Extreme Low-Light Environment-Driven Image Denoising over Permanently Shadowed Lunar Regions with a Physical Noise Model
2021 IEEE/CVF CONFERENCE ON COMPUTER VISION AND PATTERN RECOGNITION, CVPR 2021(2021)
摘要
Recently, learning-based approaches have achieved impressive results in the field of low-light image denoising. Some state of the art approaches employ a rich physical model to generate realistic training data. However, the performance of these approaches ultimately depends on the realism of the physical model, and many works only concentrate on everyday photography. In this work we present a denoising approach for extremely low-light images of permanently shadowed regions (PSRs) on the lunar surface, taken by the Narrow Angle Camera on board the Lunar Reconnaissance Orbiter satellite. Our approach extends existing learning-based approaches by combining a physical noise model of the camera with real noise samples and training image scene selection based on 3D ray tracing to generate realistic training data. We also condition our denoising model on the camera's environmental metadata at the time of image capture (such as the camera's temperature and age), showing that this improves performance. Our quantitative and qualitative results show that our method strongly outperforms the existing calibration routine for the camera and other baselines. Our results could significantly impact lunar science and exploration, for example by aiding the identification of surface water-ice and reducing uncertainty in rover and human traverse planning into PSRs.
更多查看译文
关键词
extreme low-light environment,permanently shadowed Lunar regions,physical noise model,learning-based approaches,low-light image denoising,rich physical model,realistic training data,denoising approach,low-light images,permanently shadowed regions,lunar surface,Narrow Angle Camera,Lunar Reconnaissance Orbiter satellite,noise samples,training image scene selection,denoising model,image capture,lunar science
AI 理解论文
溯源树
样例
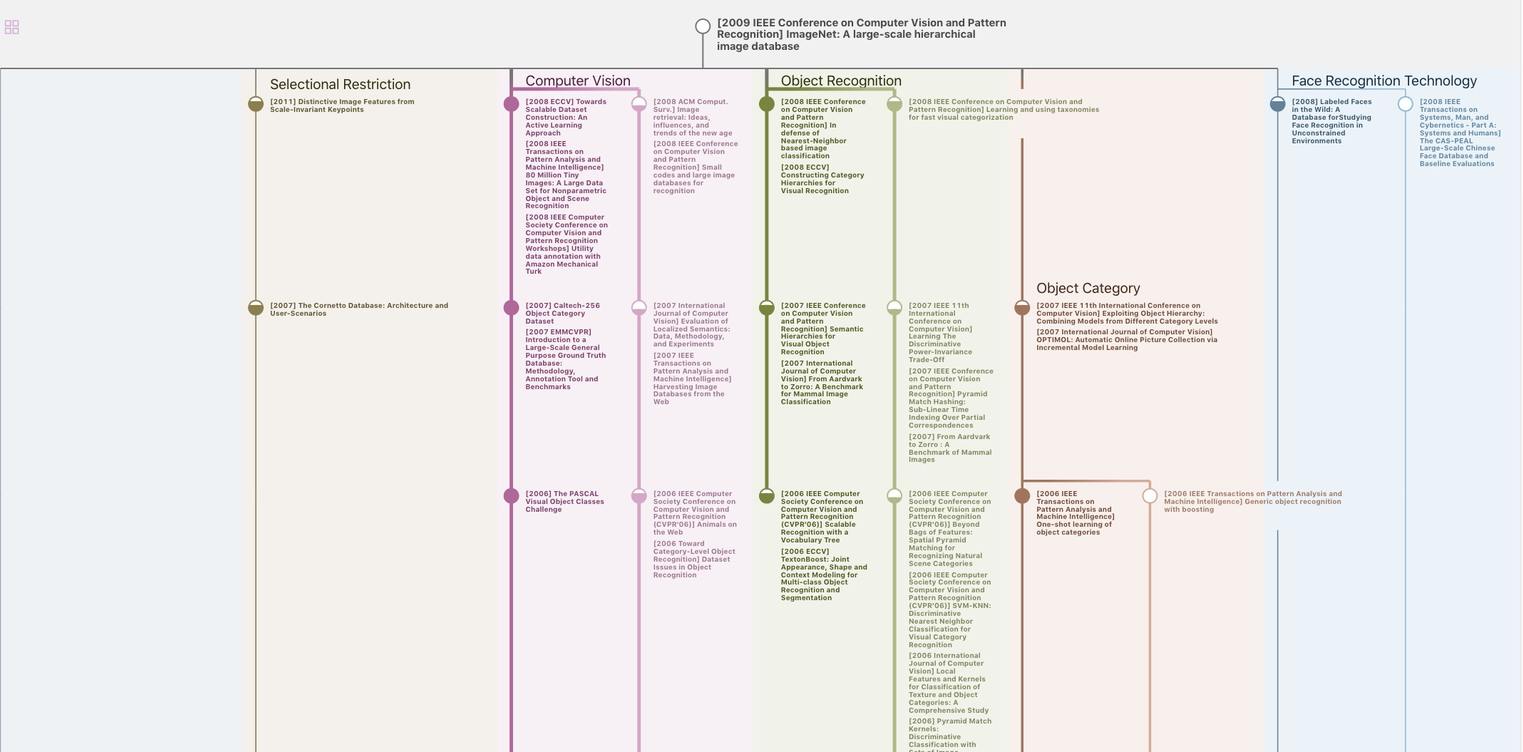
生成溯源树,研究论文发展脉络
Chat Paper
正在生成论文摘要