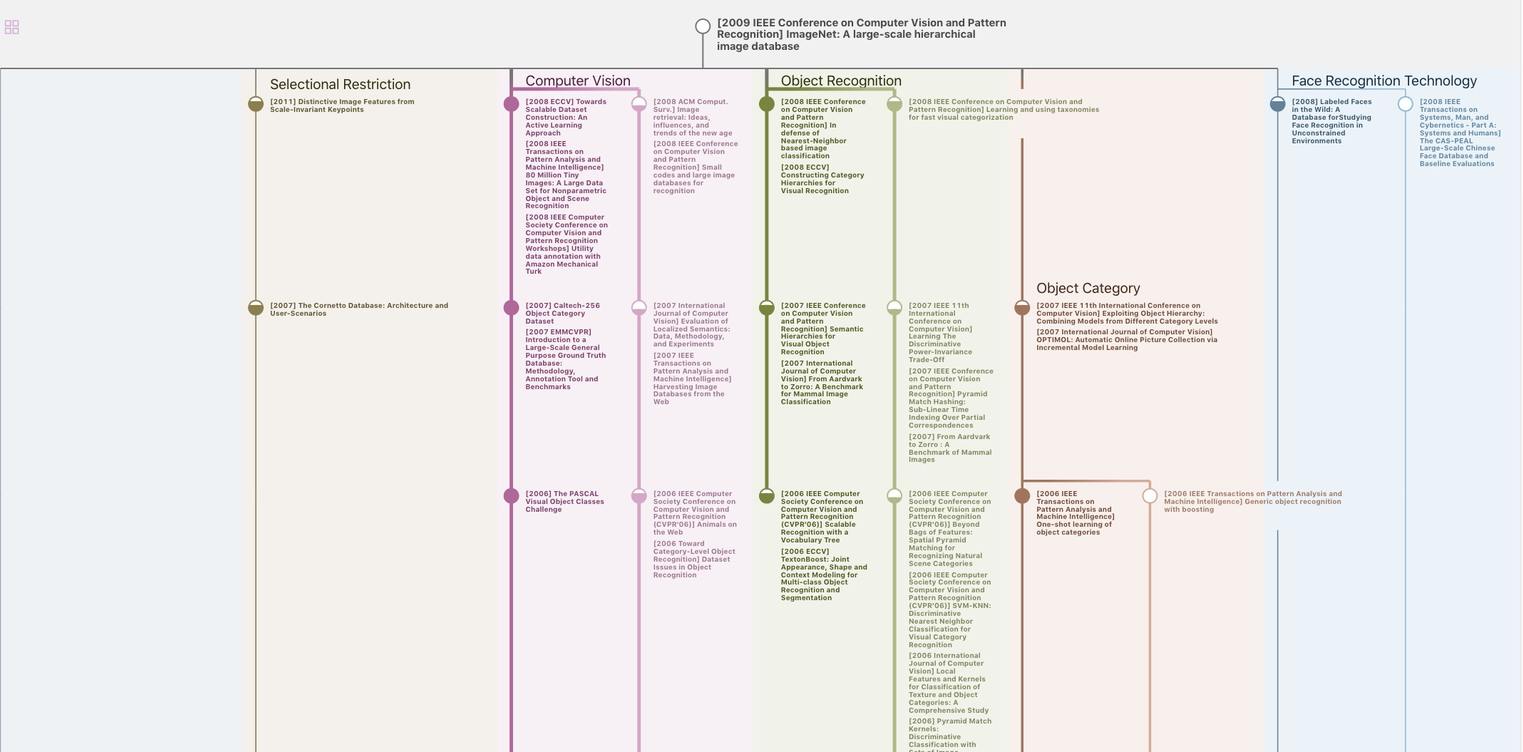
生成溯源树,研究论文发展脉络
Chat Paper
正在生成论文摘要