Limitations Of Recursive Logit For Inverse Reinforcement Learning Of Bicycle Route Choice Behavior In Amsterdam
12TH INTERNATIONAL CONFERENCE ON AMBIENT SYSTEMS, NETWORKS AND TECHNOLOGIES (ANT) / THE 4TH INTERNATIONAL CONFERENCE ON EMERGING DATA AND INDUSTRY 4.0 (EDI40) / AFFILIATED WORKSHOPS(2021)
摘要
Used for route choice modelling by the transportation research community, recursive logit is a form of inverse reinforcement learning. By solving a large-scale system of linear equations recursive logit allows estimation of an optimal (negative) reward function in a computationally efficient way that performs for large networks and a large number of observations. In this paper we review examples of recursive logit and inverse reinforcement learning models applied to real world GPS travel trajectories and explore some of the challenges in modeling bicycle route choice in the city of Amsterdam using recursive logit as compared to a simple baseline multinomial logit model with environmental variables.We discuss conceptual, computational, numerical and statistical issues that we encountered and conclude with recommendation for further research. (C) 2021 The Authors. Published by Elsevier B.V.
更多查看译文
关键词
recursive logit, Markov decision process, inverse reinforcement learning, GPS trajectory, bicycle route choice
AI 理解论文
溯源树
样例
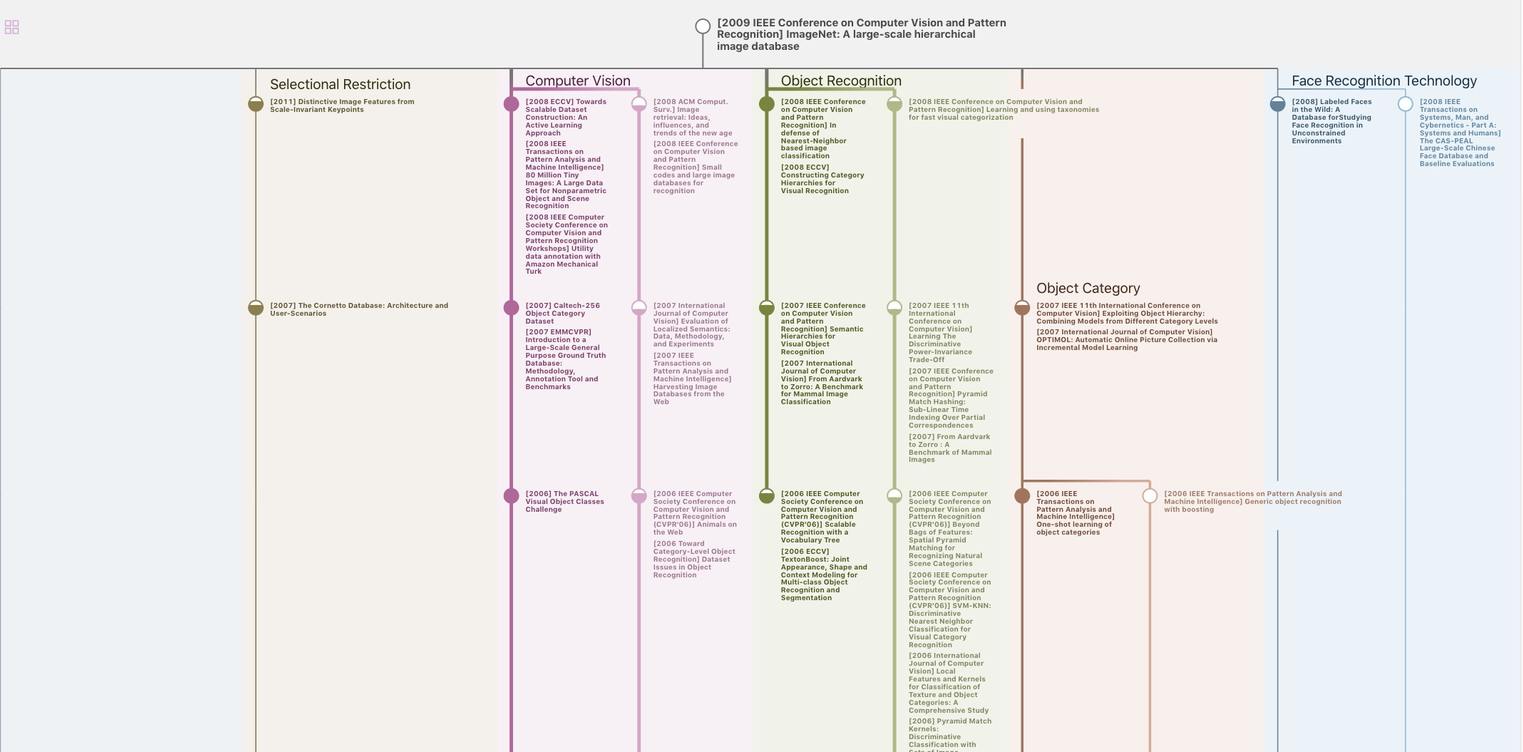
生成溯源树,研究论文发展脉络
Chat Paper
正在生成论文摘要