LAU-Net: Latitude Adaptive Upscaling Network for Omnidirectional Image Super-resolution
2021 IEEE/CVF CONFERENCE ON COMPUTER VISION AND PATTERN RECOGNITION, CVPR 2021(2021)
摘要
The omnidirectional images (ODIs) are usually at low-resolution, due to the constraints of collection, storage and transmission. The traditional two-dimensional (2D) image super-resolution methods are not effective for spherical ODIs, because ODIs tend to have non-uniformly distributed pixel density and varying texture complexity across latitudes. In this work, we propose a novel latitude adaptive upscaling network (LAU-Net) for ODI super-resolution, which allows pixels at different latitudes to adopt distinct upscaling factors. Specifically, we introduce a Laplacian multi-level separation architecture to split an ODI into different latitude bands, and hierarchically upscale them with different factors. In addition, we propose a deep reinforcement learning scheme with a latitude adaptive reward, in order to automatically select optimal upscaling factors for different latitude bands. To the best of our knowledge, LAU-Net is the first attempt to consider the latitude difference for ODI super-resolution. Extensive results demonstrate that our LAU-Net significantly advances the super-resolution performance for ODIs. Codes are available at https://github.com/wangh-allen/LAU-Net.
更多查看译文
关键词
ODI super-resolution,Laplacian multilevel separation architecture,latitude bands,latitude adaptive reward,optimal upscaling factors,latitude difference,omnidirectional image super-resolution,two-dimensional image super-resolution methods,spherical ODIs,pixel density,texture complexity,novel latitude adaptive upscaling network,LAU-Net,upscaling factors
AI 理解论文
溯源树
样例
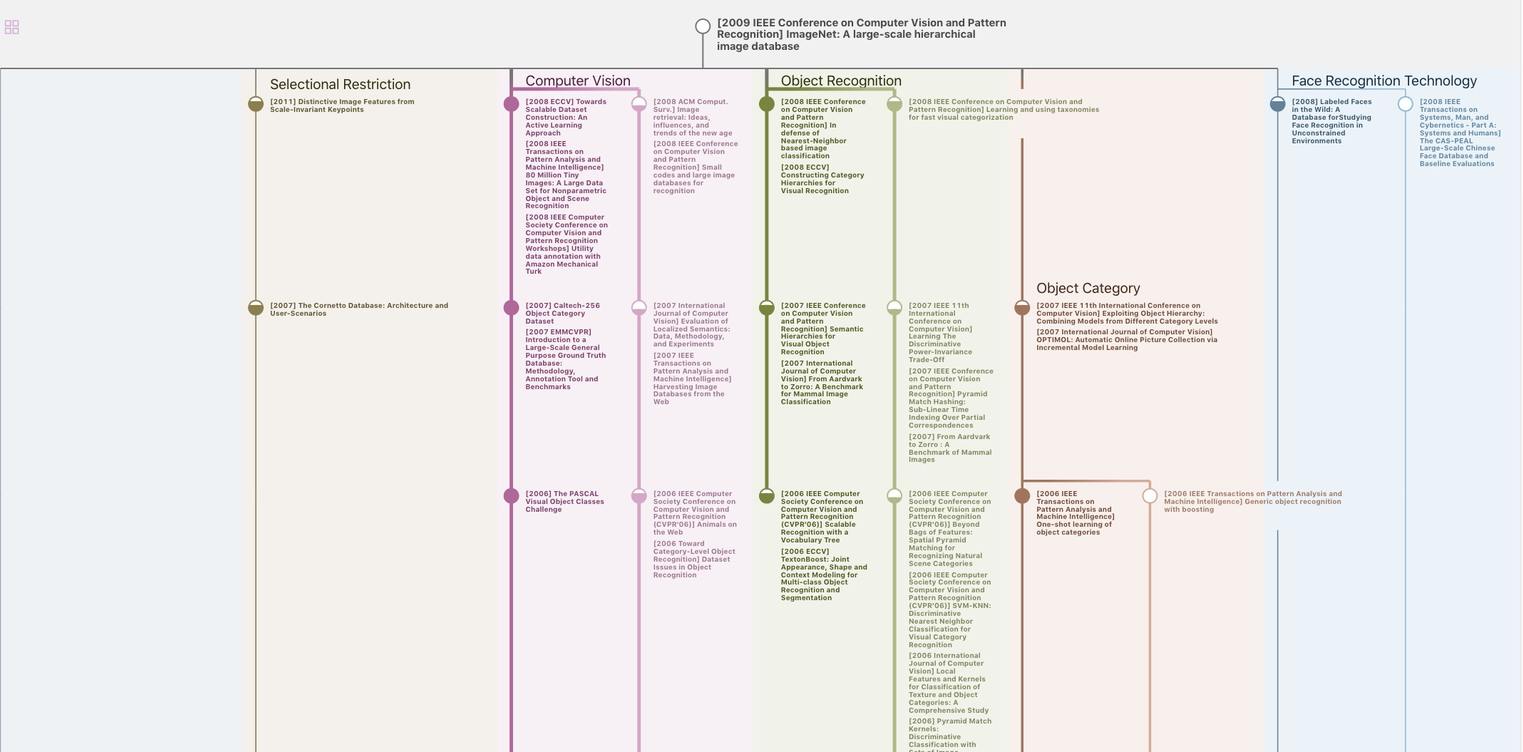
生成溯源树,研究论文发展脉络
Chat Paper
正在生成论文摘要