Uncertainty Guided Collaborative Training for Weakly Supervised Temporal Action Detection
IEEE transactions on pattern analysis and machine intelligence(2022)
摘要
In weakly supervised (WSAL) and unsupervised temporal action localization (UAL), the target is to simultaneously localize temporal boundaries and identify category labels of actions with only video-level category labels (WSAL) or category numbers in a dataset (UAL) during training. Among existing methods, attention based methods have achieved superior performance in both tasks by highlighting action segments with foreground attention weights. However, without the segment-level supervision on the attention weight learning, the quality of the attention weight hinders the performance of these methods. In this paper, we propose a novel Uncertainty Guided Collaborative Training (UGCT) strategy to alleviate this problem, which mainly includes two key designs: (1) The first design is an online pseudo label generation module, in which the RGB and FLOW streams work collaboratively to learn from each other. (2) The second design is an uncertainty aware learning module, which can mitigate the noise in the generated pseudo labels. These two designs work together to promote the model performance effectively and efficiently by exchanging information between RGB and FLOW streams. Extensive experimental results on two benchmark datasets with three attention based methods demonstrate the effectiveness of the proposed method, e.g, more than 7.0% performance gain for mAP@IoU=0.5 on THUMOS14 dataset.
更多查看译文
关键词
Training,Uncertainty,Location awareness,Testing,Task analysis,Proposals,Noise measurement,Weakly supervised,unsupervised,action localization,collaborative training
AI 理解论文
溯源树
样例
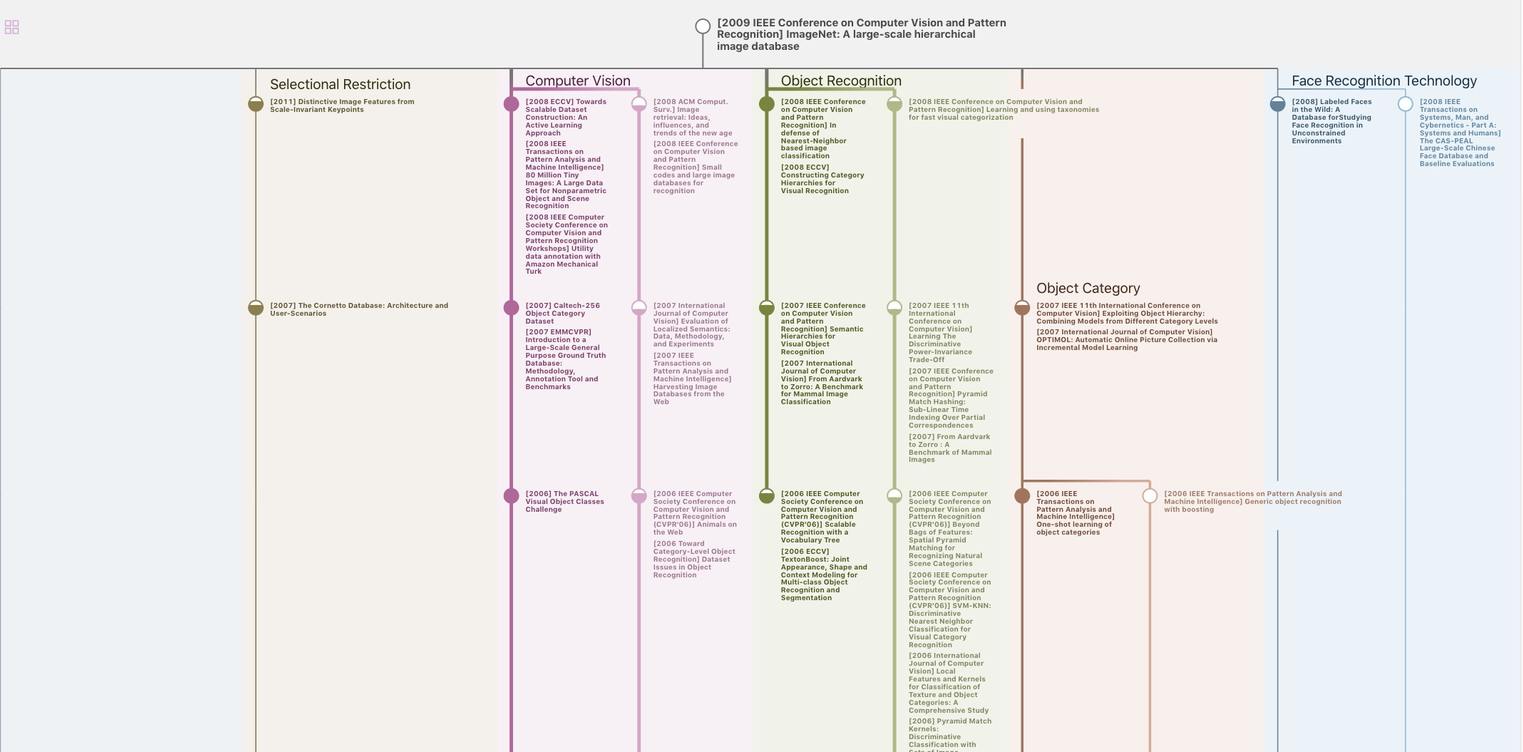
生成溯源树,研究论文发展脉络
Chat Paper
正在生成论文摘要