Hierarchical Video Prediction using Relational Layouts for Human-Object Interactions
2021 IEEE/CVF CONFERENCE ON COMPUTER VISION AND PATTERN RECOGNITION, CVPR 2021(2021)
摘要
Learning to model and predict how humans interact with objects while performing an action is challenging, and most of the existing video prediction models are ineffective in modeling complicated human-object interactions. Our work builds on hierarchical video prediction models, which disentangle the video generation process into two stages: predicting a high-level representation, such as pose sequence, and then learning a pose-to-pixels translation model for pixel generation. An action sequence for a human-object interaction task is typically very complicated, involving the evolution of pose, person's appearance, object locations, and object appearances over time. To this end, we propose a Hierarchical Video Prediction model using Relational Layouts. In the first stage, we learn to predict a sequence of layouts. A layout is a high-level representation of the video containing both pose and objects' information for every frame. The layout sequence is learned by modeling the relationships between the pose and objects using relational reasoning and recurrent neural networks. The layout sequence acts as a strong structure prior to the second stage that learns to map the layouts into pixel space. Experimental evaluation of our method on two datasets, UMD-HOI and Bimanual, shows significant improvements in standard video evaluation metrics such as LPIPS, PSNR, and SSIM. We also perform a detailed qualitative analysis of our model to demonstrate various generalizations.
更多查看译文
关键词
SSIM,PSNR,LPIPS,UMD-HOI,recurrent neural networks,relational reasoning,hierarchical video prediction model,relational layouts,human-object interaction task,action sequence,pose-to-pixels translation model,video generation process,hierarchical video prediction models,existing video prediction models,human-object interactions,standard video evaluation metrics,layout sequence,high-level representation,object appearances
AI 理解论文
溯源树
样例
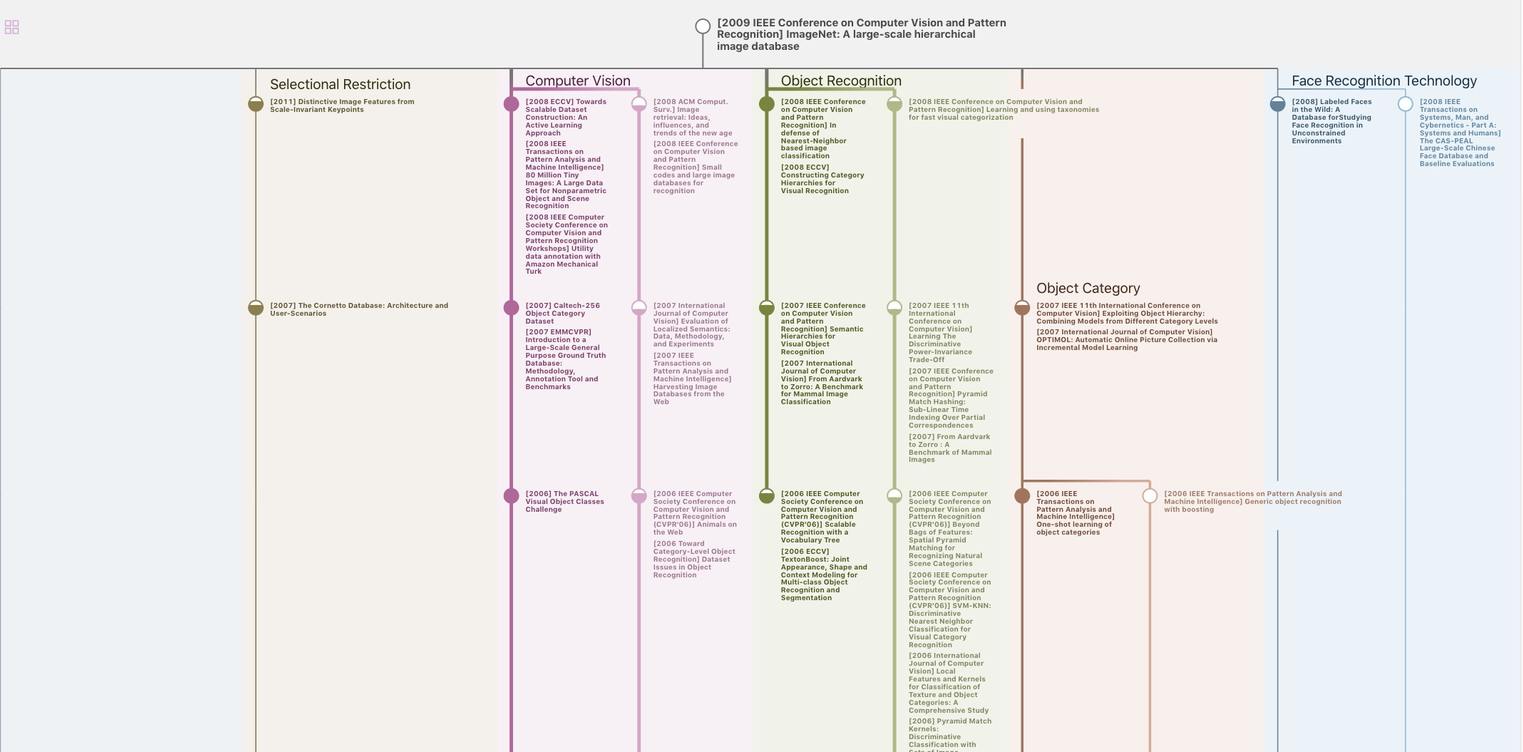
生成溯源树,研究论文发展脉络
Chat Paper
正在生成论文摘要