Dynamic Probabilistic Graph Convolution for Facial Action Unit Intensity Estimation
2021 IEEE/CVF CONFERENCE ON COMPUTER VISION AND PATTERN RECOGNITION, CVPR 2021(2021)
摘要
Deep learning methods have been widely applied to automatic facial action unit (AU) intensity estimation and achieved the state-of-the-art performance. These methods, however, are mostly appearance-based and fail to exploit the underlying structural information among AUs. In this paper, we propose a novel dynamic probabilistic graph convolution (DPG) model to simultaneously exploit AU appearances, AU dynamics, and their semantic structural dependencies for AU intensity estimation. Firstly, we propose to use Bayesian Network to capture the inherent dependencies among AUs. Secondly, we introduce probabilistic graph convolution that allows to perform graph convolution on the distribution of Bayesian Network structure to extract AU structural features. Finally, we introduce a dynamic deep model based on LSTM to simultaneously combine AU appearance features, AU dynamic features, and AU structural features for AU intensity estimation. In experiments, our method achieves comparable and even better performance with the state-of-the-art methods on two benchmark facial AU intensity estimation databases, i.e., FERA 2015 and DISFA.
更多查看译文
关键词
Bayesian network,dynamic deep model,AU appearance features,automatic facial action unit intensity estimation,dynamic probabilistic graph convolution model,deep learning,AU structural feature extraction,LSTM,AU dynamic features
AI 理解论文
溯源树
样例
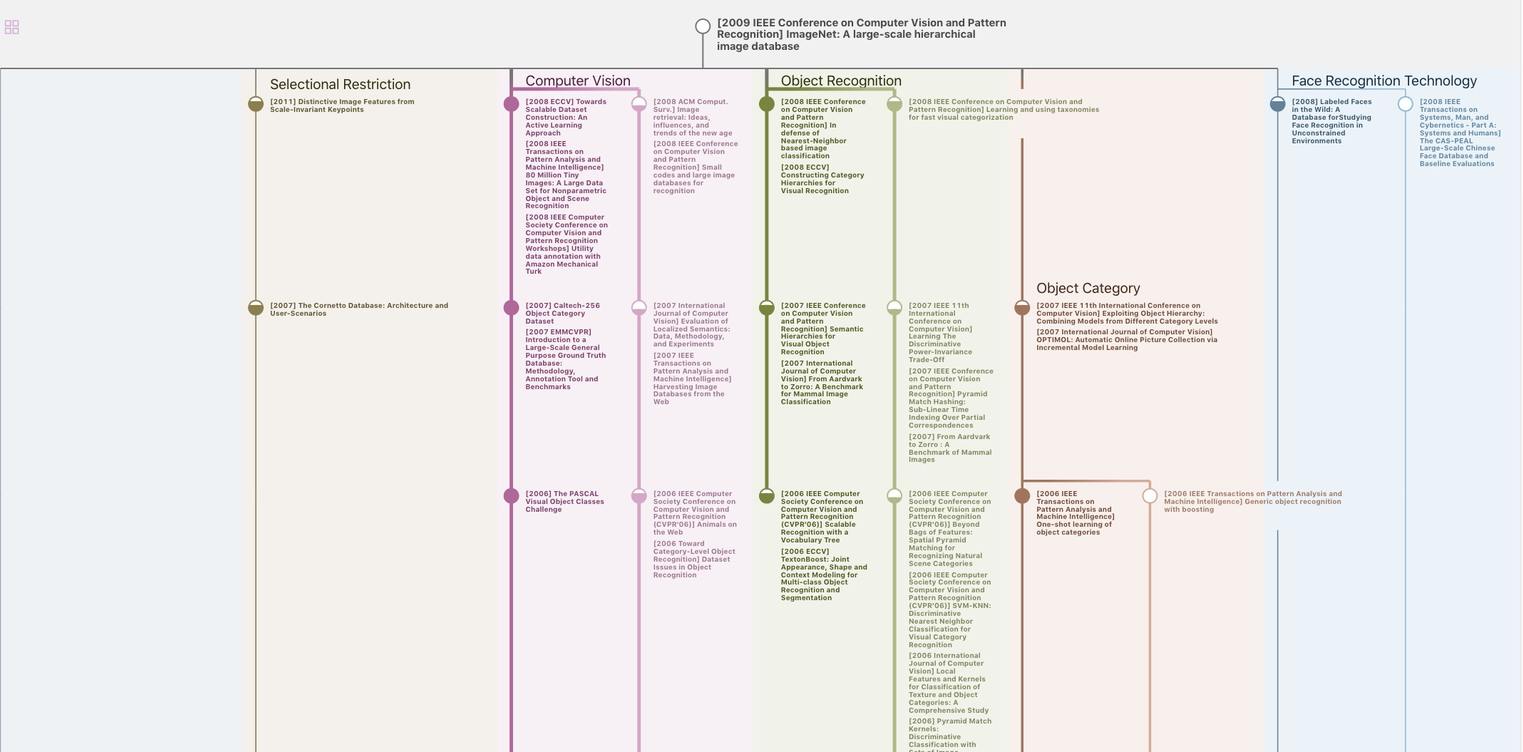
生成溯源树,研究论文发展脉络
Chat Paper
正在生成论文摘要